A Scalable Deep Reinforcement Learning Algorithm for Partially Observable Pursuit-Evasion Game
2022 International Conference on Machine Learning, Cloud Computing and Intelligent Mining (MLCCIM)(2022)
摘要
The cooperative control of multiple Unmanned Aerial Vehicles (UAVs) has become an essential topic in recent years since it is the foundation of numerous research fields, such as the pursuit-evasion games. In these games, multiple pursuers attempt to imprison the evaders who are trying to escape the capture within the constraints formulated by the environment. In this paper, we consider the decentralized and partially observable multi-UAV pursuit-evasion game with multiple static obstacles in a bounded two-dimensional environment. To solve the cooperative pursuit control problem of UAVs, we propose an Attention-based Multi-agent Deep Deterministic Policy Gradient (Att-MADDPG) algorithm based on the centralized critic and distributed actor structure. Specifically, we formulate the game as a partially-observable Markov decision process and handle the arbitrary number of observable agents with a self-attention module. Simulation results reveal that in the dynamic environment with obstacles, the improvement of our algorithm in terms of scalability and efficiency is significant compared to existing techniques.
更多查看译文
关键词
pursuit-evasion game,multi-agent reinforcement learning,attention mechanism,cooperating UAVs
AI 理解论文
溯源树
样例
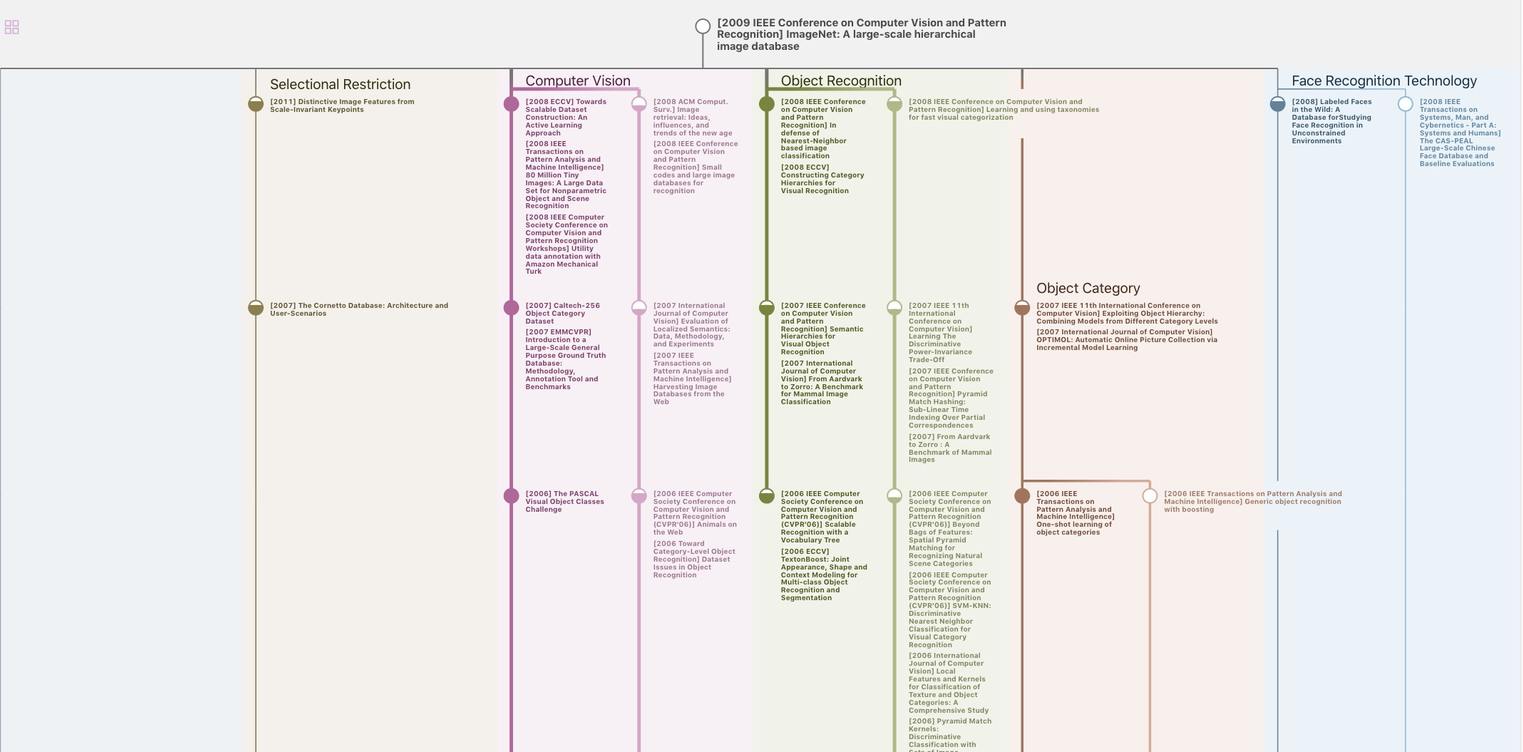
生成溯源树,研究论文发展脉络
Chat Paper
正在生成论文摘要