Approximate Boltzmann Distributions in Quantum Approximate Optimization
arxiv(2023)
Abstract
Approaches to compute or estimate the output probability distributions from the quantum approximate optimization algorithm (QAOA) are needed to assess the likelihood it will obtain a quantum computational advantage. We analyze output from QAOA circuits solving 7,200 random MaxCut instances, with $n=14-23$ qubits and depth parameter $p \leq 12$, and find that the average basis state probabilities follow approximate Boltzmann distributions: The average probabilities scale exponentially with their energy (cut value), with a peak at the optimal solution. The rate of exponential scaling or ``effective temperature" $T$ is found to scale as $T \sim C_\mathrm{min}/n\sqrt{p}$, with $C_\mathrm{min}$ the optimal solution energy. Using this scaling we generate approximate output distributions with up to 38 qubits and find these give accurate accounts of important performance metrics in cases we can simulate exactly. Based on the observed scalings we estimate that high-fidelity circuits involving hundreds of algorithmic layers will be needed to identify optimal solutions for problems with hundreds of qubits.
MoreTranslated text
AI Read Science
Must-Reading Tree
Example
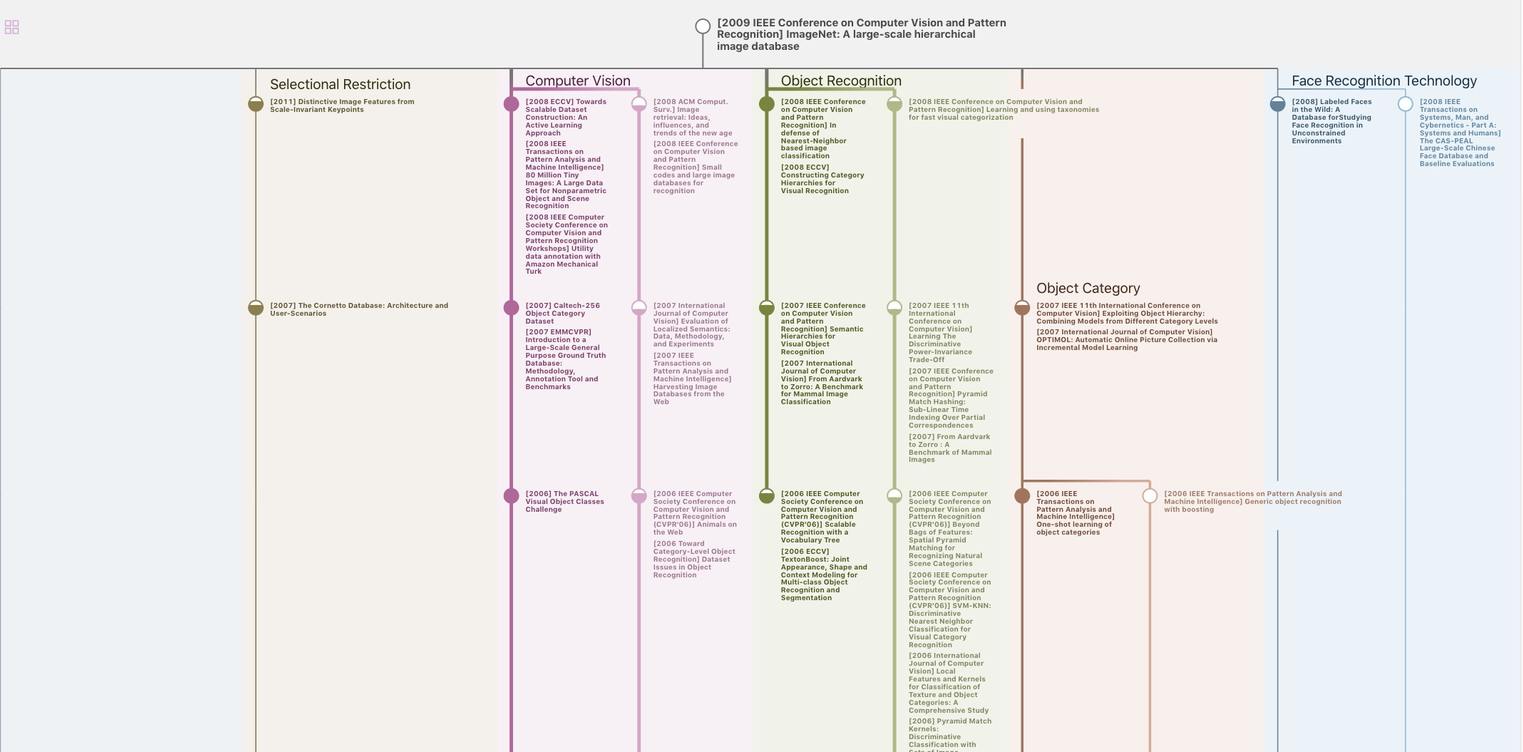
Generate MRT to find the research sequence of this paper
Chat Paper
Summary is being generated by the instructions you defined