Predicting ozone formation in petrochemical industrialized Lanzhou city by interpretable ensemble machine learning
Environmental Pollution(2023)
摘要
Ground-level ozone (O3) formation depends on meteorology, precursor emissions, and atmospheric chemistry. Understanding the key drivers behind the O3 formation and developing an accurate and efficient method for timely assessing the O3–VOCs-NOx relationships applicable in different O3 pollution events are essential. Here, we developed a novel machine learning ensemble model coupled with a Shapley additive explanation algorithm to predict the O3 formation regime and derive O3 formation sensitivity curves. The algorithm was tested for O3 events during the COVID-19 lockdown, a sandstorm event, and a heavy O3 pollution episode (maximum hourly O3 concentration >200 μg/m3) from 2019 to 2021. We show that increasing O3 concentrations during the COVID-19 lockdown and the heavy O3 pollution event were mainly caused by the photochemistry subject to local air quality and meteorological conditions. Influenced by the sandstorm weather, low O3 levels were mainly attributable to weak sunlight and low precursor levels. O3 formation sensitivity curves demonstrate that O3 formation in the study area was in a VOCs-sensitive regime. The VOCs-specific O3 sensitivity curves can also help make hybrid and timely strategies for O3 abatement. The results demonstrate that machine learning driven by observational data has the potential to be a very useful tool in predicting and interpreting O3 formation.
更多查看译文
关键词
Ground-level ozone (O3),O3 formation sensitivity,Pollution episodes,Shapley algorithm,Ensemble machine learning
AI 理解论文
溯源树
样例
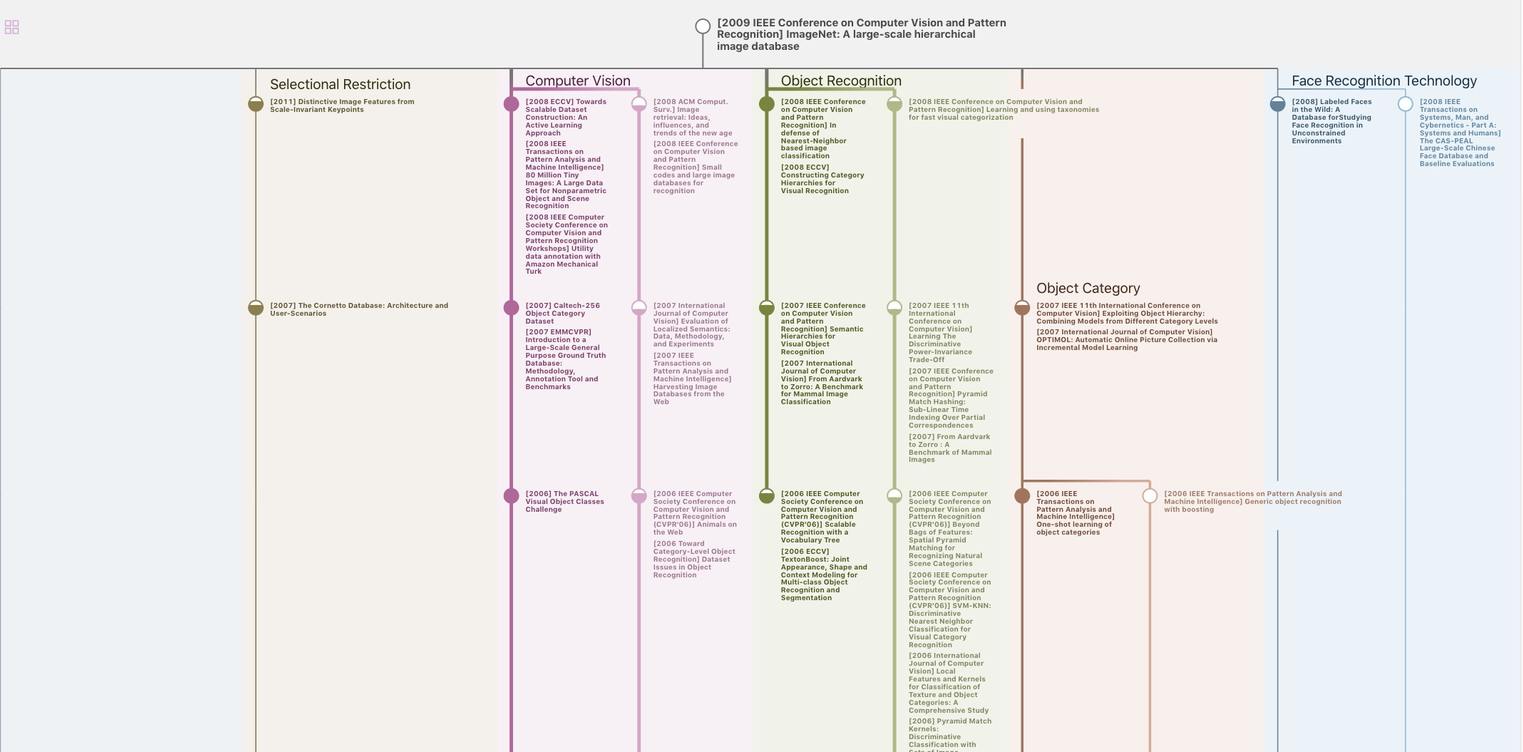
生成溯源树,研究论文发展脉络
Chat Paper
正在生成论文摘要