Semi-Supervised Image-to-Image Translation for Lane Detection in Rain
2022 IEEE 25th International Conference on Intelligent Transportation Systems (ITSC)(2022)
摘要
Impressive progress has recently been made in deep learning based lane detection for the autonomous vehicle domain using an in-car camera. However, relatively little attention was paid to lane detection under bad weather conditions. The general difficulty stems from the water on the road or raindrops remaining on the windscreen and hampering lane detectability. In this paper, we propose a lane enhancement approach to improve lane detection accuracy under rain. We formulate image enhancement as an image-to-image translation problem, and devise semi-supervised techniques to efficiently learn from an image set containing images from source domain (rain images) and target domain (clear images). Our semi-supervision approach differs from the conventional unsupervised image-to-image translation, in that a small amount of labelled rain images are added to the target domain in order to guide the translation to focus on enhancing the lanes while preserving the background. Specifically, we first compute the road regions in an image using vanishing points from camera intrinsic matrix. We then define a loss function using the road regions as constrains, in order to enforce lane-aware image generation. As a result, new rain images are generated by highlighting the lanes explicitly in thick bright lines. Our empirical results show that using only a few labelled images, our proposed semi-supervised learning is able to enhance lanes efficiently and improving lane detection significantly.
更多查看译文
关键词
road regions,lane-aware image generation,labelled images,semisupervised learning,semisupervised image-to-image translation,deep learning based lane detection,autonomous vehicle domain,windscreen,hampering lane detectability,lane enhancement approach,lane detection accuracy,image enhancement,image-to-image translation problem,semisupervised techniques,image set,source domain,target domain,clear images,semisupervision approach differs,labelled rain images
AI 理解论文
溯源树
样例
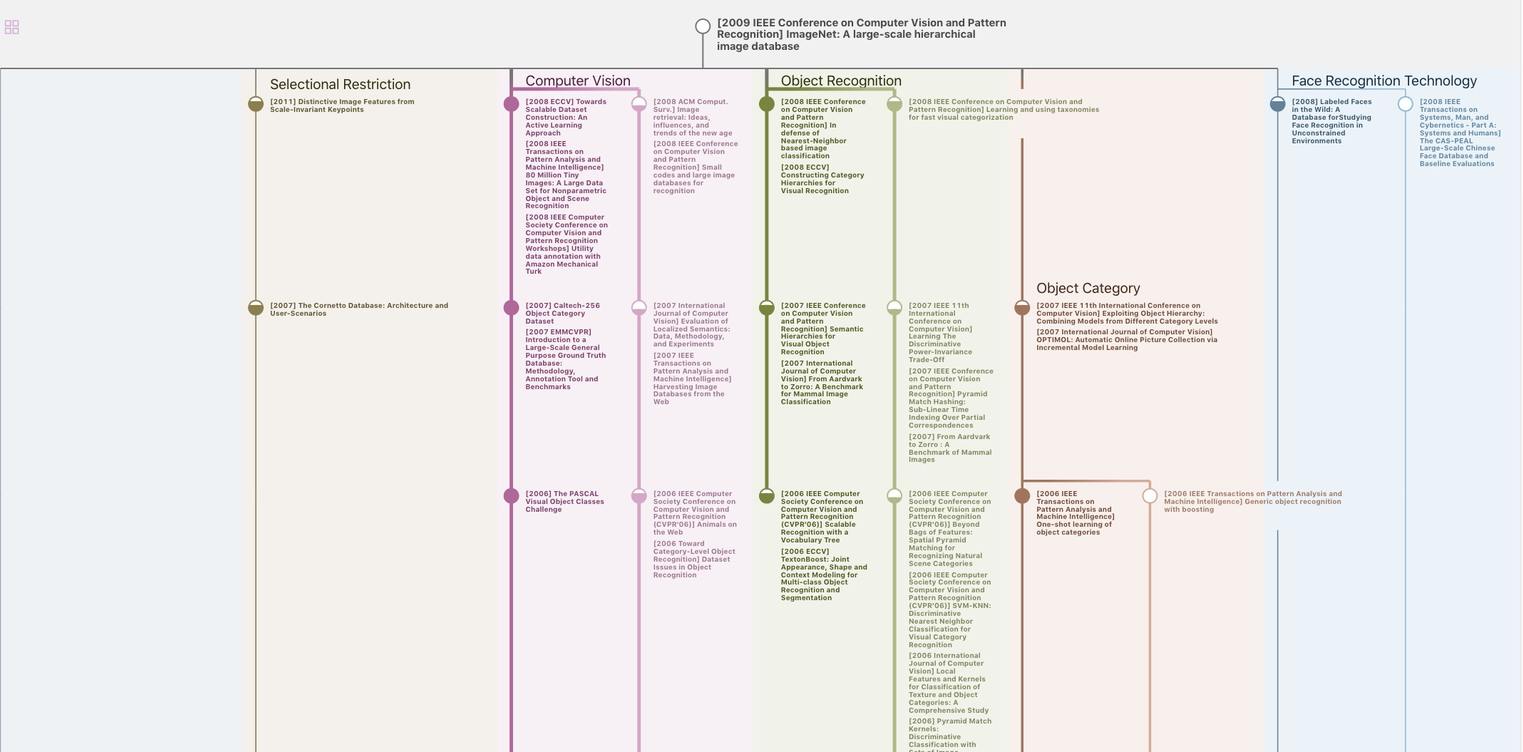
生成溯源树,研究论文发展脉络
Chat Paper
正在生成论文摘要