Cost Aggregation with Transformers for Sparse Correspondence
2022 IEEE International Conference on Consumer Electronics-Asia (ICCE-Asia)(2022)
Abstract
In this work, we introduce a novel network, namely SuperCATs, which aims to find a correspondence field between visually similar images. SuperCATs stands on the shoulder of the recently proposed matching networks, SuperGlue and CATs, taking the merits of both for constructing an integrative framework. Specifically, given keypoints and corresponding descriptors, we first apply attentional aggregation consisting of self- and cross-graph neural network to obtain feature descriptors. Subsequently, we construct a cost volume using the descriptors, which then undergoes a tranformer aggregator for cost aggregation. With this approach, we manage to replace the hand-crafted module based on solving an optimal transport problem initially included in SuperGlue with a transformer well known for its global receptive fields, making our approach more robust to severe deformations. We conduct experiments to demonstrate the effectiveness of the proposed method, and show that the proposed model is on par with SuperGlue for both indoor and outdoor scenes.
MoreTranslated text
Key words
Sparse Correspondence,Aggregation,Transformer,Neural Networks
AI Read Science
Must-Reading Tree
Example
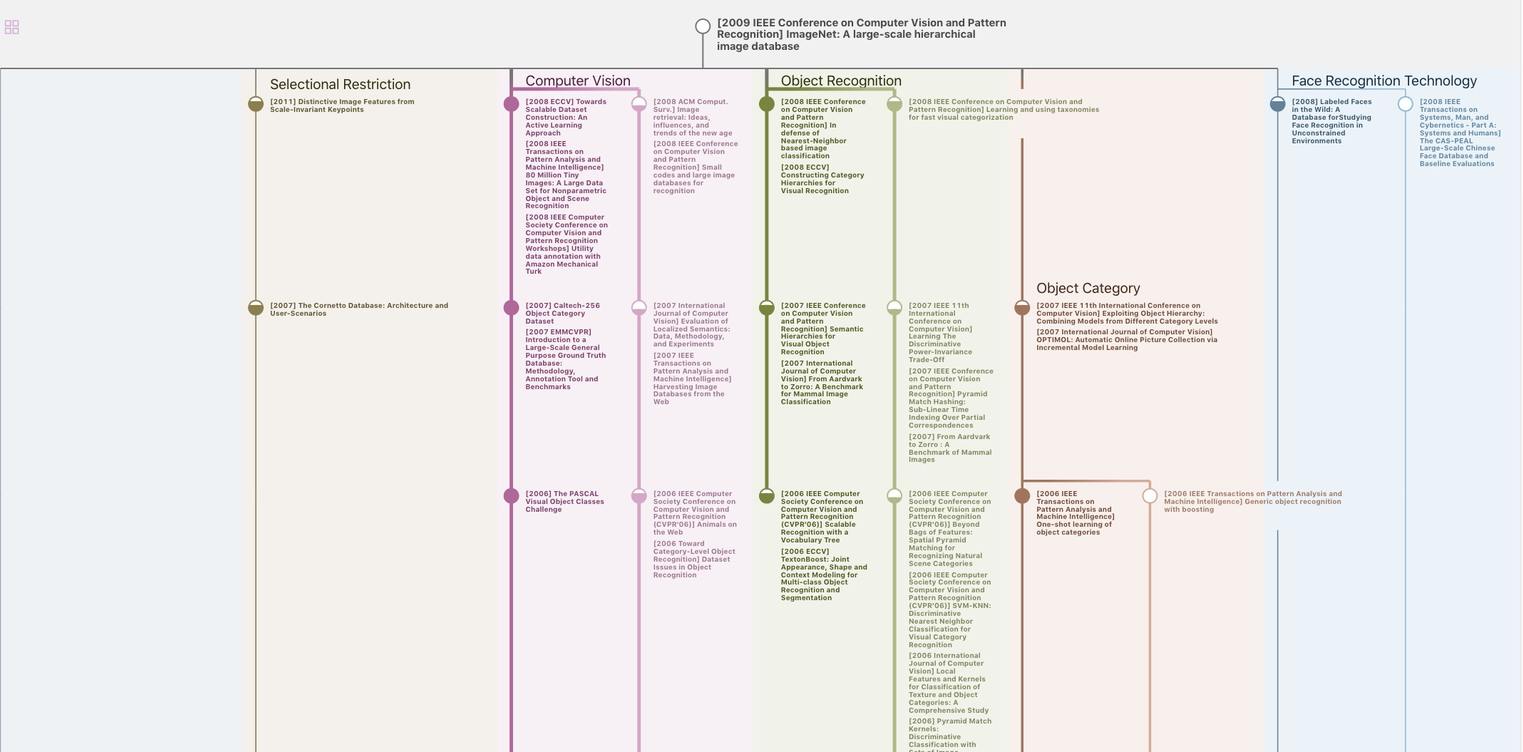
Generate MRT to find the research sequence of this paper
Chat Paper
Summary is being generated by the instructions you defined