A Small-sample Intelligent Fault Diagnosis Method Based on Deep Transfer Learning
2022 5th International Conference on Data Science and Information Technology (DSIT)(2022)
摘要
In real industrial environments, it is difficult to obtain a large amount of monitoring data of the equipment to be diagnosed under specific working conditions. At the same time, the distribution of training and test data often differs greatly due to the variation of working conditions and operation modes. Directly using small sample data for training will lead to the degradation of model accuracy, while using different working conditions data for joint training may lead to the degradation of diagnostic accuracy due to domain differences. Therefore, how to ensure the accuracy of cross-domain fault diagnosis under small sample conditions becomes a challenge that must be faced. In this paper, an end-to-end deep transfer learning method based on convolutional neural networks (CNN) is proposed to solve the cross-domain diagnosis problem. Specifically, the method uses CNN to extract the source domain data features and build a pre-trained model; subsequently, the model is fine-tuned using a small amount of data from the target domain according to the transfer learning strategy to obtain the final intelligent diagnostic model. In addition, experiments using a publicly available dataset demonstrate the usability and effectiveness of the proposed method in this study.
更多查看译文
关键词
fault diagnosis,transfer learning,domain adaptation,small sample learning,deep learning
AI 理解论文
溯源树
样例
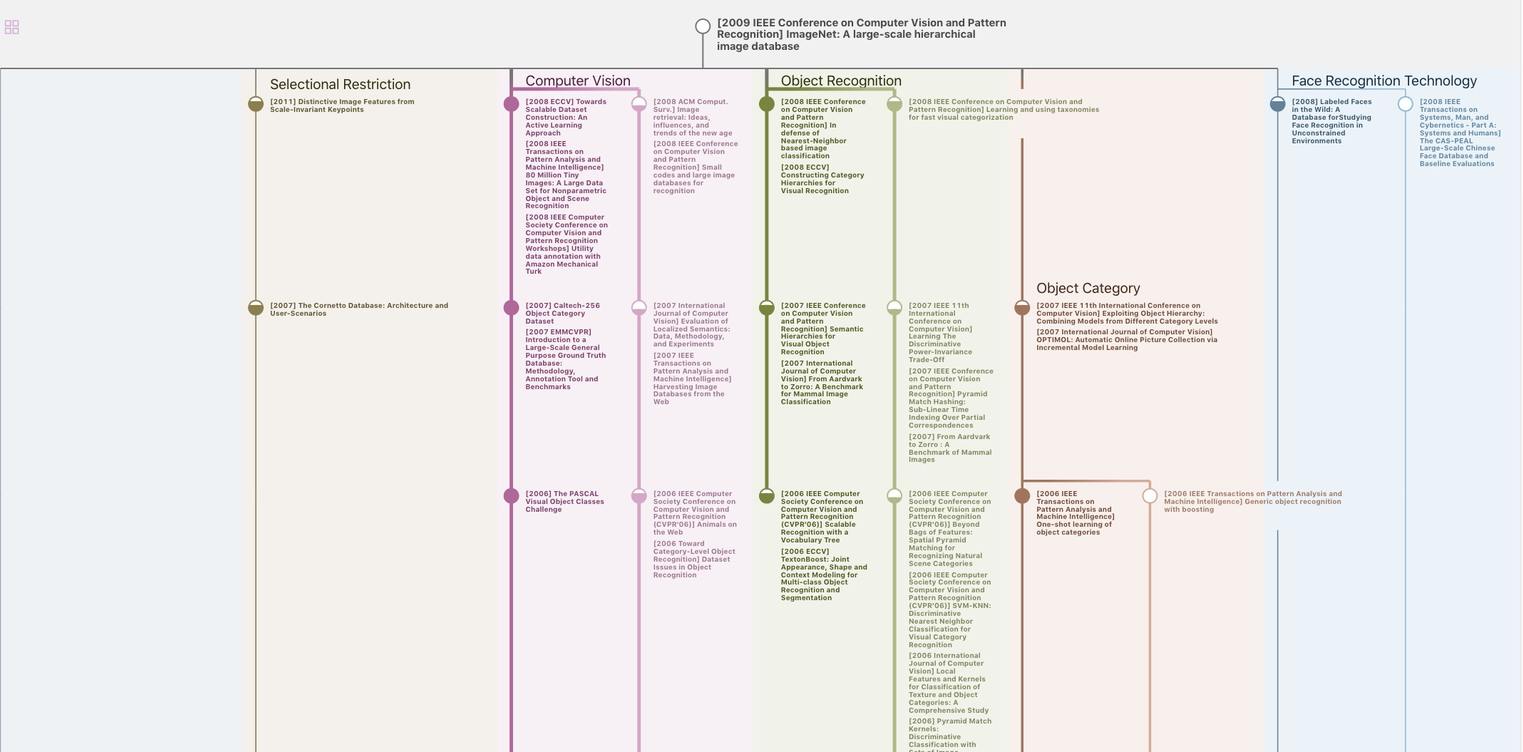
生成溯源树,研究论文发展脉络
Chat Paper
正在生成论文摘要