TD3-based Algorithm for Node Selection on Multi-tier Federated Learning
2022 International Conference on Computing, Communication, Perception and Quantum Technology (CCPQT)(2022)
摘要
Federated learning enables distributed devices to conduct cooperative training models while protecting data privacy, so it is widely promoted in big data scenario and the scope of the Internet of Things. Federated learning in multi-tier computing can integrate the resources of the device-edge-fog-cloud layer to interact and cooperate. For example, in addition to offloading training locally, the tasks of the device layer can also be uploaded to the edge layer or the fog layer for training, while the global aggregation node can be selected at the edge or fog or cloud. However, due to the uncertainty of network bandwidth, computing resources and terminal training tasks at each layer, it brings challenges to resource allocation and task offloading under federated learning in multi-tier computing. Therefore, we propose a TD3-based algorithm which aims to solve how to select training nodes and aggregation nodes during joint training on multi-tier federated learning to minimize the average task delay. Numerical experiments show that our method has better performance in terms of energy consumption and delay compared with edge federated learning and traditional federated learning.
更多查看译文
关键词
component,Deep reinforcement learning,Task scheduling,Federated learning,Multi-tier computing
AI 理解论文
溯源树
样例
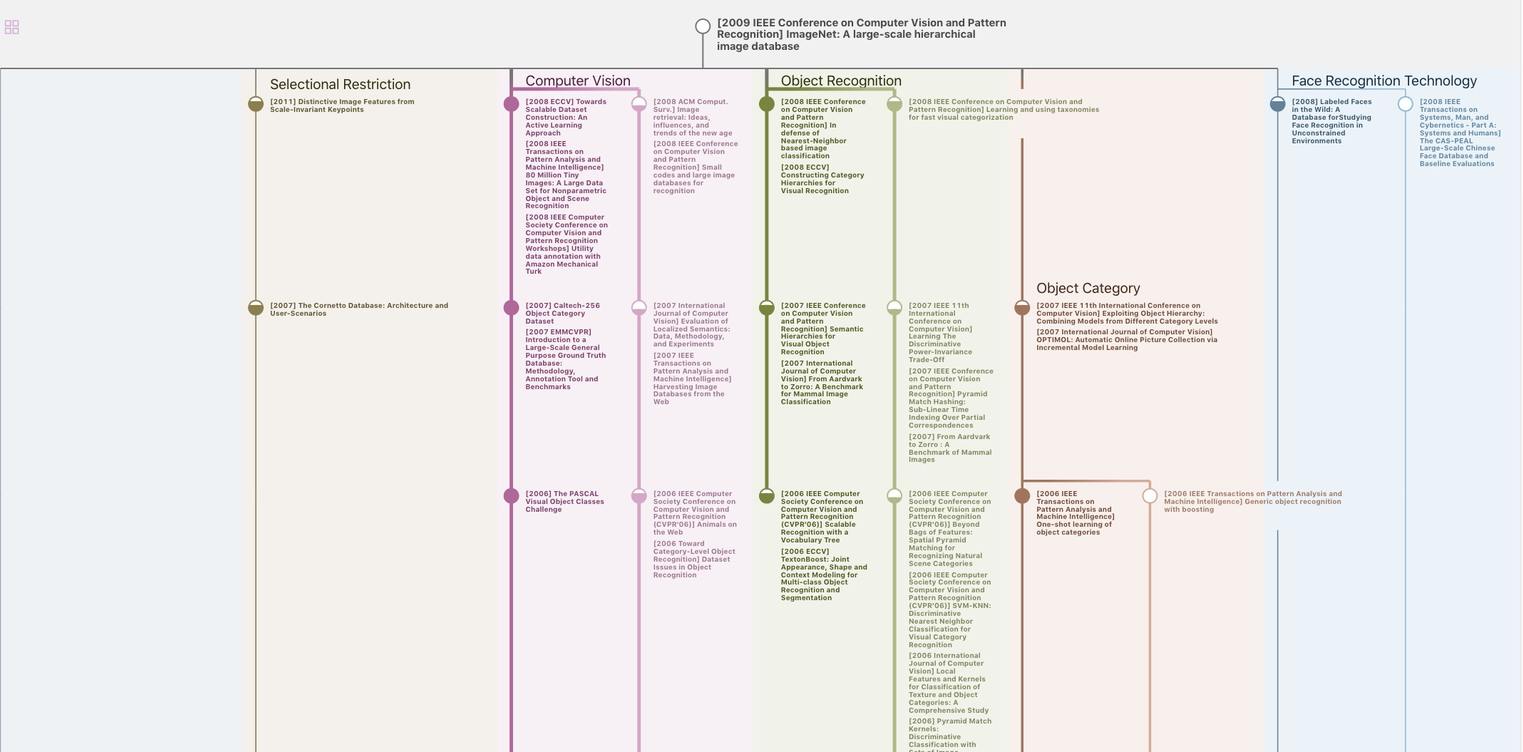
生成溯源树,研究论文发展脉络
Chat Paper
正在生成论文摘要