A Fine-grained Classification Method of Thangka Image Based on SENet
2022 International Conference on Cyberworlds (CW)(2022)
摘要
"Thangka", is a word in the Tibetan language that refers to a kind of scroll painting mounted on silk. Thangka art, which is a very cherished and intangible cultural heritage, has a long history and distinctive characteristics. As an important prerequisite for digital protection, the research on how to classify the Thangka images quickly and accurately has become a problem of concern to scholars from in various fields. Due to the high similarity and complex structure of the Thangka image, manual classification needs to consume a large number of human resources with sufficient knowledge reserves. To improve the efficiency and accuracy of Thangka classification, researchers began to focus on computer-based machine learning and deep learning technology to complete the Thangka image classification task. Due to the complexity of the Thangka image and the lack of training samples, the existing classification methods cannot well complete the Thangka classification task. To solve the above problems, this paper proposes a fine-grained classification method Tk-SENet for Thangka images based on SENet. This method introduces the dual mechanism of spatial attention and channel attention and uses different sizes of convolution kernels to perform convolution operations in images and gives different weights according to the importance of channels and regions, which improves the classification efficiency. The pooling method in the squeeze operation and the activation function in the excitation operation are optimized to improve the classification accuracy. In the training process, the unique training method of the Thangka image is used to prepare for the excellent completion of the fine-grained classification task of the Thangka image. The experimental results show that the improved network model improves the classification accuracy by 3.6658% compared with SENet. Compared with AlexNet, ZFNet, VGG16, VGG19, ResNet-152 , DenseNet and SKNet, the accuracy increases by 6.7747%, 6.2804%, 6.6528%, 5.2586%, 6.0404% , 5.6962% and 2.9241% respectively. At the same time, compared with the existing classification methods of Thangka images, the proposed method can not only accurately identify the categories of Thangka statues, but also identify the production process types of Thangka images. Therefore, this method is more suitable for the fine-grained classification of Thangka images and provides strong technical support for the digital protection of intangible cultural heritage.
更多查看译文
关键词
Thangka image,Intangible cultural heritage,Fine-grained classification,SENet,Training strategy
AI 理解论文
溯源树
样例
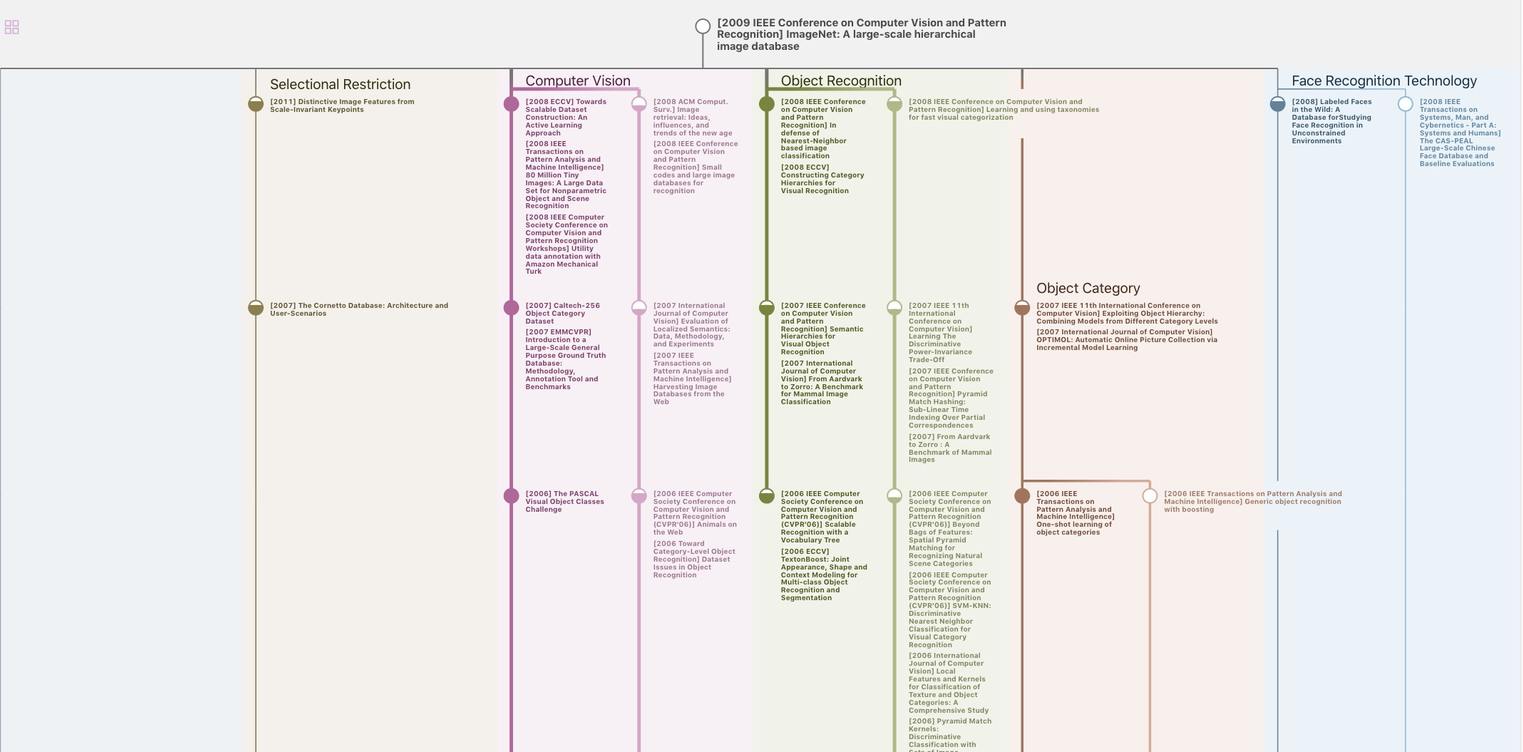
生成溯源树,研究论文发展脉络
Chat Paper
正在生成论文摘要