The Correlation between Training Set Perturbations and Robustness for CNN Models
2022 9th International Conference on Dependable Systems and Their Applications (DSA)(2022)
摘要
Convolutional Neural Network (CNN) models perform well in image processing and are increasingly used in face recognition, self-driving cars, etc. However, CNN models are susceptible to perturbation and thus fail. Adding perturbation samples to the training sets is a method to improve the perturbation resistance of CNN models. In this paper, we give the methods including dataset construction, model retraining, and robustness metrics. The empirical study on the correlation between Project Gradient Descent (PGD) adversarial training and CNN model robustness is carried out in perturbation degree, proportion, and sample feature in training sets. The results show that the trend of CNN model robustness is related to the network architecture and it changes with the perturbation degree and proportion, and that perturbing images with one feature in the training set can improve the ability of CNN models to recognize images with that feature, and training for the scenario to which the CNN model is applied can improve the robustness of the model.
更多查看译文
关键词
convolutional neural network,robustness,training set perturbations,image features
AI 理解论文
溯源树
样例
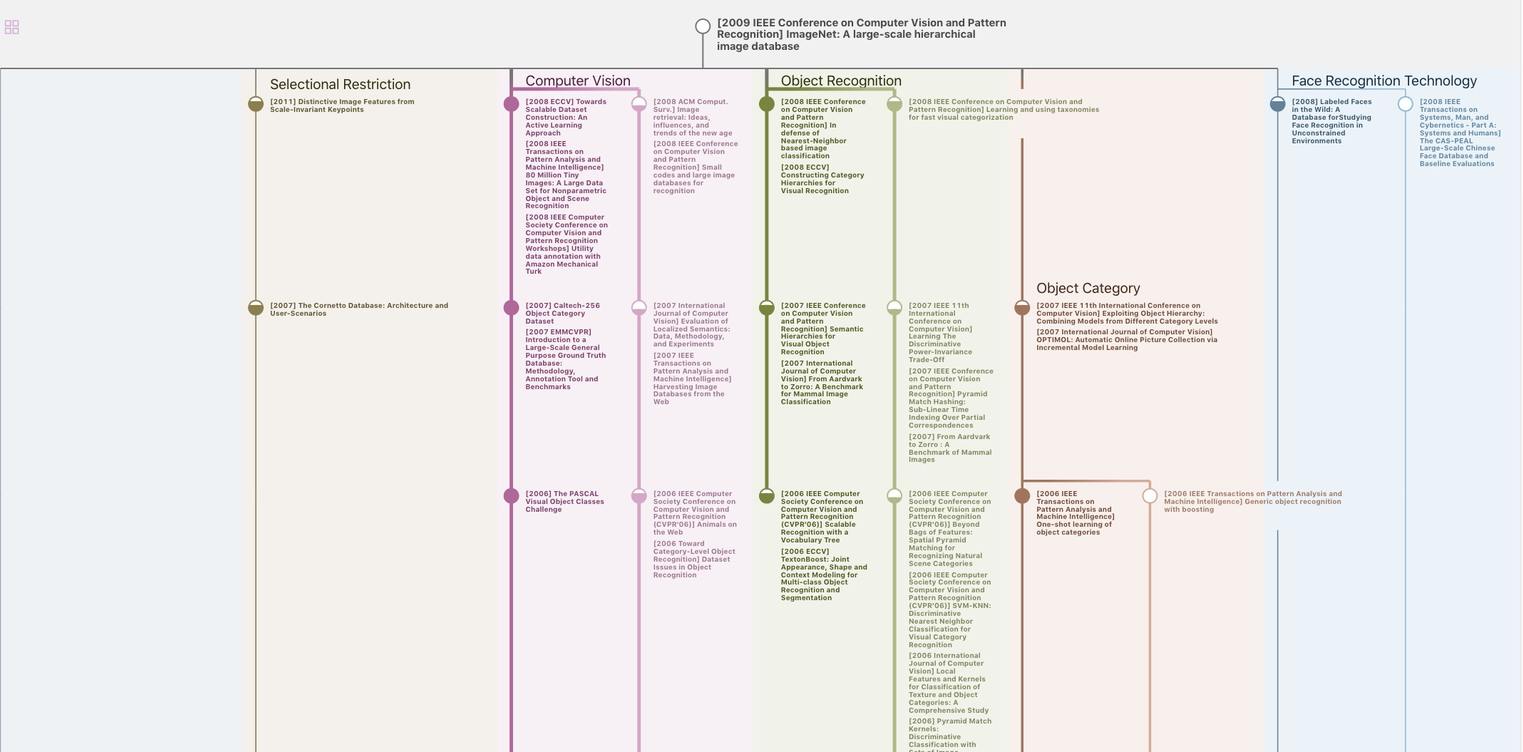
生成溯源树,研究论文发展脉络
Chat Paper
正在生成论文摘要