Operator inference with roll outs for learning reduced models from scarce and low-quality data
COMPUTERS & MATHEMATICS WITH APPLICATIONS(2023)
摘要
Data-driven modeling has become a key building block in computational science and engineering. However, data that are available in science and engineering are typically scarce, often polluted with noise and affected by measurement errors and other perturbations, which makes learning the dynamics of systems challenging. In this work, we propose to combine data-driven modeling via operator inference with the dynamic training via roll outs of neural ordinary differential equations. Operator inference with roll outs inherits interpretability, scalability, and structure preservation of traditional operator inference while leveraging the dynamic training via roll outs over multiple time steps to increase stability and robustness for learning from low-quality and noisy data. Numerical experiments with data describing shallow water waves and surface quasi-geostrophic dynamics demonstrate that operator inference with roll outs provides predictive models from training trajectories even if data are sampled sparsely in time and polluted with noise of up to 10%.
更多查看译文
关键词
Data-driven modeling,Scientific machine learning,Model reduction,Scarce and noisy data
AI 理解论文
溯源树
样例
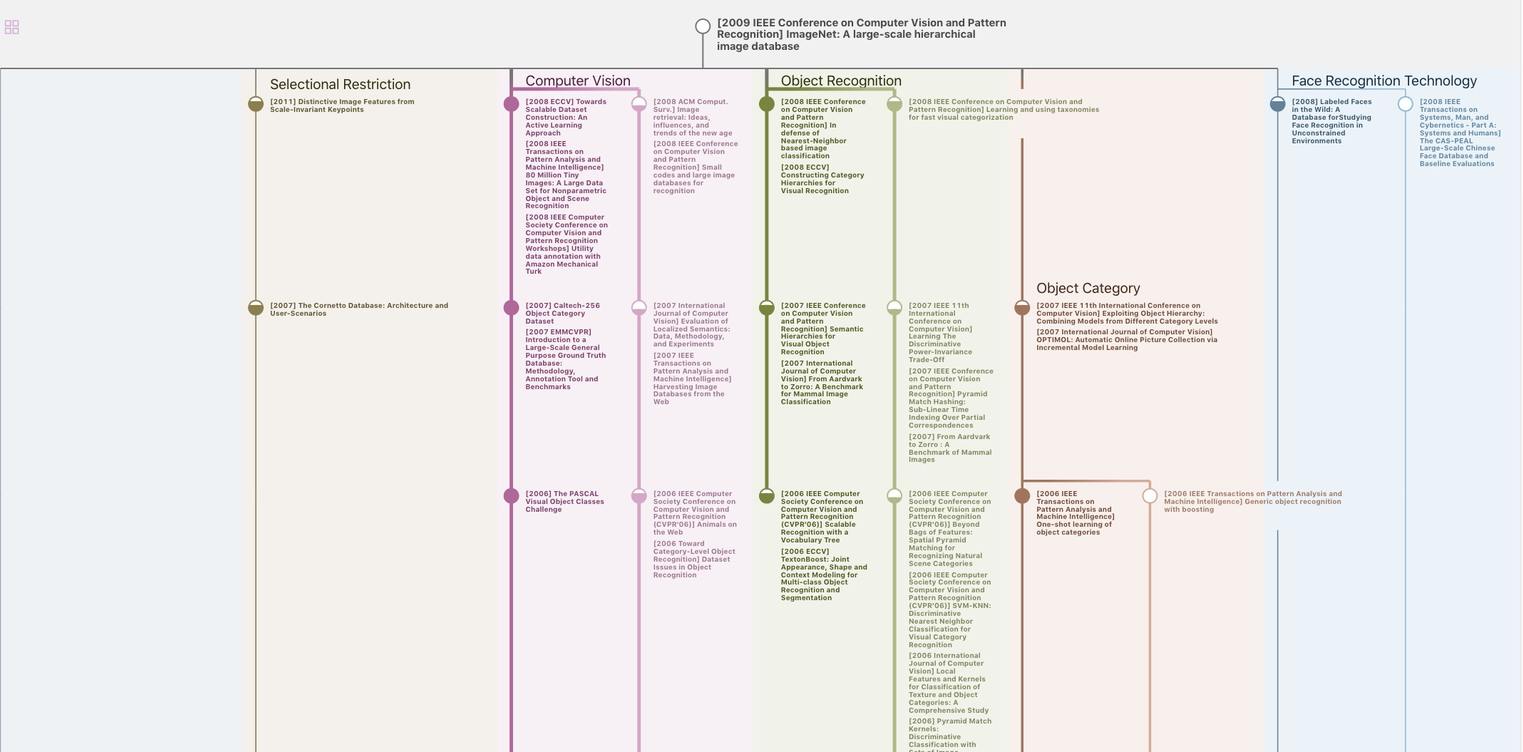
生成溯源树,研究论文发展脉络
Chat Paper
正在生成论文摘要