Convolution, aggregation and attention based deep neural networks for accelerating simulations in mechanics
arxiv(2023)
摘要
Deep learning surrogate models are being increasingly used in accelerating scientific simulations as a replacement for costly conventional numerical techniques. However, their use remains a significant challenge when dealing with real-world complex examples. In this work, we demonstrate three types of neural network architectures for efficient learning of highly non-linear deformations of solid bodies. The first two architectures are based on the recently proposed CNN U-NET and MAgNET (graph U-NET) frameworks which have shown promising performance for learning on mesh-based data. The third architecture is Perceiver IO, a very recent architecture that belongs to the family of attention-based neural networks-a class that has revolutionised diverse engineering fields and is still unexplored in computational mechanics. We study and compare the performance of all three networks on two benchmark examples, and show their capabilities to accurately predict the non-linear mechanical responses of soft bodies.
更多查看译文
关键词
surrogate modeling,deep learning-artificial neural network,CNN U-NET,graph U-net,perceiver IO,finite element method
AI 理解论文
溯源树
样例
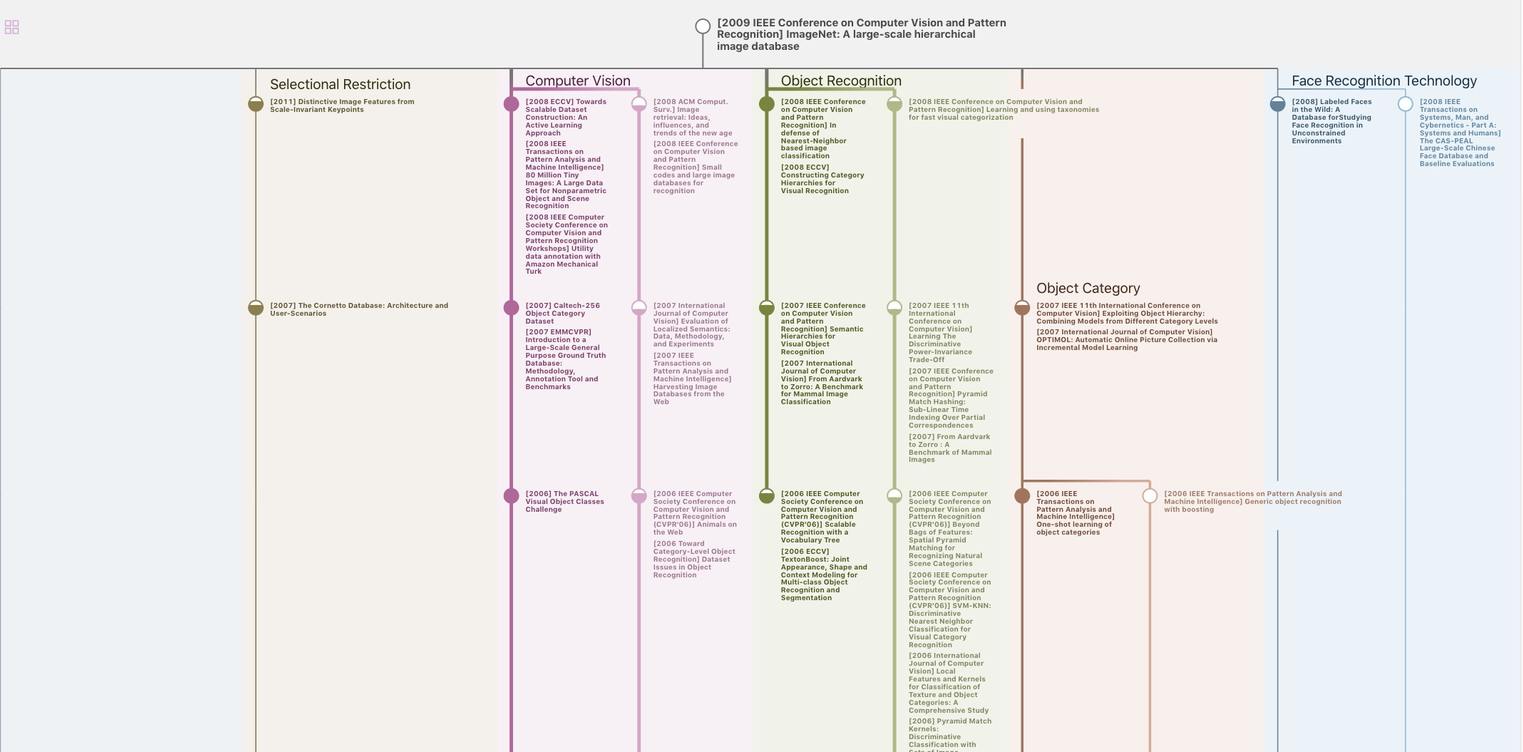
生成溯源树,研究论文发展脉络
Chat Paper
正在生成论文摘要