Function Placement and Acceleration for In-Network Federated Learning Services
2022 18th International Conference on Network and Service Management (CNSM)(2022)
Abstract
Edge intelligence combined with federated learning is considered as a way to distributed learning and inference tasks in a scalable way, by analyzing data close to where it is generated, unlike traditional cloud computing where data is offloaded to remote servers. In this paper, we address the placement of Artificial Intelligence Functions (AIF) making use of federated learning and hardware acceleration. We model the behavior of federated learning and related inference point to guide the placement decision, taking into consideration the specific constraint and the empirical behavior of a virtualized infrastructure anomaly detection use-case. Besides hardware acceleration, we consider the specific training time trend when distributing training over a network, by using empirical piece-wise linear distributions. We model the placement problem as a MILP and we propose a variant of the problem. Simulation results show the impact that hardware acceleration can have in the decision of the number of AIF to enable, while dividing by a relevant factor the distributed training time. We also show how our approach exacerbates the importance of monitoring an end-to-end learning system delay budget composed of link propagation delay and distributed training time in the location of AIFs.
MoreTranslated text
Key words
Artificial intelligence function,network management,federated learning
AI Read Science
Must-Reading Tree
Example
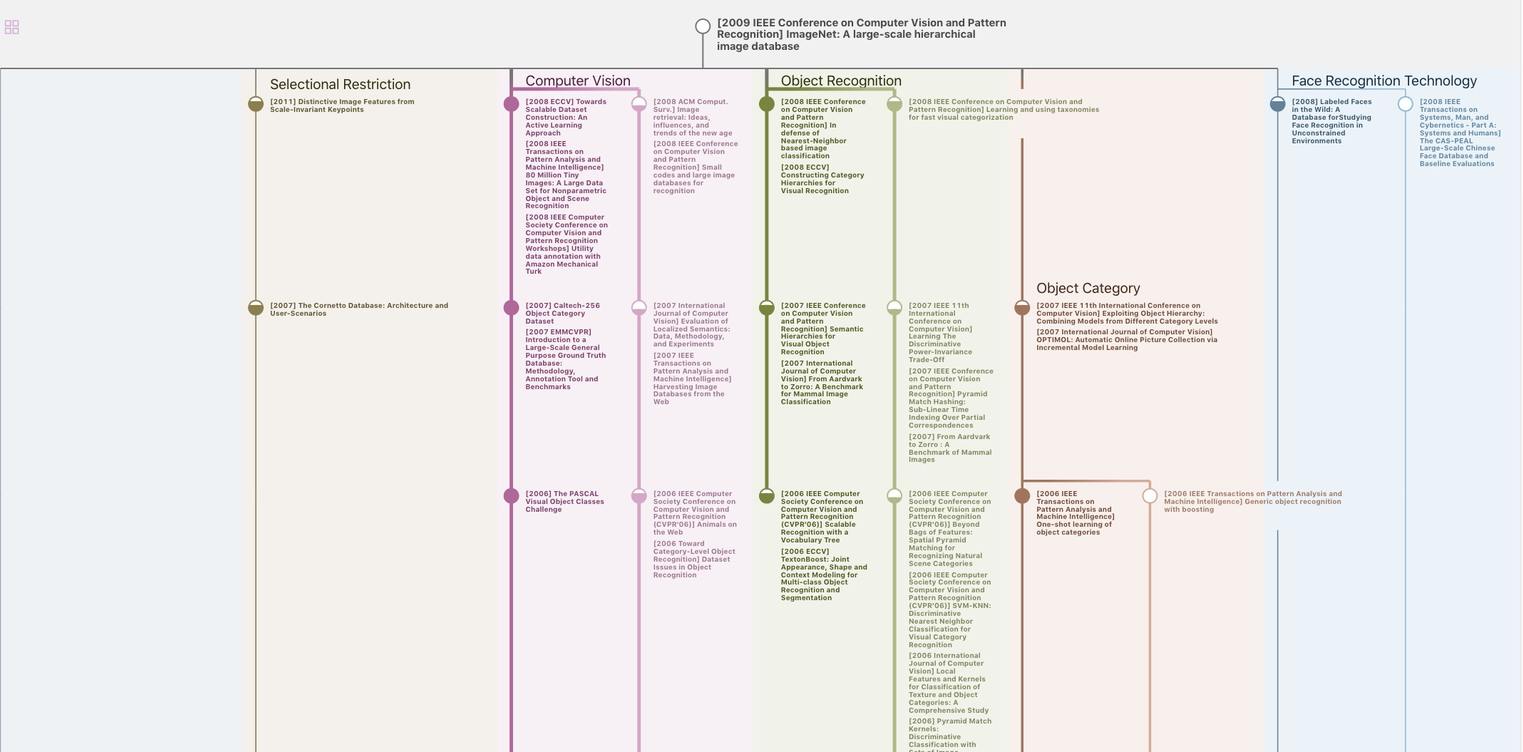
Generate MRT to find the research sequence of this paper
Chat Paper
Summary is being generated by the instructions you defined