Flexible 3D Object Appearance Observation Based on Pose Regression and Active Motion
2022 IEEE 18th International Conference on Automation Science and Engineering (CASE)(2022)
摘要
3D object appearance inspection plays an important role in manufacturing industry. To observe clear images of different parts of a 3D object in a semi-structured scene, camera pose should be properly adjusted to several different viewpoints. In this paper, we propose a flexible appearance observation framework for 3D-shaped objects with 3-DoF pose (2D position and 1D angle) uncertainty. First, we propose 3-DoF Pose Regression Network (PR3Net) based on convolutional neural network (CNN), to estimate the 3-DoF pose of a target 3D object placed on a platform. Considering the data scarcity problem in practical application and the variety of object types, we utilize data synthesis to automatically generate training samples from only one annotated image sample, so that the pose learning can be conducted conveniently. Besides, a semi-supervised fine-tuning method is used to improve the generalization ability by leveraging plenty of unlabeled images. Second, the teachable active motion strategy is designed to enable the inspection robot to observe a 3D object from multiple viewpoints. The human user teaches the standard viewpoints once beforehand. The robot actively moves its camera multiple times according to both the predefined viewpoints and the regressed 3-DoF pose, so that the images of multiple parts of object are collected. The effectiveness of the proposed methods is validated by a series of experiments.
更多查看译文
关键词
3-DoF Pose Regression Network,PR3Net,target 3D object,object types,annotated image sample,pose learning,semisupervised fine-tuning method,teachable active motion strategy,flexible 3D object appearance observation,3D object appearance inspection,flexible appearance observation framework,3D-shaped objects
AI 理解论文
溯源树
样例
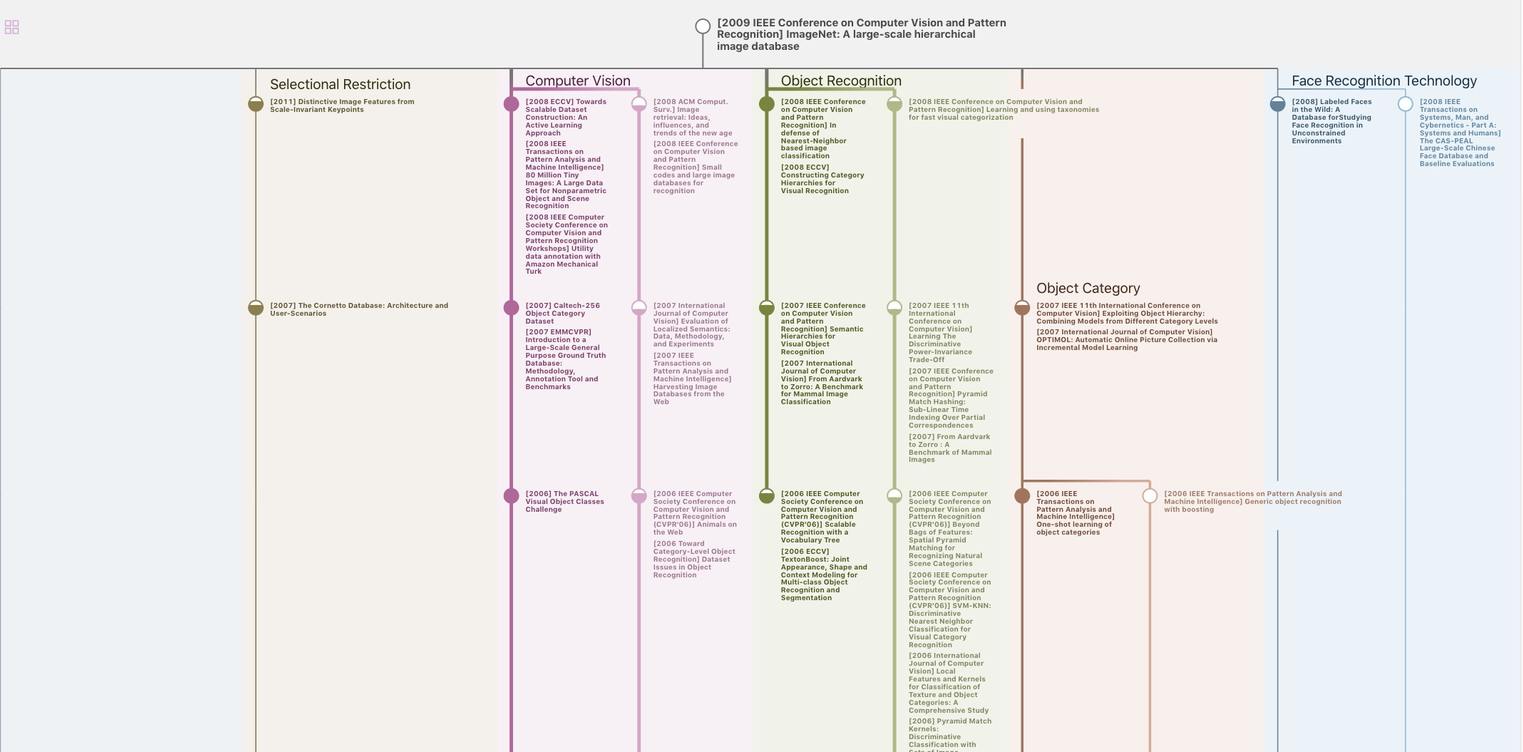
生成溯源树,研究论文发展脉络
Chat Paper
正在生成论文摘要