Prediction of radiosensitivity and radiocurability using a novel supervised artificial neural network
BMC cancer(2022)
摘要
Background Radiotherapy has been widely used to treat various cancers, but its efficacy depends on the individual involved. Traditional gene-based machine-learning models have been widely used to predict radiosensitivity. However, there is still a lack of emerging powerful models, artificial neural networks (ANN), in the practice of gene-based radiosensitivity prediction. In addition, ANN may overfit and learn biologically irrelevant features. Methods We developed a novel ANN with Selective Connection based on Gene Patterns (namely ANN-SCGP) to predict radiosensitivity and radiocurability. We creatively used gene patterns (gene similarity or gene interaction information) to control the "on–off" of the first layer of weights, enabling the low-dimensional features to learn the gene pattern information. ANN-SCGP was trained and tested in 82 cell lines and 1,101 patients from the 11 pan-cancer cohorts. Results For survival fraction at 2 Gy, the root mean squared errors (RMSE) of prediction in ANN-SCGP was the smallest among all algorithms (mean RMSE: 0.1587–0.1654). For radiocurability, ANN-SCGP achieved the first and second largest C-index in the 12/20 and 4/20 tests, respectively. The low dimensional output of ANN-SCGP reproduced the patterns of gene similarity. Moreover, the pan-cancer analysis indicated that immune signals and DNA damage responses were associated with radiocurability. Conclusions As a model including gene pattern information, ANN-SCGP had superior prediction abilities than traditional models. Our work provided novel insights into radiosensitivity and radiocurability.
更多查看译文
关键词
Computational Biology,High-Throughput Sequencing,Multi-Omics,Neural Network Models,Radiosensitivity
AI 理解论文
溯源树
样例
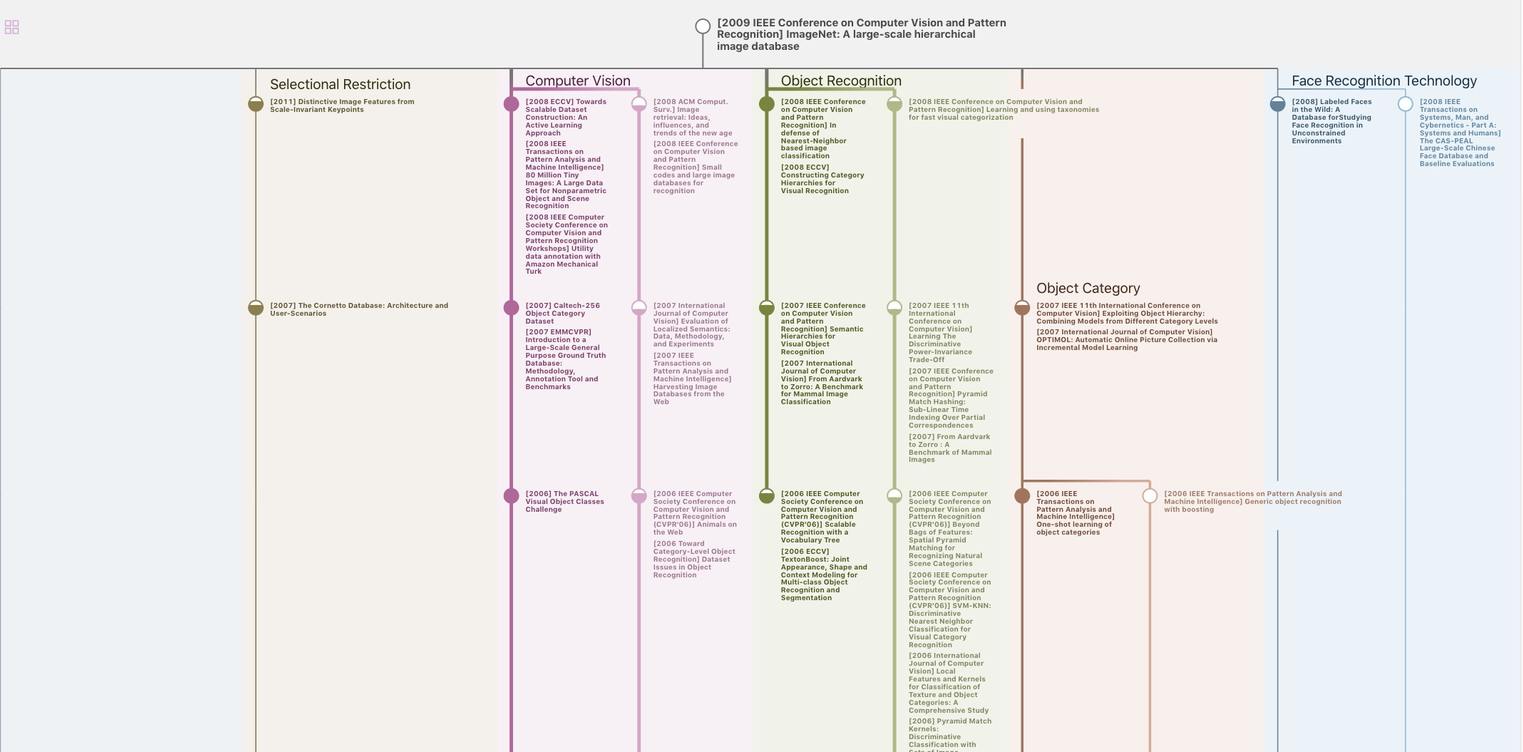
生成溯源树,研究论文发展脉络
Chat Paper
正在生成论文摘要