FBW-SNN: A Fully Binarized Weights-Spiking Neural Networks for Edge-AI Applications
2022 International Conference on IC Design and Technology (ICICDT)(2022)
摘要
This study proposes a Fully Binarized Weight Spiking Neuron Network (FBW-SNN). The core network is built based on XNOR arrays with binary weights where the weights of the first and the last layers are represented by a stochastic binary stream (stochastic numbers). We also introduced an algorithm that combines straight-through and surrogate gradients to train FBW-SNN. The evaluation results on the CIFAR-10 dataset show that the proposed FBW-SNN could achieve an accuracy of 82.68% with only 14-time steps, which is comparable to the accuracy of the Binarized SNN (with real weights at the first and the last layer) and conventional SNNs. By fully binarized, the proposed network model could be a promising candidate for Edge-AI applications implemented on low-power and resource-constrained devices.
更多查看译文
关键词
Binary Spiking Neural Network,Neuromorphic Computing,In-memory Computing,Edge-AI
AI 理解论文
溯源树
样例
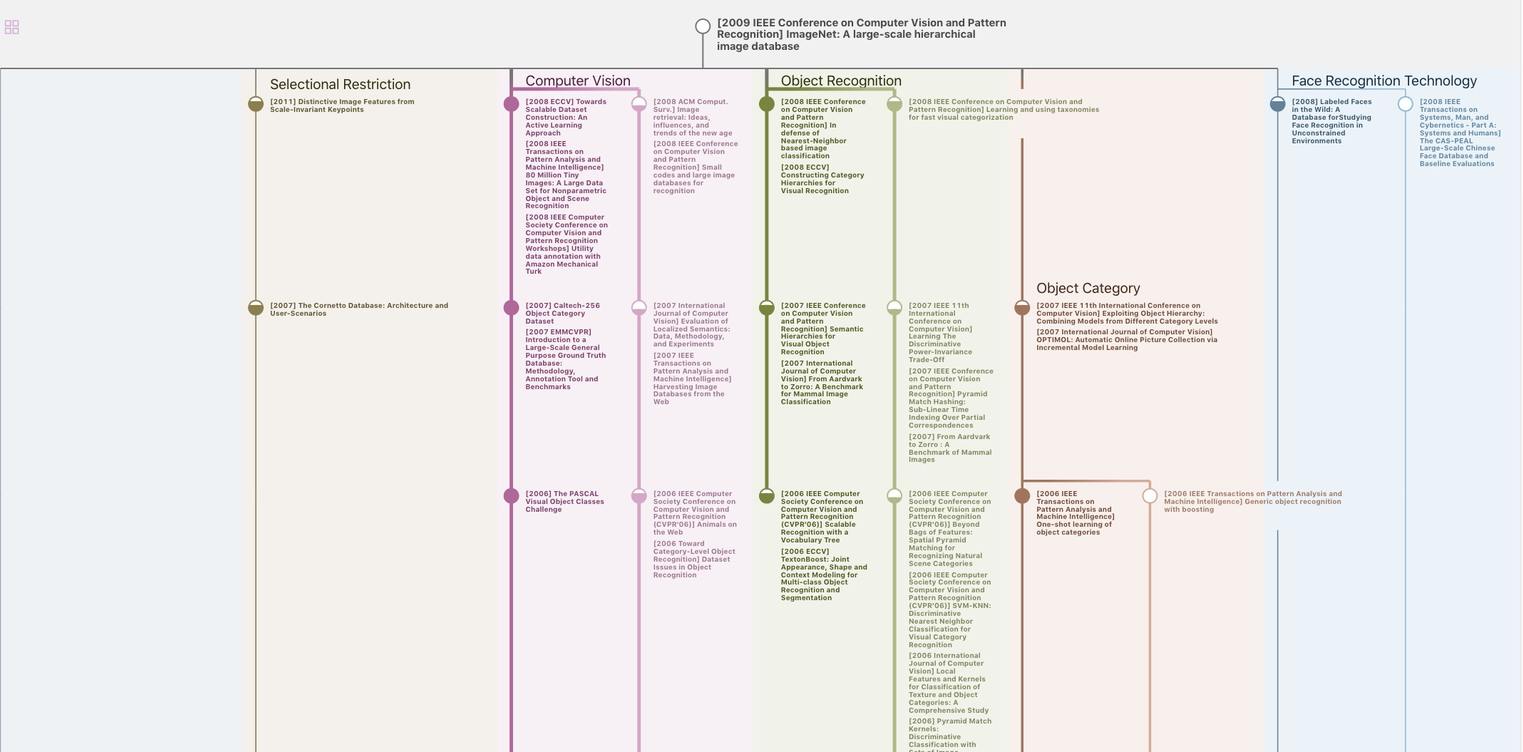
生成溯源树,研究论文发展脉络
Chat Paper
正在生成论文摘要