Active learning framework for tunnel geological reconstruction based on TBM operational data
AUTOMATION IN CONSTRUCTION(2024)
摘要
Exploring the geological information of construction tunnels is an important issue in a tunnel boring machine (TBM)-based underground project. This paper proposes an active learning framework with an incremental generative adversarial network (AL-iGAN) for reconstructing the previous geological profile of a tunnel after construction based on TBM operational data. The framework consists of two main strategies: one applies active learning techniques to recommend new drilling locations for labeling TBM operational data as new training samples; and the other incrementally updates the weights of iGAN for geological reconstruction (iGAN-GR) to improve the reconstruction performance by using these new samples. Numerical experiments show that the proposed framework can accurately estimate the thickness of each rock soil type appearing in a tunnel before construction, and the new training samples recommended by active learning significantly improve the reconstruction accuracy. The provided knowledge of the rock soil types distributed around the tunnel before construction is conducive to developing an effective strategy for the health detection and assessment of the tunnel after construction. The AL-iGAN framework has good generalizability and can be applied to detect geological conditions in other underground engineering projects.
更多查看译文
关键词
Tunnel boring machine,Generative adversarial network,Active learning,Geological reconstruction,Incremental learning
AI 理解论文
溯源树
样例
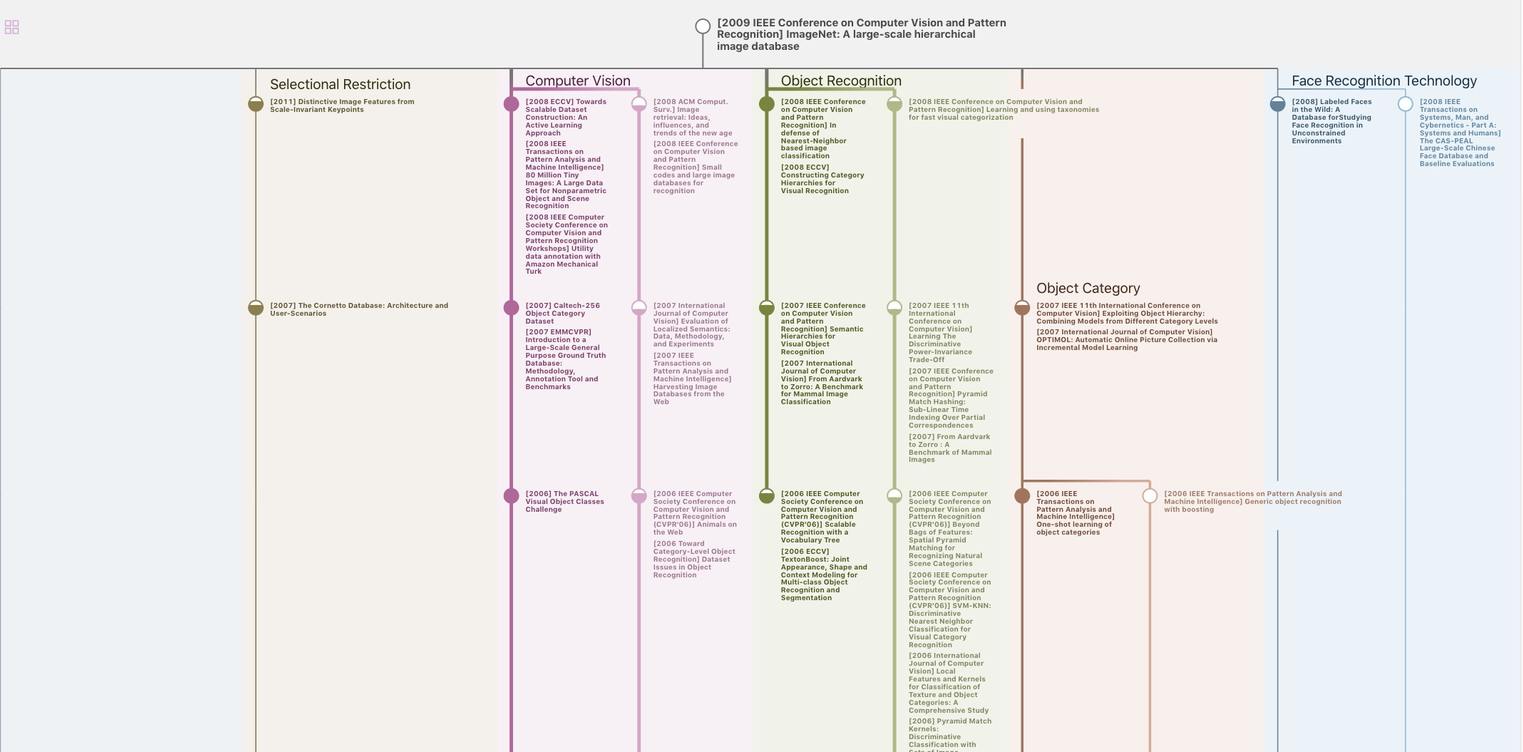
生成溯源树,研究论文发展脉络
Chat Paper
正在生成论文摘要