Dimensionality-Reduction Methods for the Analysis of Web Traffic
International Joint Conference 15th International Conference on Computational Intelligence in Security for Information Systems (CISIS 2022) 13th International Conference on EUropean Transnational Education (ICEUTE 2022)(2022)
摘要
One of the usual targets for attackers are websites. Thus, protecting such assets is a key issue and consequently, a great effort has been devoted so far to address this problem. However, scant attention has been paid to investigate the contribution of unsupervised machine learning to the analysis of web traffic in order to detect attacks. To bridge this gap, the present paper proposes the novel application of dimensionality reduction methods to generate intuitive visualizations that can support the visual analysis of web traffic. More precisely, Laplacian Eigenmap, Isomap, t-Distributed Stochastic Neighbor Embedding, and Beta Hebbian Learning have been benchmarked. Promising results have been obtained on the standard CSIC2010 v2 dataset, encouraging further research on this topic.
更多查看译文
关键词
Cybersecurity, Web attacks, Unsupervised learning, Exploratory projection, Visual analysis
AI 理解论文
溯源树
样例
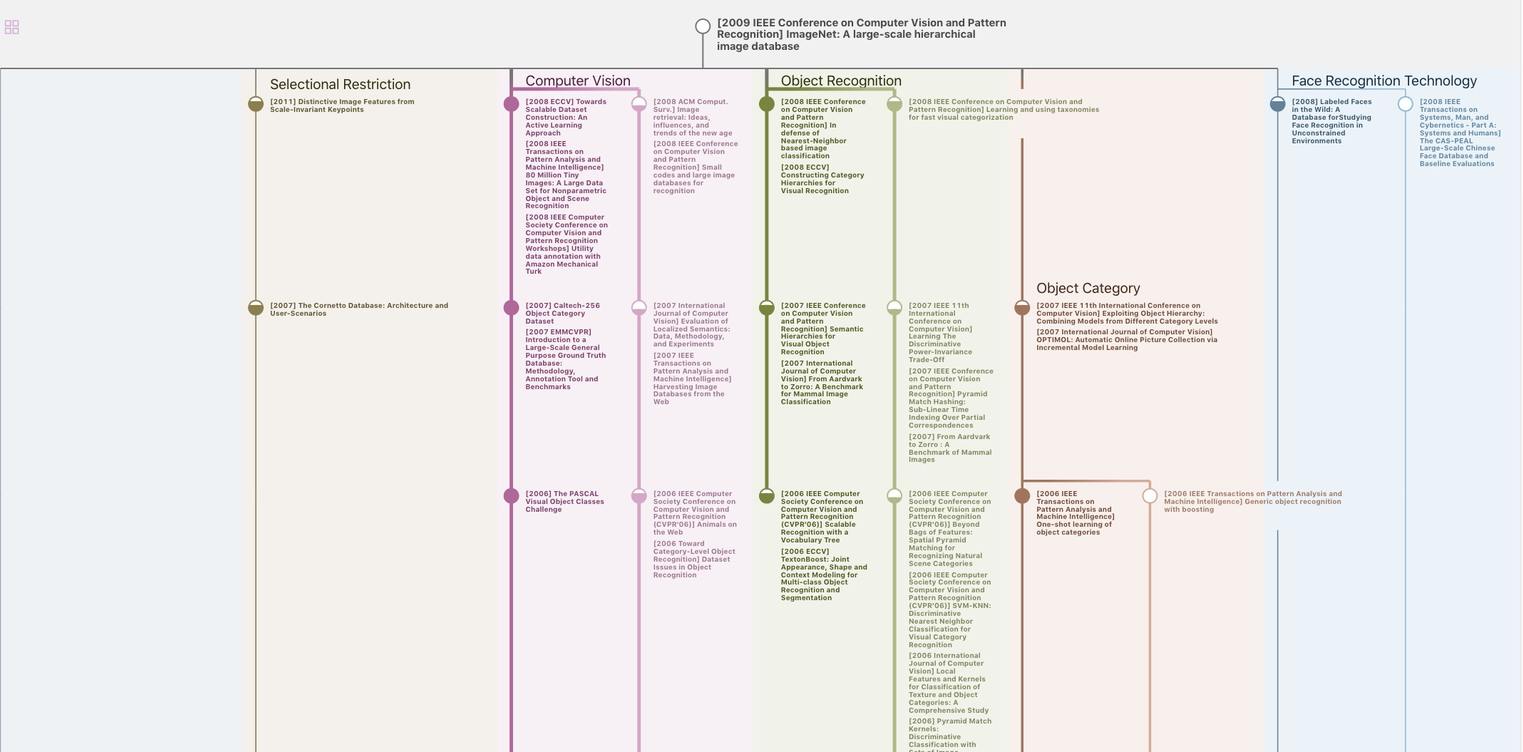
生成溯源树,研究论文发展脉络
Chat Paper
正在生成论文摘要