High resolution scene parsing network based on semantic segmentation
CHINESE JOURNAL OF LIQUID CRYSTALS AND DISPLAYS(2022)
Abstract
In order to efficiently segment and analyze complex scenes such as urban landscapes, this paper combines the high-resolution network (HRNet) and supplements the global context information through the pyramid pooling module, and proposes a high-resolution scene analysis network. Firstly, HRNet was used as the backbone feature extraction network, and the atrous separable convolution was used to improve its widely used residual module, so as to reduce the amount of parameters and improve the segmentation ability of multi-scale targets. Secondly, the mixed cavity convolution framework was used to design the multi-level cavity rate, which can dense the receptive field and reduce the influence of the grid problem. Then, a multi-stage continuous up-sampling structure was designed to improve the simple post fusion mechanism of HRNetV2. Finally, the improved pyramid pooling module which can adapt to different image resolutions was used to aggregate the context information of different regions to obtain high-quality segmentation images. The accuracy of 83. 3% MIOU is achieved with only 16. 4 Mbit parameters on the CityScapes urban landscape dataset, and good results are also achieved on the Camvid dataset. A more reliable, accurate, and low-computing scene analysis method based on semantic segmentation has realized.
MoreTranslated text
Key words
deep learning,neural network,semantic segmentation,high resolution network,atrous convolution
AI Read Science
Must-Reading Tree
Example
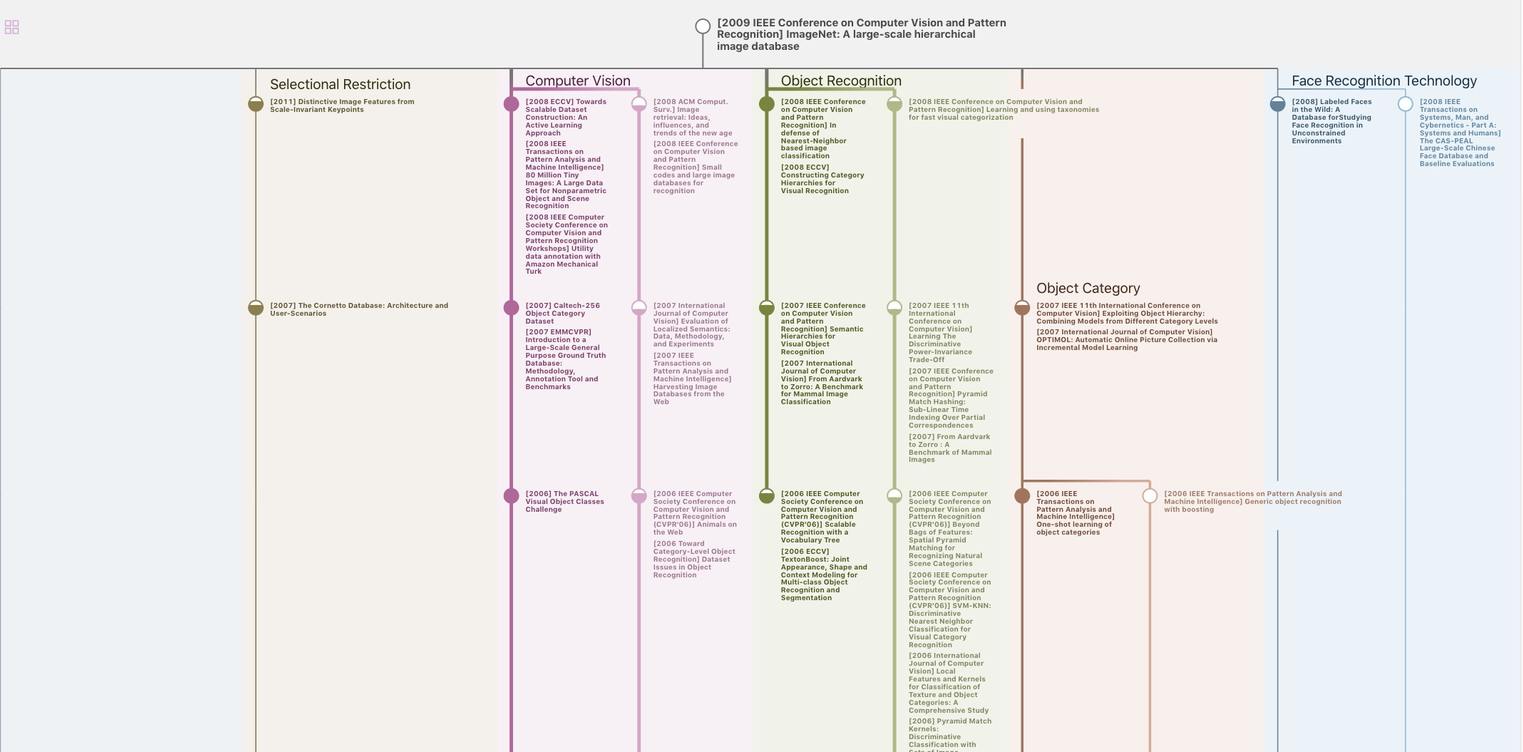
Generate MRT to find the research sequence of this paper
Chat Paper
Summary is being generated by the instructions you defined