Improving the Classification Effectiveness of Network Intrusion Detection Using Ensemble Machine Learning Techniques and Deep Neural Networks
2022 International Conference on Intelligent Data Science Technologies and Applications (IDSTA)(2022)
摘要
Sophisticated cyber-attacks and ever-evolving threats have made securing networks highly complex due to the advent of Big data and Connected systems, and inaccuracy and incompetency of current Network Intrusion Detection Systems (NIDS). This poses a need for better network intrusion detection models to enhance network security and secure communication channels in the future. Over the years, machine learning and deep learning models have proven to be effective in detecting network intrusion and classification of attacks on networks. In this paper, we present our proposed NIDS based on machine learning and deep learning techniques to enhance the performance of current network intrusion detection systems. Decision tree, ensemble machine learning techniques like Random Forest and XGBoost, and Deep Neural Networks (DNN) have been used on the modern substitutes of the benchmark KDD CUP 99 dataset, the NSL KDD, and the UNSW NB-15. We apply unique feature selection methods and achieve competitive results. For Binary Classification, the results show that our models achieve high accuracies of more than 99.25% for the NSL KDD dataset and above 93% for UNSW NB15 dataset. For Multiclass Classification, our models achieve accuracies of more than 97.70% for NSL KDD and above S2.50% for the UNSW NB15 dataset.
更多查看译文
关键词
Network Intrusion Detection,cyber-attacks,Ensemble Machine Learning,Deep Neural Networks,Classification Effectiveness
AI 理解论文
溯源树
样例
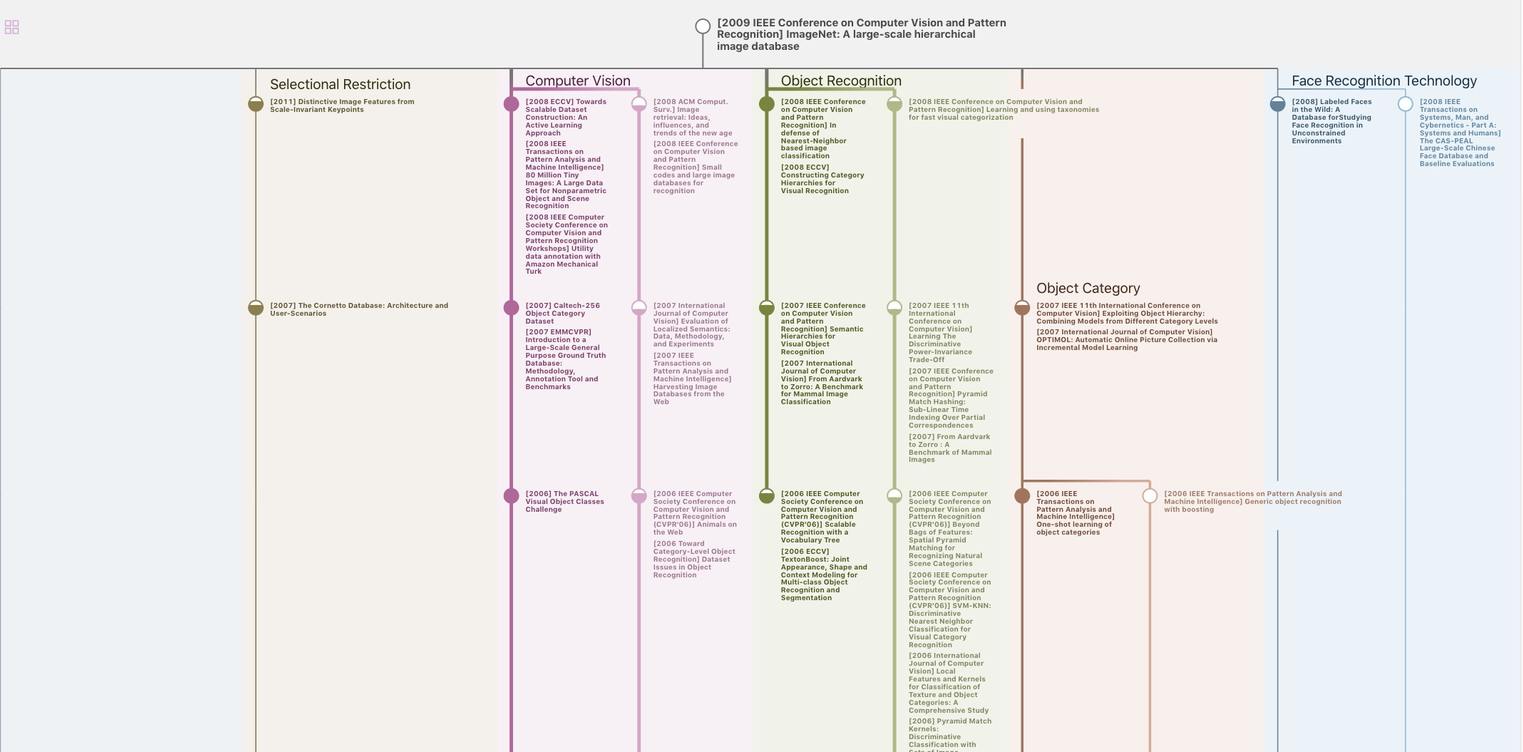
生成溯源树,研究论文发展脉络
Chat Paper
正在生成论文摘要