Tailored 3D CT contrastive pretraining to improve pulmonary pathology classification
2022 16th IEEE International Conference on Signal Processing (ICSP)(2022)
摘要
Learning useful representations is a key task for supervised, unsupervised, and self-supervised algorithms. These latter methods have shown great promise for learning meaningful visual representations from unlabeled data, which can then be readily used for downstream tasks (e.g., classification). Recently proposed contrastive self-supervised learning methods have shown high performance on natural images. In this work, we show the usefulness of such approaches in the medical domain when used for 3D chest CT patch pathology classification. We observe that pretraining on unlabeled domain-specific medical images using contrastive self-supervised learning with specific data transformation significantly improves the accuracy of our 3D patch-based pathology classifiers. Specifically, we show that contrastive pretraining outperforms end-to-end supervised training by a large margin (weighted accuracy: 90.33 % vs 77.75 %, respectively).
更多查看译文
关键词
Self-supervised learning,Contrastive learning,Pathology classification,Medical imaging
AI 理解论文
溯源树
样例
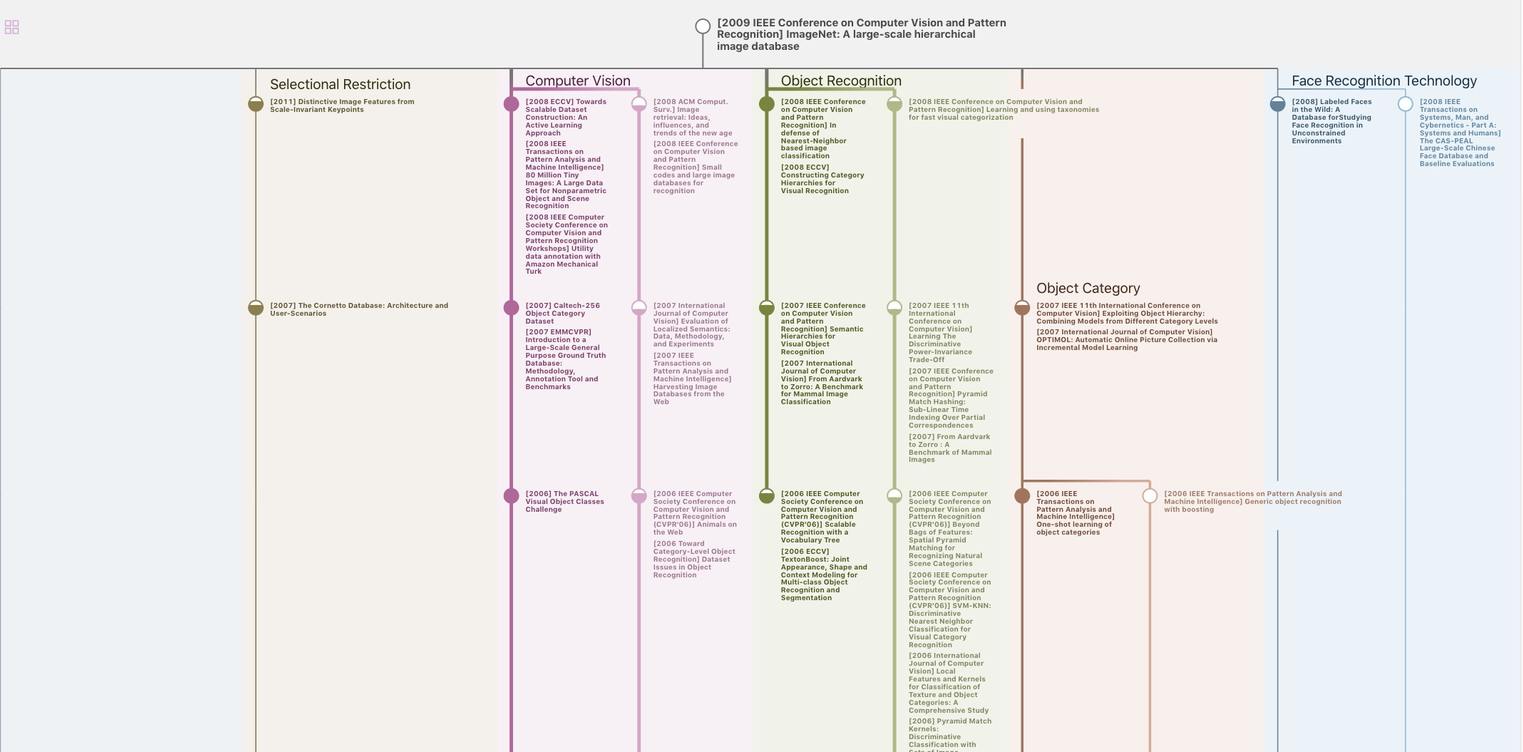
生成溯源树,研究论文发展脉络
Chat Paper
正在生成论文摘要