Data-Driven Human-Like Path Planning for Autonomous Driving Based on Imitation Learning
2022 5th International Conference on Intelligent Autonomous Systems (ICoIAS)(2022)
摘要
In autonomous driving systems, path planning algorithms play a crucial role in ensuring vehicle safety, driving efficiency, and passenger comfort. The traditional path planning algorithms cannot meet the real-time and passenger comfort requirements of autonomous driving, an alternative is imitation learning, a data-driven approach that mimics expert agents' driving behavior and leverages recent advances in supervised learning. We present a learning-based planner that aims to robustly drive a vehicle by mimicking human drivers' driving behavior. First, we leverage a mid-to-mid approach that allows us to reduce sample complexity. Furthermore, we introduce task losses that penalize undesirable behaviors, which is done by introducing a differentiable rasterizer that directly converts the waypoints output by the network into images. Most importantly, we add the state information of ego vehicle at the historical time to the original LSTM network input which improves the completion rate of scene tasks and the comfort of passengers. Finally, we experiment on the nuPlan dataset to verify the effectiveness of our method.
更多查看译文
关键词
imitation learning,autonomous driving,path planning,data-driven
AI 理解论文
溯源树
样例
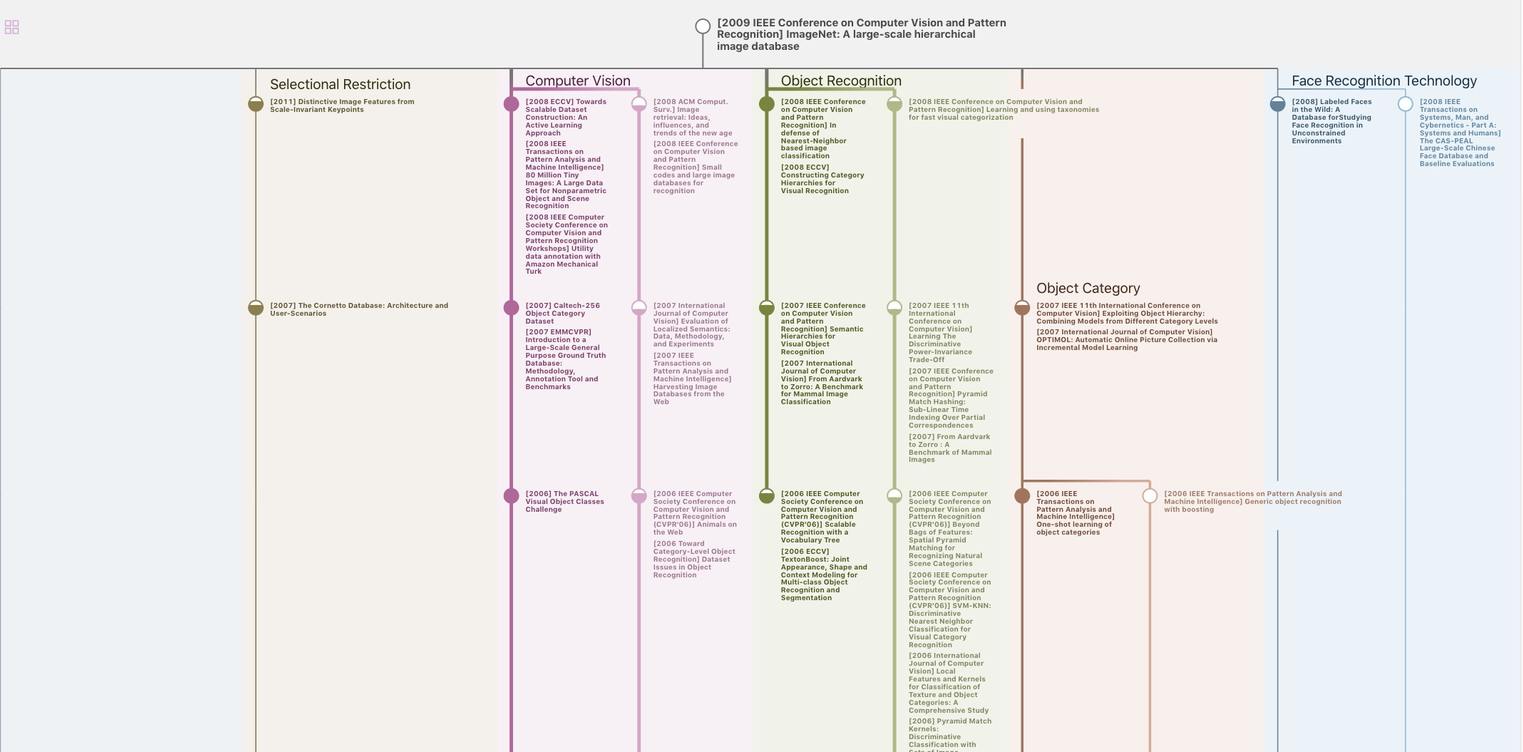
生成溯源树,研究论文发展脉络
Chat Paper
正在生成论文摘要