DisaggRec: Architecting Disaggregated Systems for Large-Scale Personalized Recommendation
arxiv(2022)
摘要
Deep learning-based personalized recommendation systems are widely used for online user-facing services in production datacenters, where a large amount of hardware resources are procured and managed to reliably provide low-latency services without disruption. As the recommendation models continue to evolve and grow in size, our analysis projects that datacenters deployed with monolithic servers will spend up to 12.4x total cost of ownership (TCO) to meet the requirement of model size and complexity over the next three years. Moreover, through in-depth characterization, we reveal that the monolithic server-based cluster suffers resource idleness and wastes up to 30% TCO by provisioning resources in fixed proportions. To address this challenge, we propose DisaggRec, a disaggregated system for large-scale recommendation serving. DisaggRec achieves the independent decoupled scaling-out of the compute and memory resources to match the changing demands from fast-evolving workloads. It also improves system reliability by segregating the failures of compute nodes and memory nodes. These two main benefits from disaggregation collectively reduce the TCO by up to 49.3%. Furthermore, disaggregation enables flexible and agile provisioning of increasing hardware heterogeneity in future datacenters. By deploying new hardware featuring near-memory processing capability, our evaluation shows that the disaggregated cluster achieves 21%-43.6% TCO savings over the monolithic server-based cluster across a three-year span of model evolution.
更多查看译文
关键词
large-scale
AI 理解论文
溯源树
样例
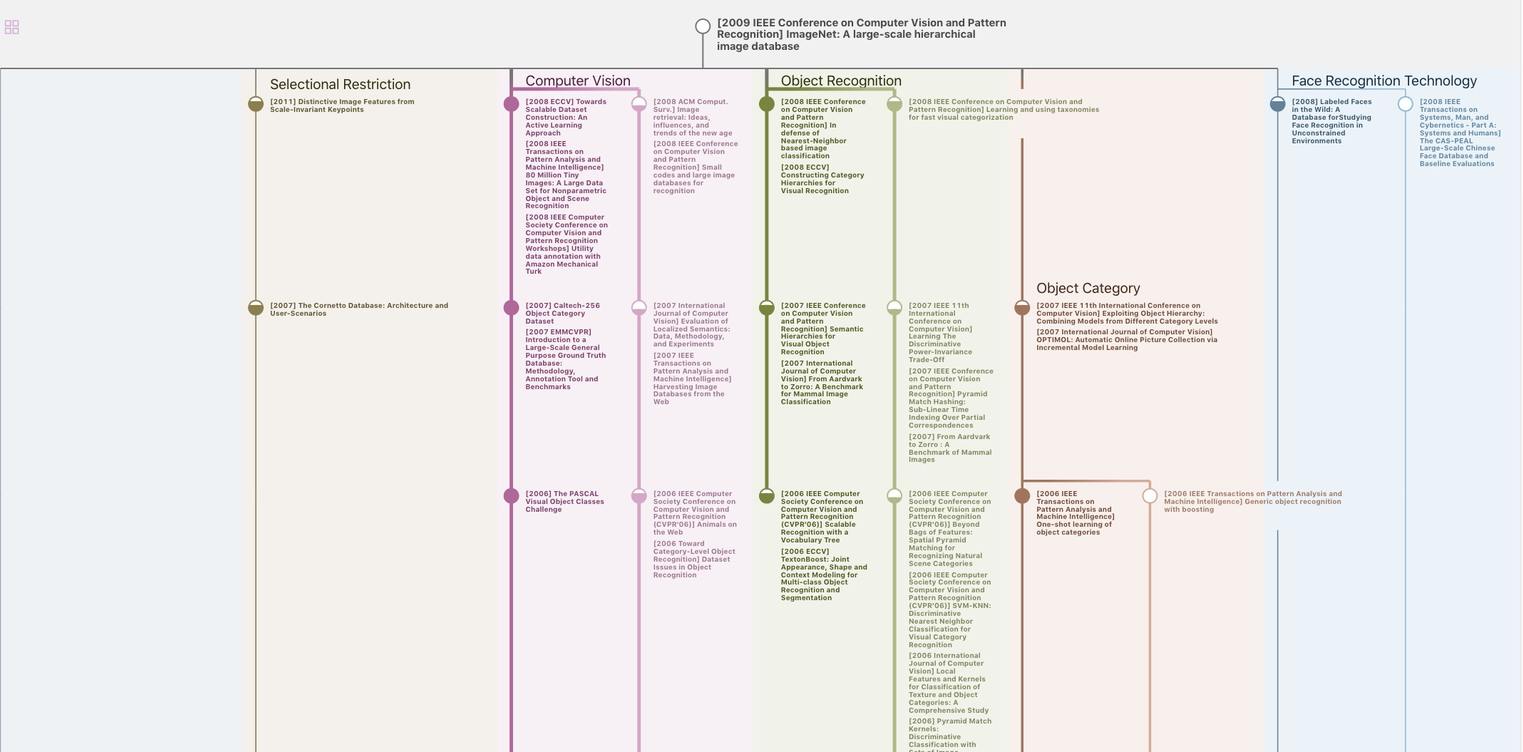
生成溯源树,研究论文发展脉络
Chat Paper
正在生成论文摘要