Mask-Net: A Hardware-efficient Object Detection Network with Masked Region Proposals
2022 IEEE 33rd International Conference on Application-specific Systems, Architectures and Processors (ASAP)(2022)
摘要
Object detection on embedded systems is challenging because it is hard to achieve real-time inference with low energy consumption and limited hardware resources. Another challenge is to find hardware-friendly methods to avoid redundant computation. To address these challenges, in this work, we propose Mask-Net, a hardware-efficient object detection network with masked region proposals in regular shapes. First, we propose a hardware-friendly region proposal method to avoid redundant computation as much as possible and as early as possible, with slight or no accuracy loss. Second, we demonstrate that our method is generalizable by applying it to several detection backbones including SkyNet, ResNet-18 and UltraNet. Our method performs well in different scenarios, including DAC-SDC dataset, UAV123 dataset and OTB100 dataset. We choose SkyNet as our base model to design an accelerator and verify our design on Xilinx ZCU106 FPGA. We observe a speedup of 1.3× and about 30% energy consumption reduction when the FPGA runs at different frequencies from 124 MHz to 214 MHz with only a slight accuracy loss. We also conduct a design space exploration and demonstrate that our accelerator can achieve a theoretical speedup of 1.76× with masked region proposals. This is achieved by optimally allocating DSPs to different parts of the accelerator to balance the computations before and after the mask.
更多查看译文
关键词
embedded systems,object detection,DNN,region proposal,mask,FPGA
AI 理解论文
溯源树
样例
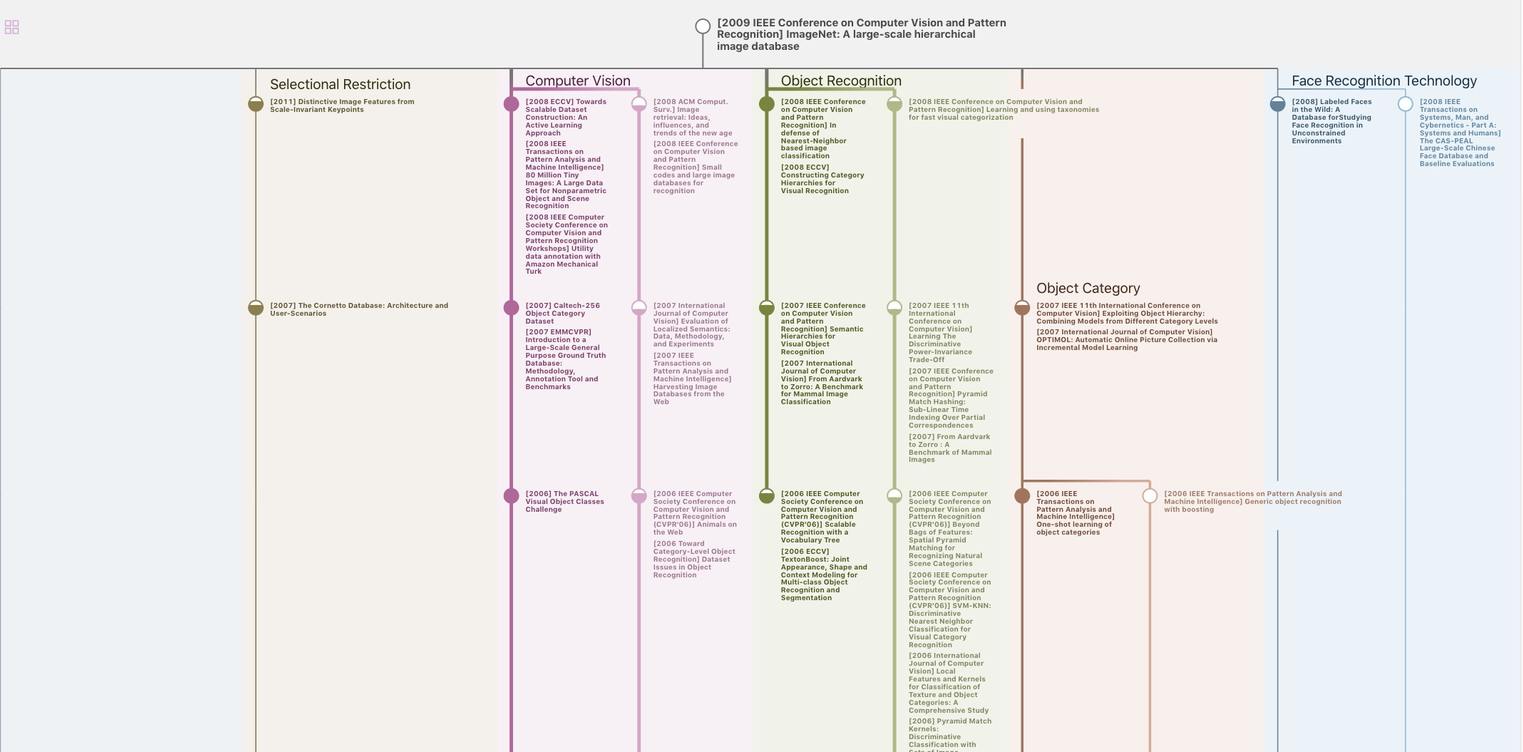
生成溯源树,研究论文发展脉络
Chat Paper
正在生成论文摘要