Does Inter-Protein Contact Prediction Benefit from Multi-Modal Data and Auxiliary Tasks?
biorxiv(2022)
摘要
Approaches to in silico prediction of protein structures have been revolutionized by AlphaFold2, while those to predict interfaces between proteins are relatively underdeveloped, owing to the overly complicated yet relatively limited data of protein–protein complexes. In short, proteins are 1D sequences of amino acids folding into 3D structures, and interact to form assemblies to function. We believe that such intricate scenarios are better modeled with additional indicative information that reflects their multi-modality nature and multi-scale functionality. To improve binary prediction of inter-protein residue-residue contacts, we propose to augment input features with multi-modal representations and to synergize the objective with auxiliary predictive tasks. ( i ) We first progressively add three protein modalities into models: protein sequences, sequences with evolutionary information, and structure-aware intra-protein residue contact maps. We observe that utilizing all data modalities delivers the best prediction precision . Analysis reveals that evolutionary and structural information benefit predictions on the difficult and rigid protein complexes, respectively, assessed by the resemblance to native residue contacts in bound complex structures. ( ii ) We next introduce three auxiliary tasks via self-supervised pre-training (binary prediction of protein-protein interaction (PPI)) and multi-task learning (prediction of inter-protein residue–residue distances and angles). Although PPI prediction is reported to benefit from predicting inter-contacts (as causal interpretations), it is not found vice versa in our study. Similarly, the finer-grained distance and angle predictions did not appear to uniformly improve contact prediction either. This again reflects the high complexity of protein–protein complex data, for which designing and incorporating synergistic auxiliary tasks remains challenging .
### Competing Interest Statement
The authors have declared no competing interest.
更多查看译文
AI 理解论文
溯源树
样例
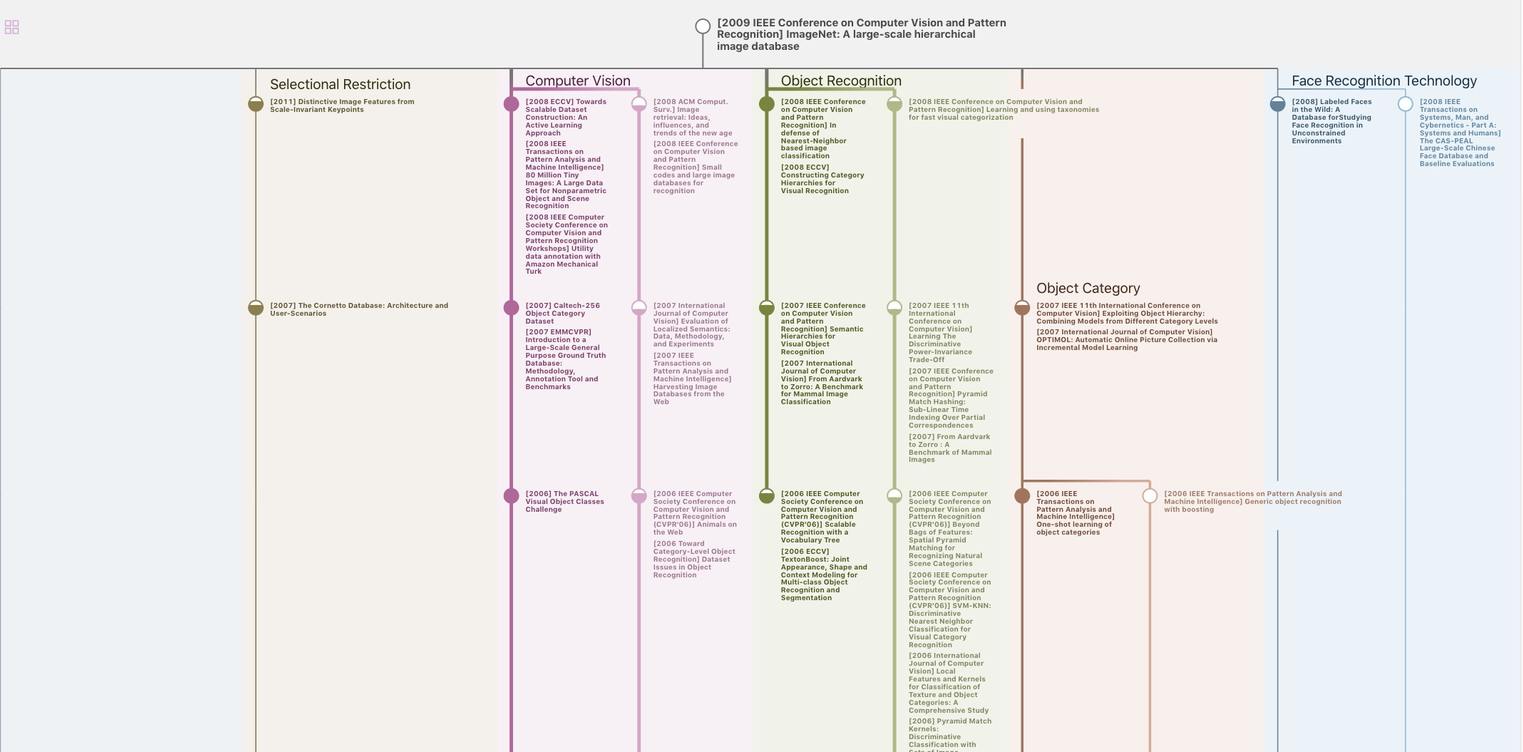
生成溯源树,研究论文发展脉络
Chat Paper
正在生成论文摘要