Avid: A Variational Inference Deliberation For Meta-Learning
2022 12th International Conference on Computer and Knowledge Engineering (ICCKE)(2022)
摘要
Meta-learning techniques enable quick learning of new tasks by using few samples with utilizing prior knowledge learned from previous tasks. Gradient-based models are widely used because of their simplicity and ability to solve a wide range of problems. However, they only succeed in solving tasks with a very similar structure since they adapt the model with a shared meta-parameter across all tasks. In recent years, some models have been proposed to enhance the gradient-based models to deal with task uncertainty and heterogeneity via sharing knowledge among similar tasks by using task clustering. Nevertheless, the high-dimensional parameter space of gradient-based models hinders them from achieving their full potential in low-data regimes. Bayesian meta-learning algorithms address this issue by learning a data-dependent latent generative representation of model parameters. Our proposed model bypasses the aforementioned limitations by leveraging Bayesian algorithms as well as clustering input tasks. The final analysis demonstrates the effectiveness of the proposed model for few-shot image classification problems.
更多查看译文
AI 理解论文
溯源树
样例
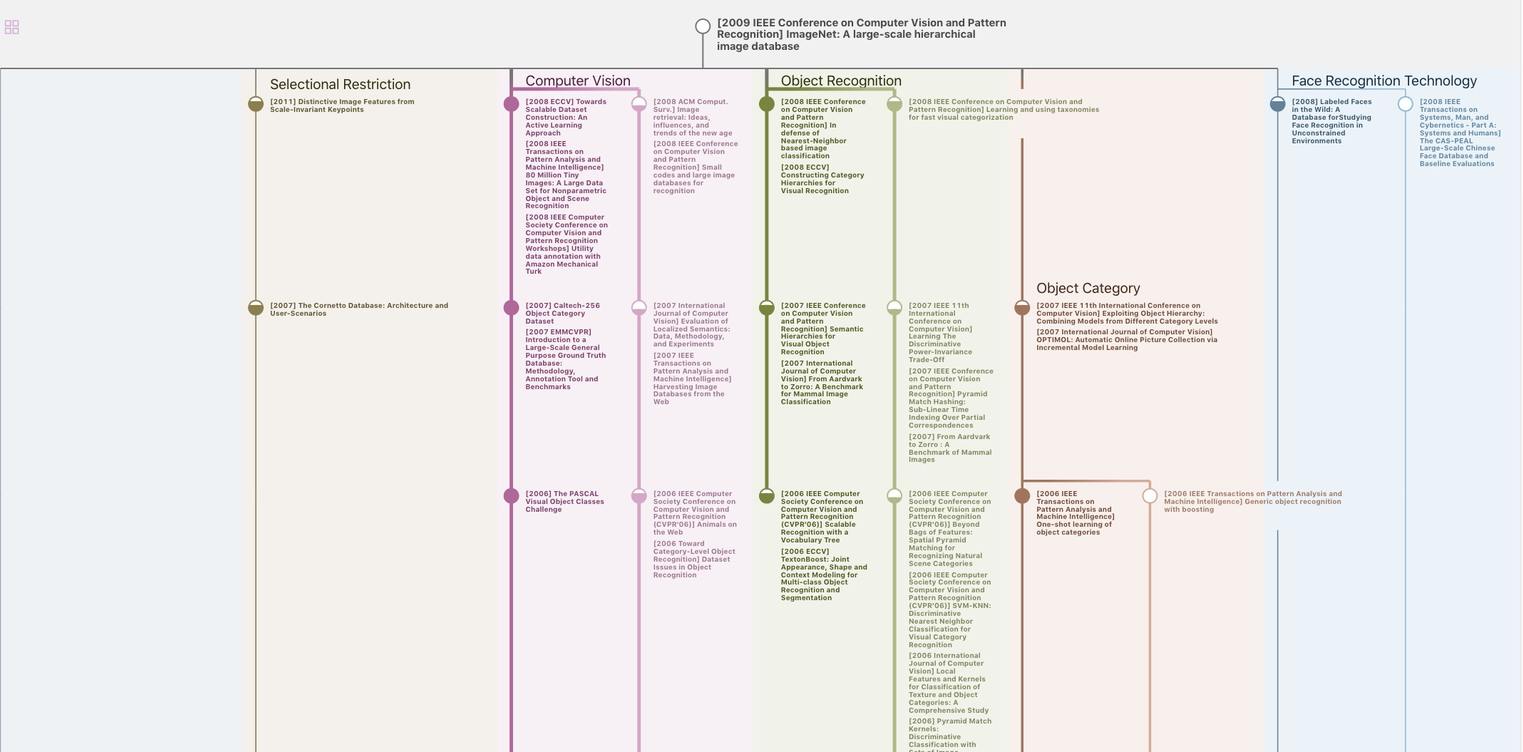
生成溯源树,研究论文发展脉络
Chat Paper
正在生成论文摘要