Channel attention generative adversarial network for super-resolution of glioma magnetic resonance image.
Computer methods and programs in biomedicine(2022)
Abstract
BACKGROUND AND OBJECTIVE:Glioma is the most common primary craniocerebral tumor caused by the cancelation of glial cells in the brain and spinal cord, with a high incidence and cure rate. Magnetic resonance imaging (MRI) is a common technique for detecting and analyzing brain tumors. Due to improper hardware and operation, the obtained brain MRI images are low-resolution, making it difficult to detect and grade gliomas accurately. However, super-resolution reconstruction technology can improve the clarity of MRI images and help experts accurately detect and grade glioma.
METHODS:We propose a glioma magnetic resonance image super-resolution reconstruction method based on channel attention generative adversarial network (CGAN). First, we replace the base block of SRGAN with a residual dense block based on the channel attention mechanism. Second, we adopt a relative average discriminator to replace the discriminator in standard GAN. Finally, we add the mean squared error loss to the training, consisting of the mean squared error loss, the L1 norm loss, and the generator's adversarial loss to form the generator loss function.
RESULTS:On the Set5, Set14, Urban100, and glioma datasets, compared with the state-of-the-art algorithms, our proposed CGAN method has improved peak signal-to-noise ratio and structural similarity, and the reconstructed glioma images are more precise than other algorithms.
CONCLUSION:The experimental results show that our CGAN method has apparent improvements in objective evaluation indicators and subjective visual effects, indicating its effectiveness and superiority.
MoreTranslated text
AI Read Science
Must-Reading Tree
Example
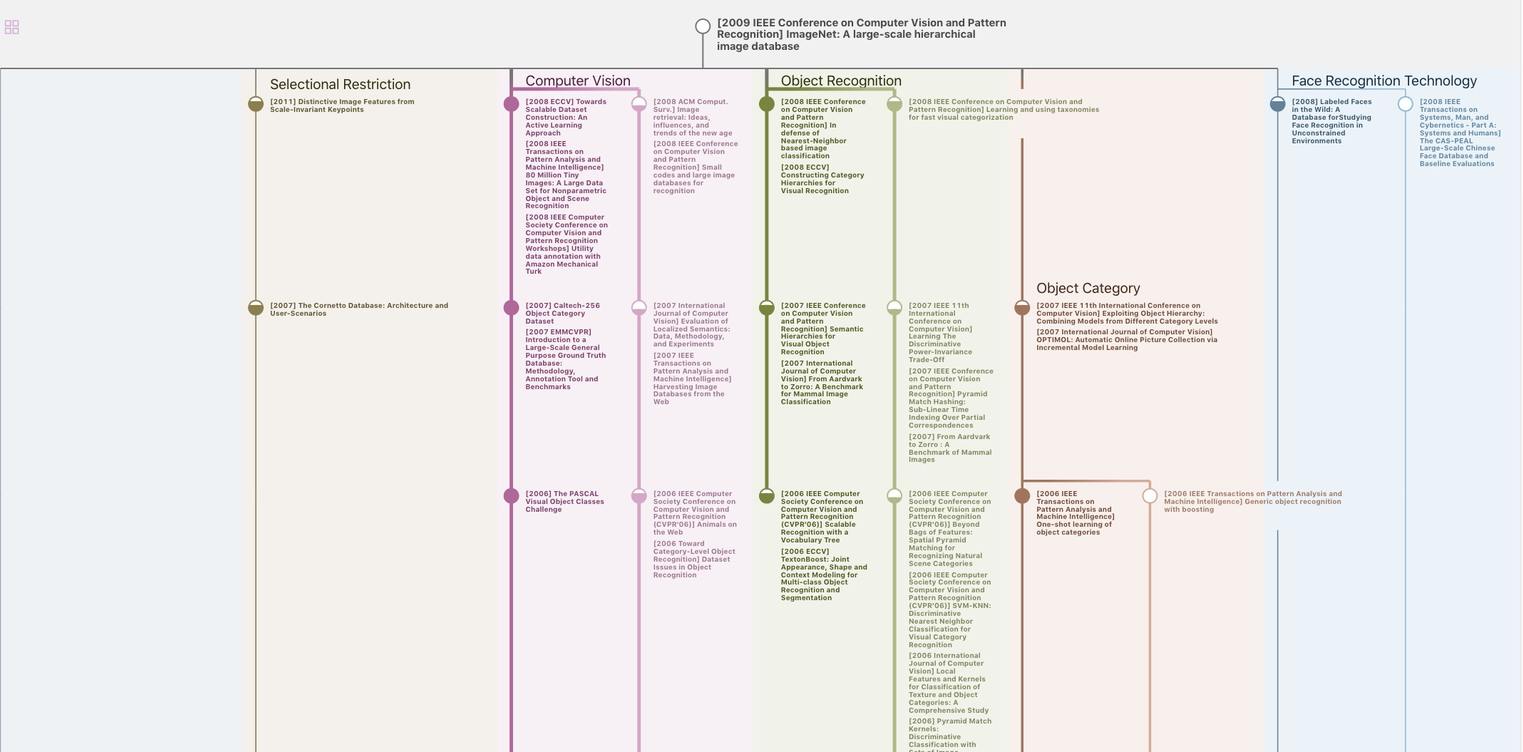
Generate MRT to find the research sequence of this paper
Chat Paper
Summary is being generated by the instructions you defined