Curriculum-Style Fine-Grained Adaption for Unsupervised Cross-Lingual Dependency Transfer
IEEE/ACM Transactions on Audio, Speech, and Language Processing(2023)
摘要
Unsupervised cross-lingual transfer has been shown great potentials for dependency parsing of the low-resource languages when there is no annotated treebank available. Recently, the self-training method has received increasing interests because of its state-of-the-art performance in this scenario. In this work, we advance the method further by coupling it with curriculum learning, which guides the self-training in an easy-to-hard manner. Concretely, we present a novel metric to measure the instance difficulty of a dependency parser which is trained mainly on a Treebank from a resource-rich source language. By using the metric, we divide a low-resource target language into several fine-grained sub-languages by their difficulties, and then apply iterative-self-training progressively on these sub-languages. To fully explore the auto-parsed training corpus from sub-languages, we exploit an improved parameter generation network to model the sub-languages for better representation learning. Experimental results show that our final curriculum-style self-training can outperform a range of strong baselines, leading to new state-of-the-art results on unsupervised cross-lingual dependency parsing. We also conduct detailed experimental analyses to examine the proposed approach in depth for comprehensive understandings.
更多查看译文
关键词
Cross-lingual dependency parsing,curriculum learning,self-training
AI 理解论文
溯源树
样例
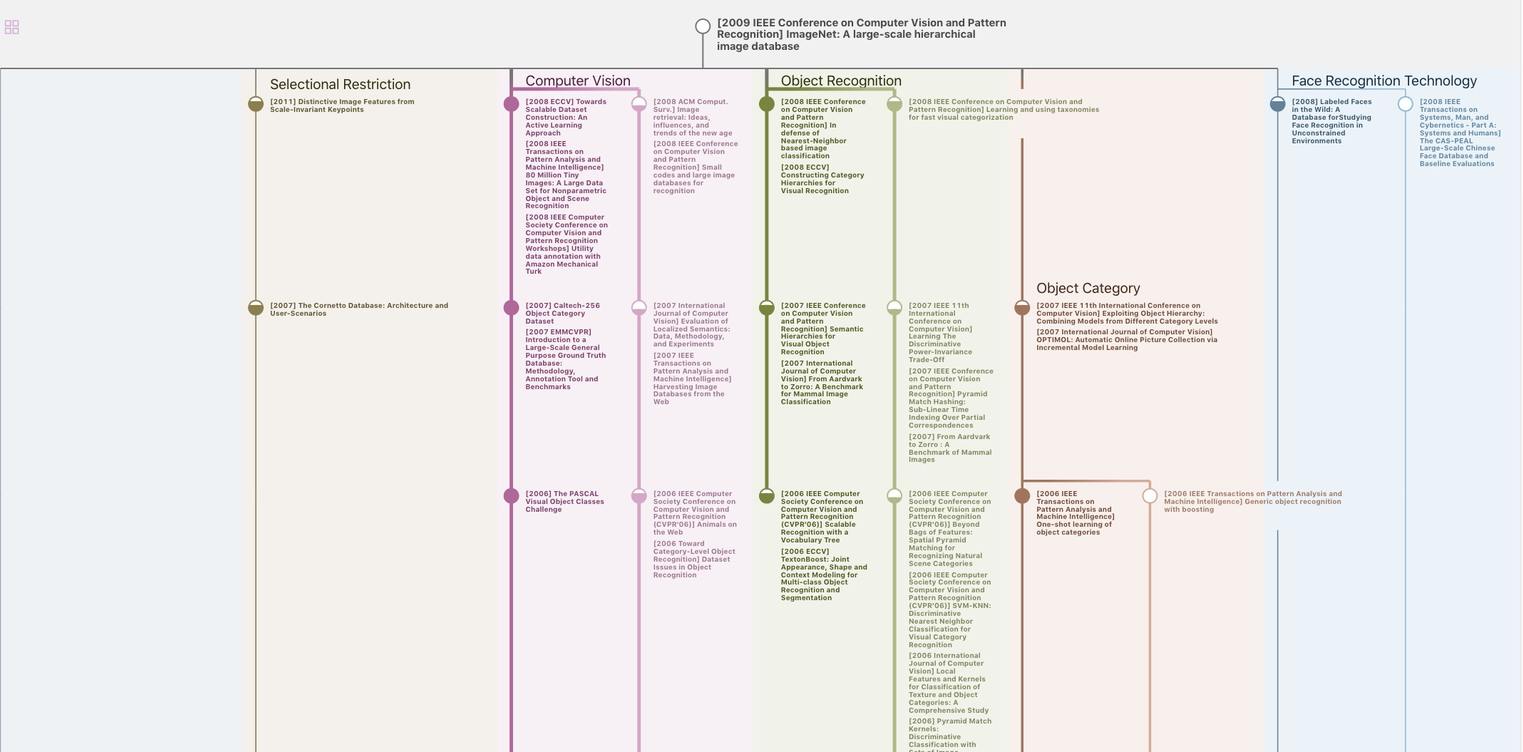
生成溯源树,研究论文发展脉络
Chat Paper
正在生成论文摘要