Low-precision logarithmic arithmetic for neural network accelerators
2022 IEEE 33rd International Conference on Application-specific Systems, Architectures and Processors (ASAP)(2022)
摘要
Resource requirements for hardware acceleration of neural networks inference is notoriously high, both in terms of computation and storage. One way to mitigate this issue is to quantize parameters and activations. This is usually done by scaling and centering the distributions of weights and activations, on a kernel per kernel basis, so that a low-precision binary integer representation can be used. This work studies low-precision logarithmic number system (LNS) as an efficient alternative. Firstly, LNS has more dynamic than fixed-point for the same number of bits. Thus, when quantizing MNIST and CIFAR reference networks without retraining, the smallest format size achieving top-1 accuracy comparable to floating-point is 1 to 3 bits smaller with LNS than with fixed-point. In addition, it is shown that the zero bit of classical LNS is not needed in this context, and that the sign bit can be saved for activations. The proposed LNS neuron is detailed and its implementation on FPGA is shown to be smaller and faster than a fixed-point one for comparable accuracy. Secondly, low-precision LNS enables efficient inference architectures where 1 / multiplications reduce to additions; 2/ the weighted inputs are converted to classical linear domain, but the tables needed for this conversion remain very small thanks to the low precision; and 3/ the conversion of the output activation back to LNS can be merged with an arbitrary activation function.
更多查看译文
关键词
neural network accelerator,arithmetic,Logarithmic Number System,FPGA,low precision
AI 理解论文
溯源树
样例
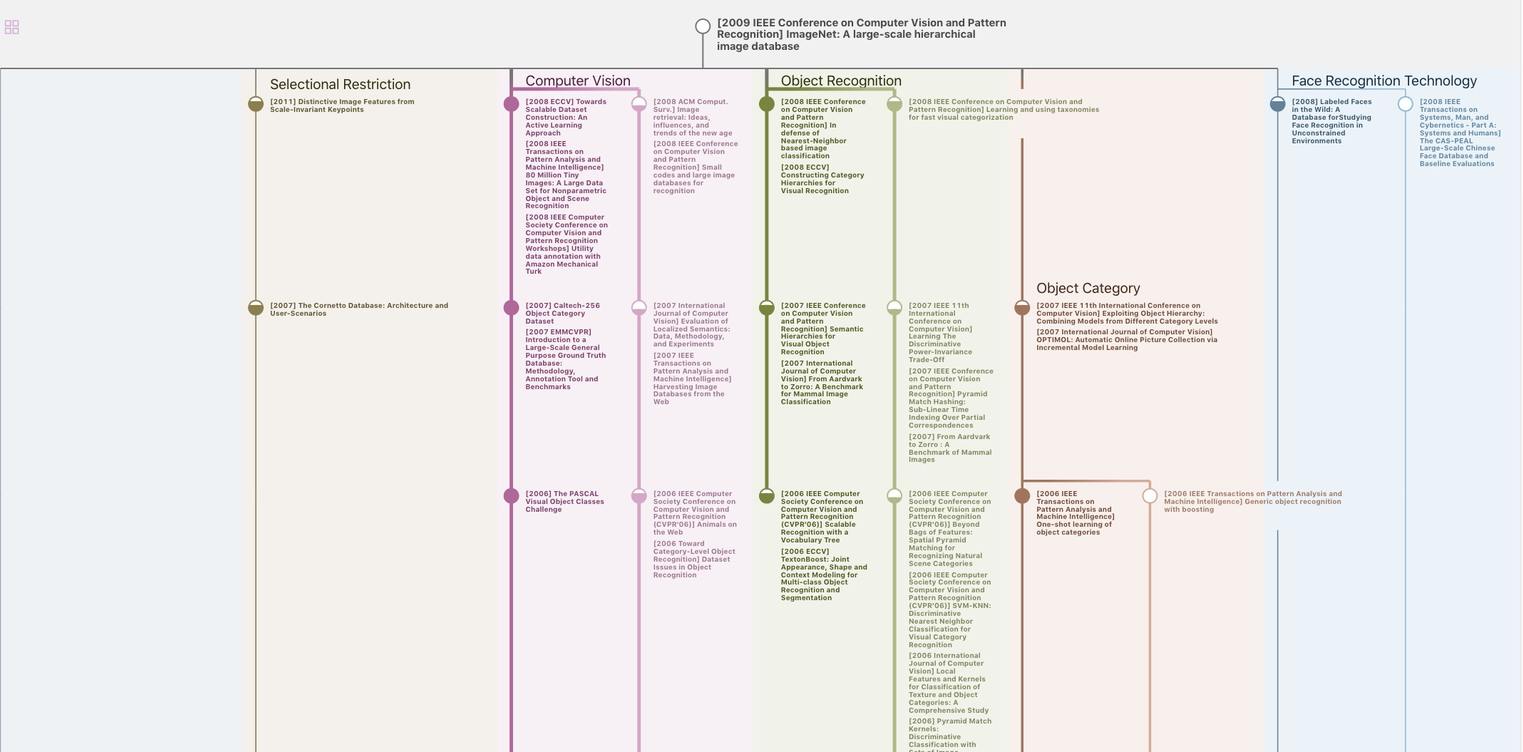
生成溯源树,研究论文发展脉络
Chat Paper
正在生成论文摘要