SingleDemoGrasp: Learning to Grasp From a Single Image Demonstration
2022 IEEE 18th International Conference on Automation Science and Engineering (CASE)(2022)
摘要
Learning-based grasping models typically require a large amount of training data and training time to generate an effective grasping model. Alternatively, small non-generic grasp models have been proposed that are tailored to specific objects by, for example, directly predicting the object’s location in 2/3D space, and determining suitable grasp poses by post processing. In both cases, data generation is a bottleneck, as this needs to be separately collected and annotated for each individual object and image. In this work, we tackle these issues and propose a grasping model that is developed in four main steps: 1. Visual object grasp demonstration, 2. Data augmentation, 3. Grasp detection model training and 4. Robot grasping action. Four different vision-based grasp models are evaluated with industrial and 3D printed objects, robot and standard gripper, in both simulation and real environments. The grasping model is implemented in the OpenDR toolkit at: https://github.com/opendr-eu/opendr/tree/master/projects/control/single_demo_grasp.
更多查看译文
关键词
Grasping,Deep Learning in Grasping and Manipulation,Perception for Grasping and Manipulation
AI 理解论文
溯源树
样例
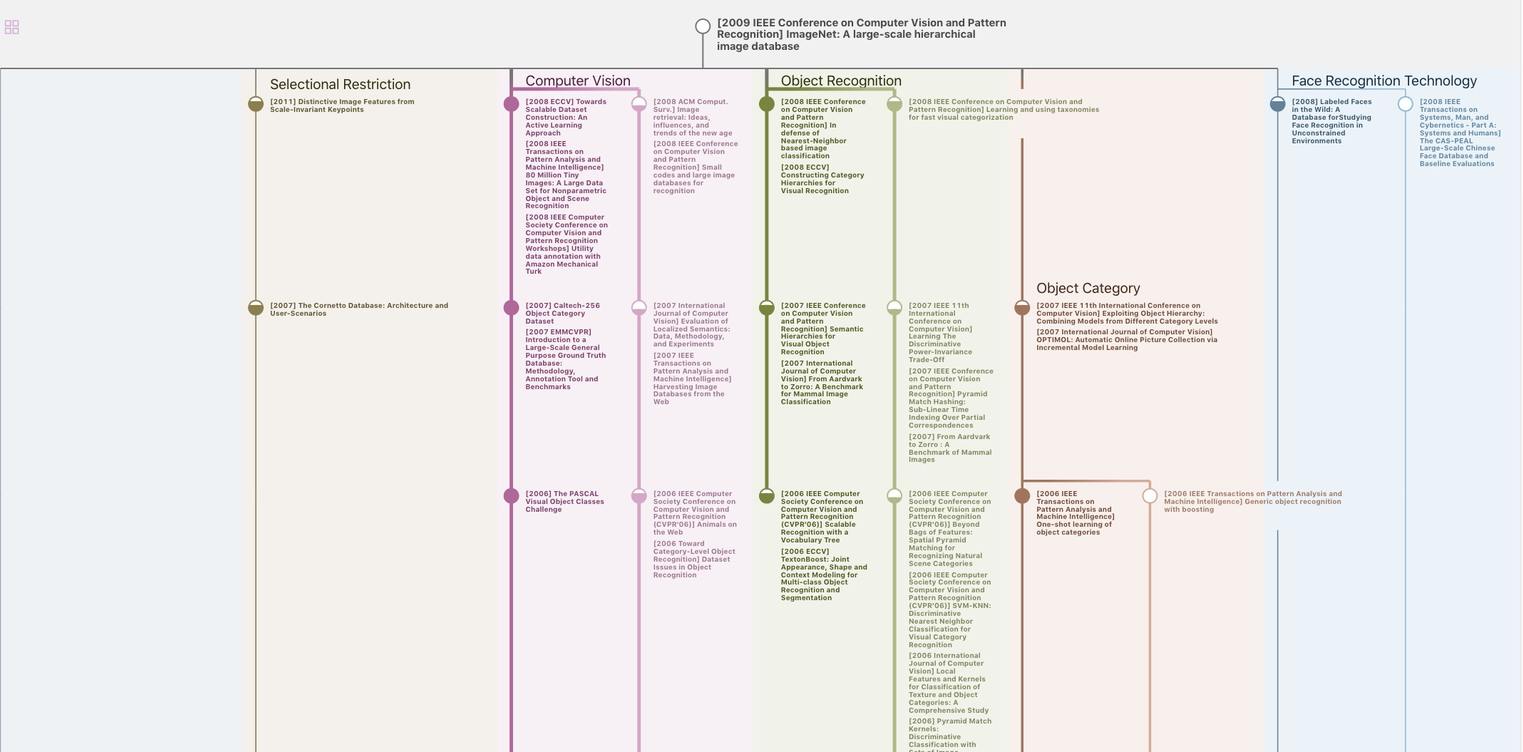
生成溯源树,研究论文发展脉络
Chat Paper
正在生成论文摘要