A Compound Loss Function with Shape Aware Weight Map for Microscopy Cell Segmentation
IEEE TRANSACTIONS ON MEDICAL IMAGING(2023)
Key words
Image segmentation,Shape,Microscopy,Computer architecture,Compounds,Microprocessors,Annotations,Microscopy cell segmentation,deep learning,compound loss function,shape aware weight map
AI Read Science
Must-Reading Tree
Example
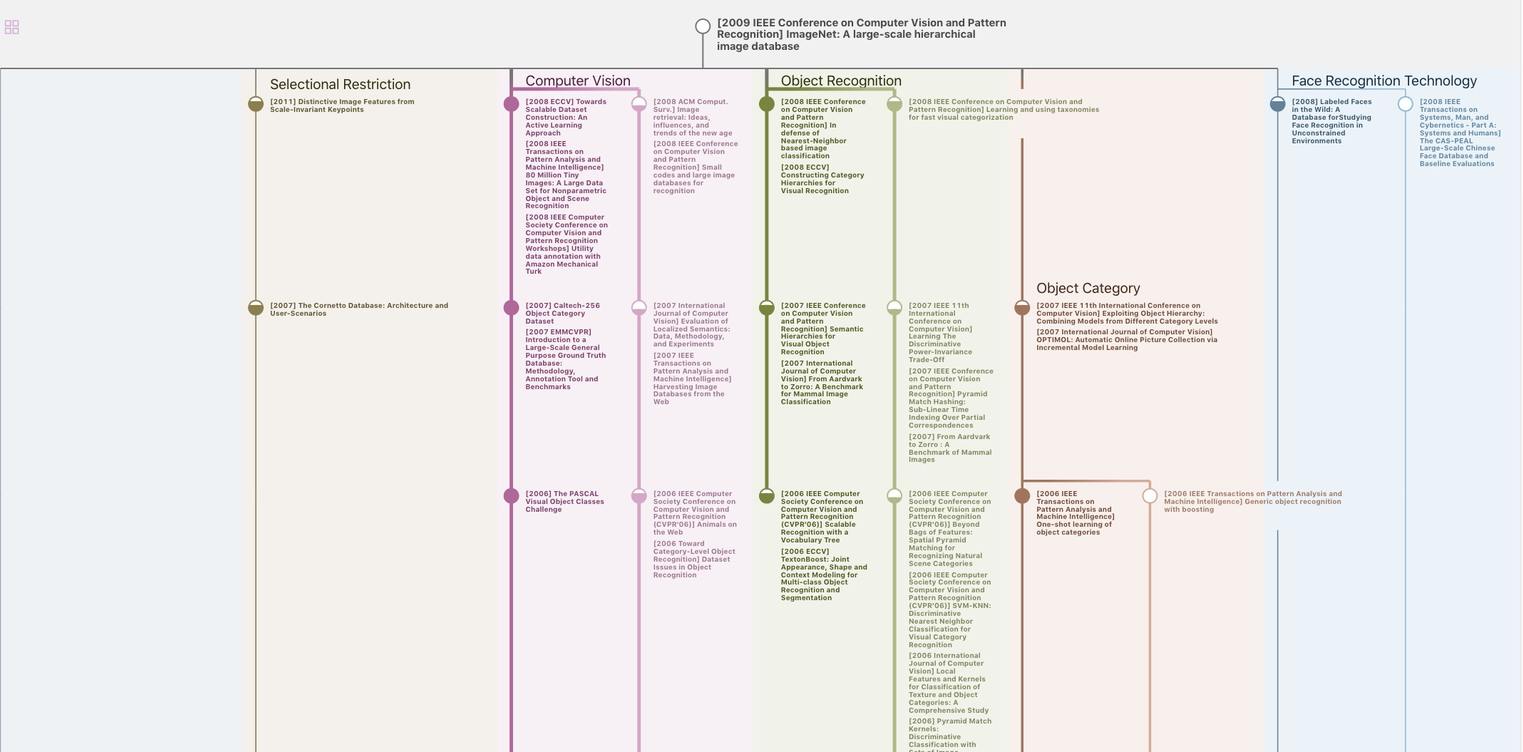
Generate MRT to find the research sequence of this paper
Chat Paper
Summary is being generated by the instructions you defined