Zone-Based Federated Learning in Indoor Positioning
2022 12th International Conference on Computer and Knowledge Engineering (ICCKE)(2022)
摘要
With the advancement of artificial intelligence (AI), indoor positioning systems have been getting more attention in smart cities and smart homes for various purposes. However, protecting the privacy of users is one of the significant challenges in all smart environments. In very recent years, federated learning (FL) has been introduced as a solution for a privacy-aware indoor positioning system. However, there is still a risk of information leakage when transferring the model gradients between the clients and the central server in the federated learning process. In this paper, to avoid revealing the location information of users, we propose a zone-based federated learning method that employs FL to determine the zone of the users instead of their exact location. Therefore, even with the leakage of model gradients, the users’ exact location cannot be determined; thus, their privacy is preserved. We verified the suggested method utilizing two real datasets and compared the results with the non-FL mode. Also, the accuracy of the proposed method is evaluated in various number of communication rounds, number of clients, and number of data for each client. The results on both datasets indicate that while the proposed method preserves the privacy of clients, the accuracy of that is very close to the accuracy of the non-FL mode.
更多查看译文
关键词
Federated learning,Indoor positioning,Privacy-preserving,Federated positioning
AI 理解论文
溯源树
样例
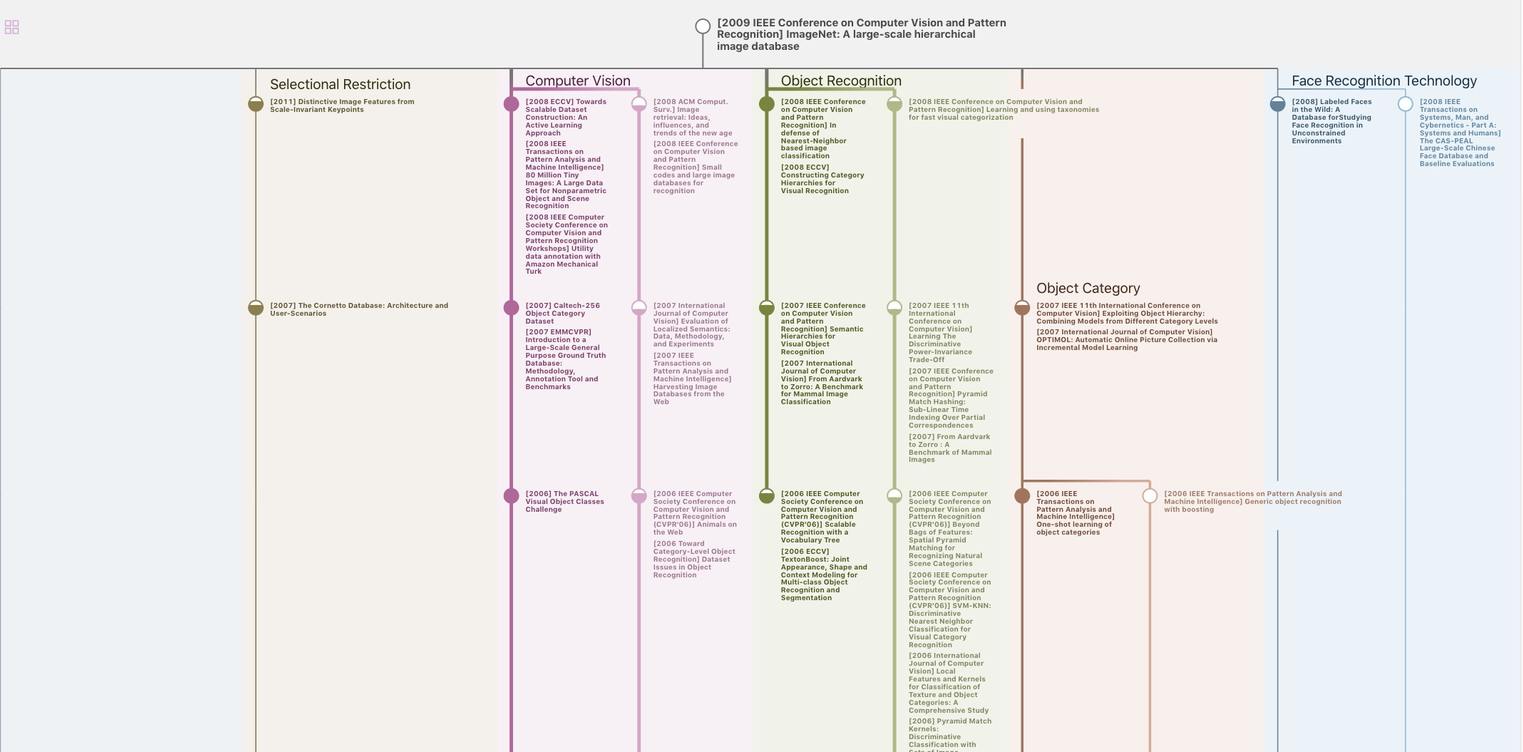
生成溯源树,研究论文发展脉络
Chat Paper
正在生成论文摘要