Theoretical Analysis of Value-Iteration-Based Q-Learning with Approximation Errors
2022 12th International Conference on Information Science and Technology (ICIST)(2022)
摘要
In this paper, the value-iteration-based Q-Iearning algorithm with approximation errors is analyzed theoretically. First, based on an upper bound of the approximation errors caused by the Q-function approximator, we get the lower and upper bound functions of the iterative Q-function, which proves that the limit of the approximate Q-function sequence is bounded. Then, we develop a stability condition for the termination of the iterative algorithm, for ensuring that the current control policy derived from the resulting approximate Q-function is stabilizing. Also, we establish an upper bound function of the approximation errors, which is caused by the policy function approximator, to guarantee that the approximate control policy is stabilizing. Finally, the numerical results verifies the theoretical results with a simulation example.
更多查看译文
关键词
Adaptive dynamic programming,Q-Iearning,value iteration,asymptotic stability
AI 理解论文
溯源树
样例
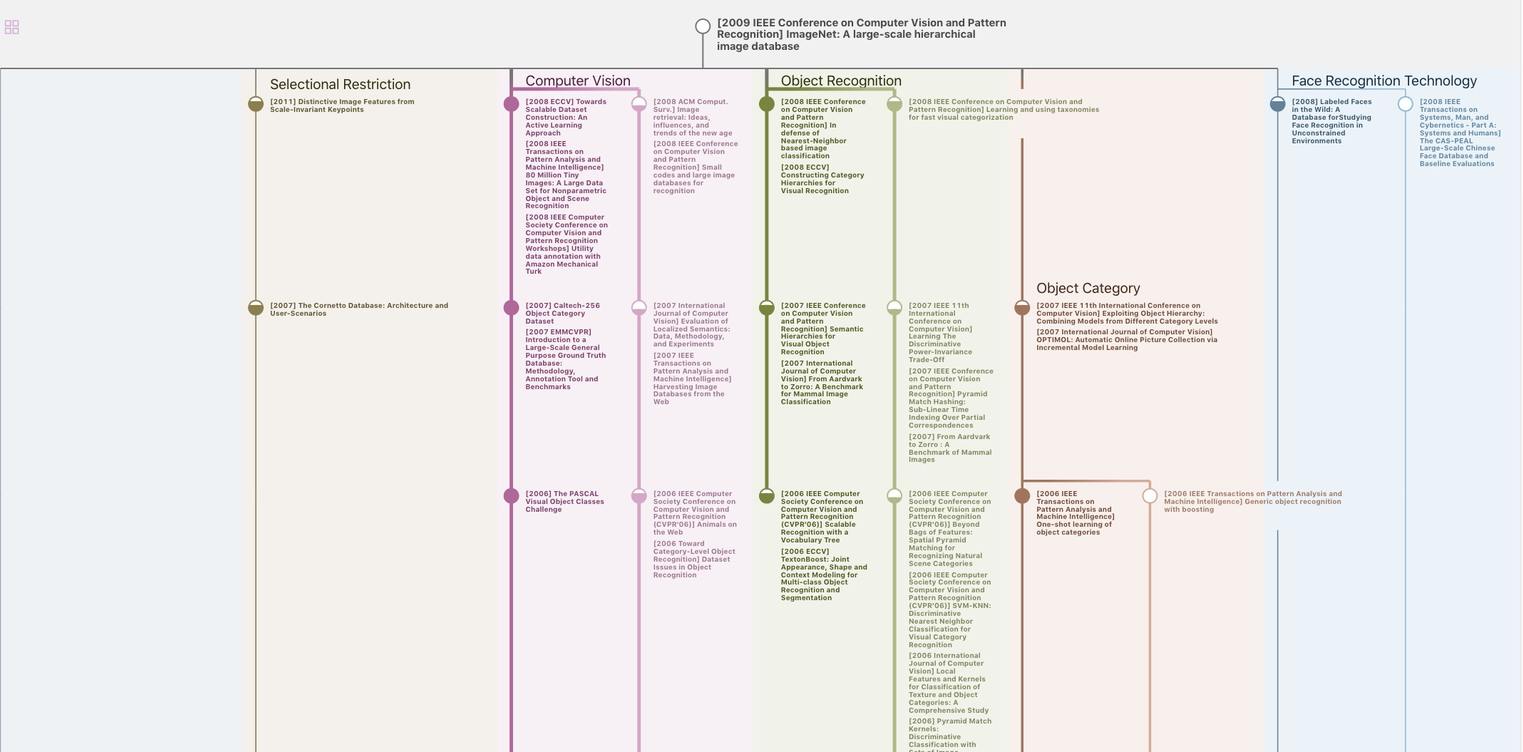
生成溯源树,研究论文发展脉络
Chat Paper
正在生成论文摘要