Video-based Pose-Estimation Data as Source for Transfer Learning in Human Activity Recognition.
ICPR(2022)
摘要
Human Activity Recognition (HAR) using on-body devices identifies specific human actions in unconstrained environments. HAR is challenging due to the inter and intra-variance of human movements; moreover, annotated datasets from on-body devices are scarce. This problem is mainly due to the difficulty of data creation, i.e., recording, expensive annotation, and lack of standard definitions of human activities. Previous works demonstrated that transfer learning is a good strategy for addressing scenarios with scarce data. However, the scarcity of annotated on-body device datasets remains. This paper proposes using datasets intended for human-pose estimation as a source for transfer learning; specifically, it deploys sequences of annotated pixel coordinates of human joints from video datasets for HAR and human pose estimation. We pre-train a deep architecture on four benchmark video-based source datasets. Finally, an evaluation is carried out on three on-body device datasets improving HAR performance.
更多查看译文
关键词
annotated on-body device datasets,annotated pixel coordinates,benchmark video-based source datasets,data creation,deep architecture,HAR performance,human activity recognition,human joints,human movements,human pose estimation,transfer learning,video-based pose-estimation data
AI 理解论文
溯源树
样例
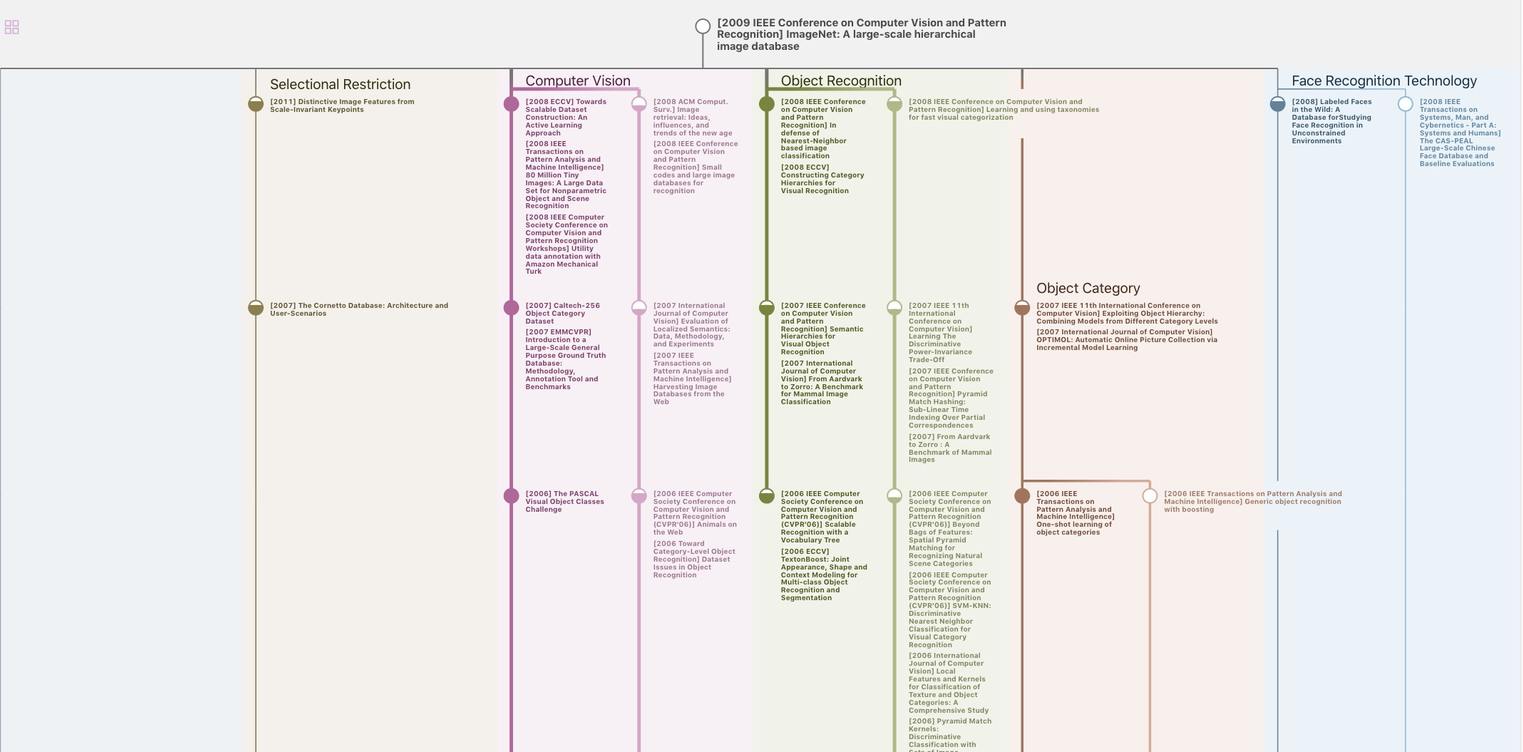
生成溯源树,研究论文发展脉络
Chat Paper
正在生成论文摘要