A Dataless FaceSwap Detection Approach Using Synthetic Images
2022 IEEE International Joint Conference on Biometrics (IJCB)(2022)
摘要
Face swapping technology used to create “Deepfakes” has advanced significantly over the past few years and now enables us to create realistic facial manipulations. Current deep learning algorithms to detect deepfakes have shown promising results, however, they require large amounts of training data, and as we show they are biased towards a particular ethnicity. We propose a deepfake detection methodology that eliminates the need for any real data by making use of synthetically generated data using Style-GAN3. This not only performs at par with the traditional training methodology of using real data but it shows better generalization capabilities when finetuned with a small amount of real data. Furthermore, this also reduces biases created by facial image datasets that might have sparse data from particular ethnicities. To promote reproducibility the code base has been made publicly available
1
1
https://github.com/anubhav1997/youneednodataset
更多查看译文
AI 理解论文
溯源树
样例
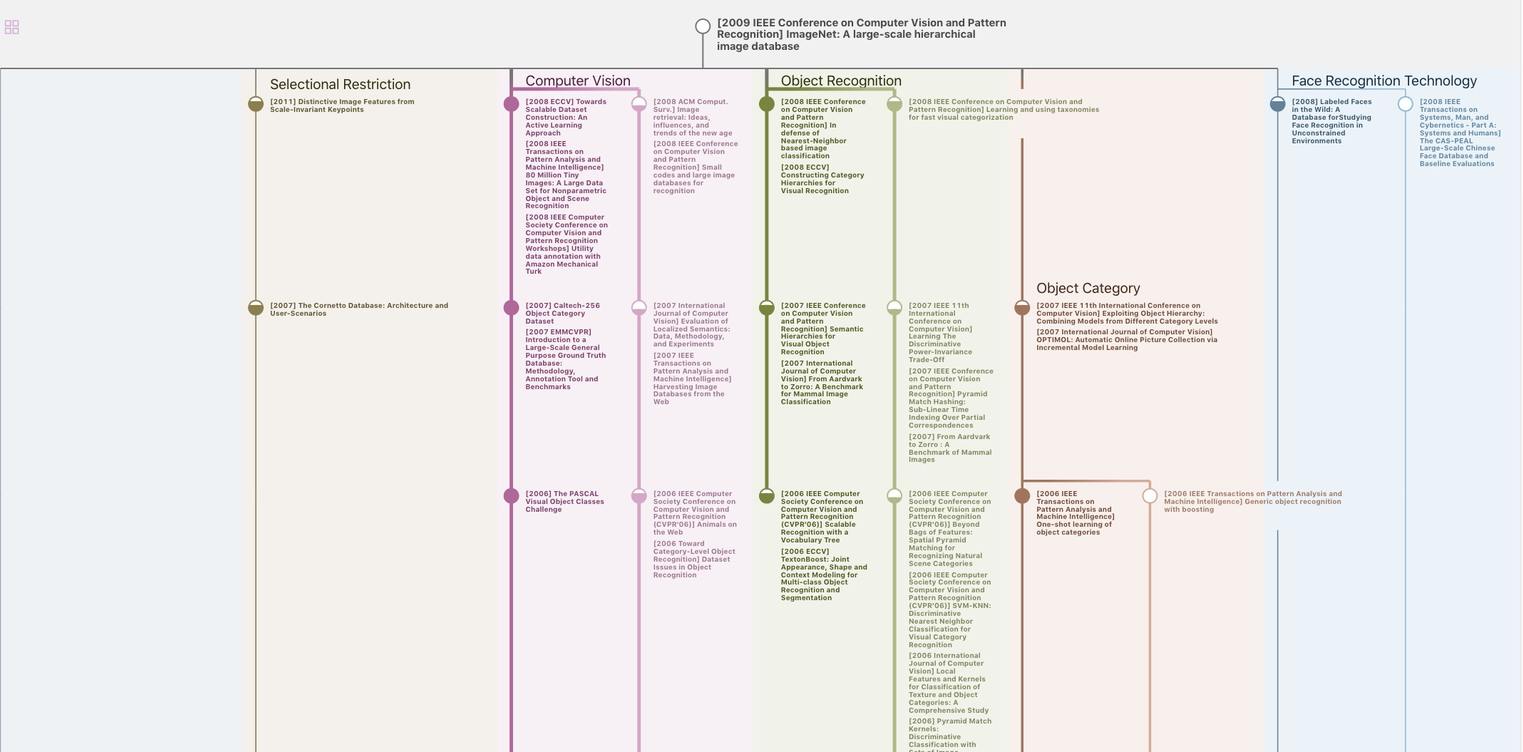
生成溯源树,研究论文发展脉络
Chat Paper
正在生成论文摘要