Spectral Network Combining Fourier Transformation and Deep Learning for Remote Sensing Object Detection
2022 International Conference on Electrical Engineering and Photonics (EExPolytech)(2022)
摘要
While general object detection with deep learning techniques has garnered immense progress, the performance of detecting small objects in remote sensing is far from satisfactory due to the lack of sufficient details. To address this problem, this paper designs a spectral network combining Fast Fourier Convolution (FFC) and detection network. By extending the receptive region of the network, features around the object are introduced as additional information to help detect small objects. Specifically, the FFC in the proposed spectral network extracts global features through its unique Fourier Unit: the spatial feature is first transformed into the frequency domain by the Fast Fourier transform (FFT), then a convolution block is performed to extract the frequency features, obviously this frequency-aware convolution block has a global receptive field in the spatial domain covering the whole image, and finally the features are recovered to the spatial domain using the inverse FFT. To demonstrate the effectiveness of our approach, we conduct experiments on the large remote sensing dataset DIOR, which shows that our approach has excellent performance compared to other detectors. It achieves an average accuracy (mAP) of 73.5% without any tricks.
更多查看译文
关键词
remote sensing,object detection,deep learning,spectral networks,Fourier transform
AI 理解论文
溯源树
样例
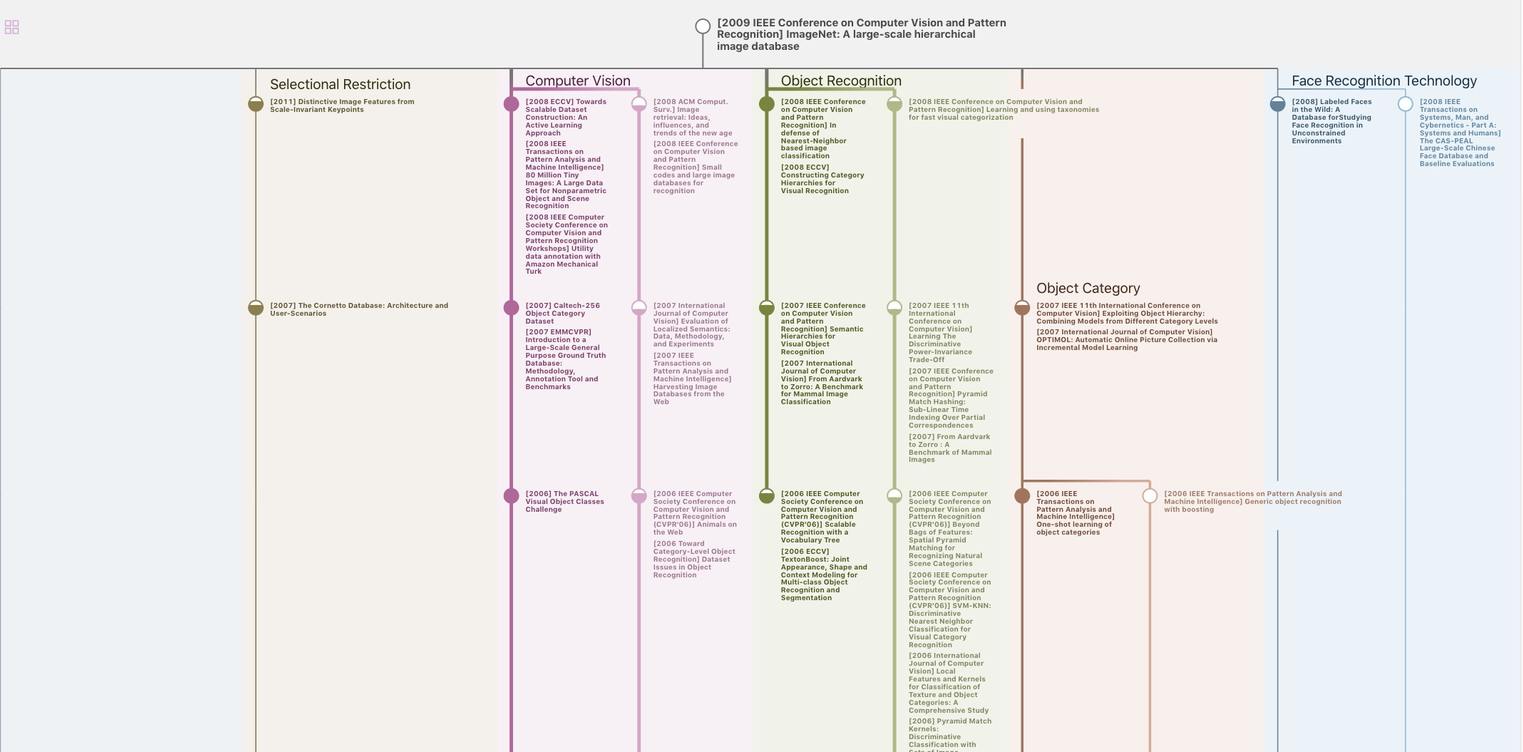
生成溯源树,研究论文发展脉络
Chat Paper
正在生成论文摘要