How Generalizable and Interpretable are Speech-Based COVID-19 Detection Systems?: A Comparative Analysis and New System Proposal
2022 IEEE-EMBS International Conference on Biomedical and Health Informatics (BHI)(2022)
摘要
Recent work has shown the potential of using speech signals for remote detection of coronavirus disease 2019 (COVID-19). Due to the limited amount of available data, however, existing systems have been typically evaluated within the same dataset. Hence, it is not clear whether systems can be generalized to unseen speech signals and if they indeed capture COVID-19 acoustic biomarkers or only dataset-specific nuances. In this paper, we start by evaluating the robustness of systems proposed in the literature, including two based on hand-crafted features and two on deep neural network architectures. In particular, these systems are tested across two international COVID-19 detection challenge datasets (COMPARE and DICOVA2). Experiments show that the performance of the explored systems degraded to chance levels when tested on unseen data, especially those based on deep neural networks. To increase the generalizability of existing systems, we propose a new set of acoustic biomarkers based on speech modulation spectrograms. The new biomarkers, when used to train a simple linear classifier, showed substantial improvements in cross-dataset testing performance. Further interpretation of the biomarkers provides a better understanding of the acoustic properties of COVID-19 speech. The generalizability and inter-pretability of the selected biomarkers allow for the development of a more reliable and lower-cost COVID-19 detection system.
更多查看译文
关键词
COVID-19 detection,speech analysis,generalizability,modulation spectrogram,acoustic properties
AI 理解论文
溯源树
样例
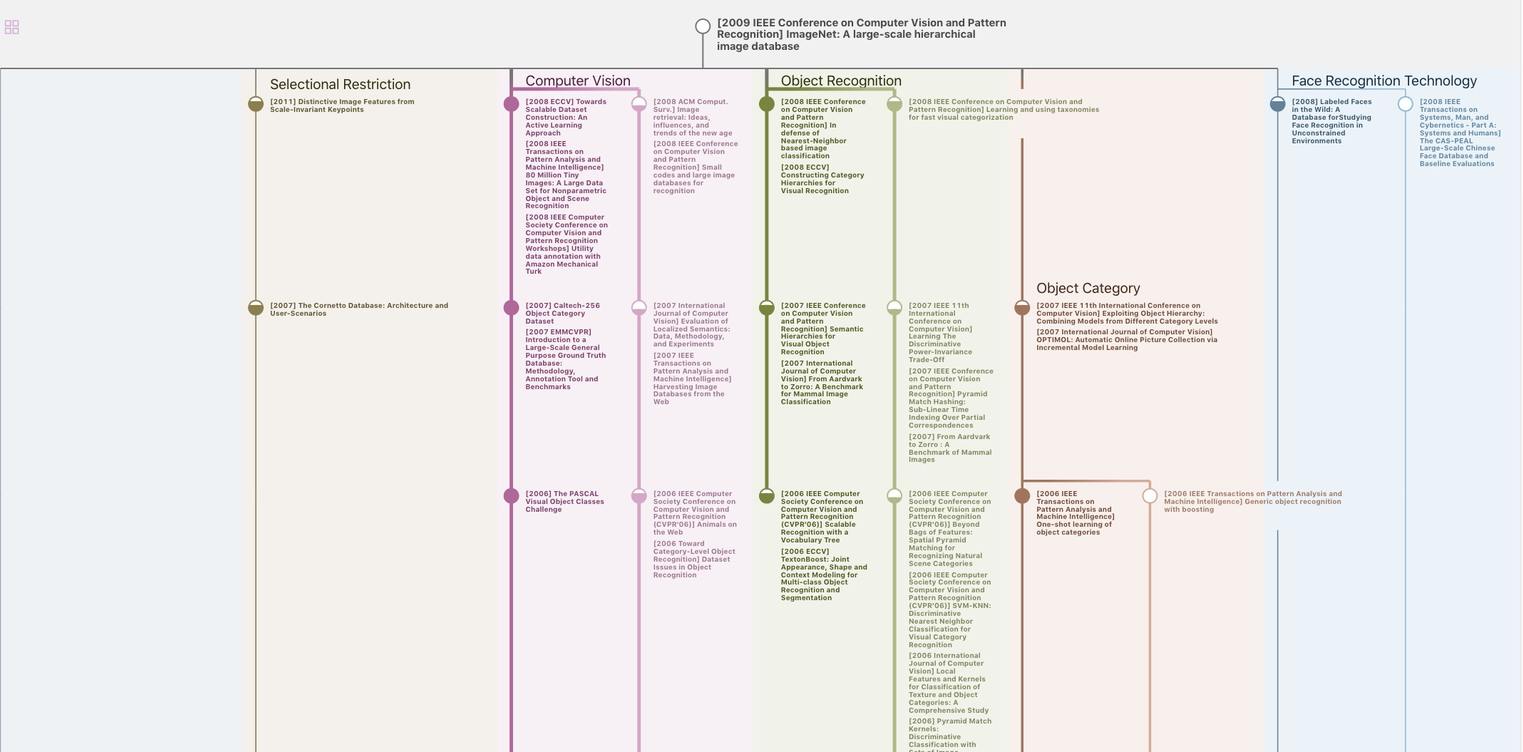
生成溯源树,研究论文发展脉络
Chat Paper
正在生成论文摘要