HARMONY: Heterogeneity-Aware Hierarchical Management for Federated Learning System
2022 55th IEEE/ACM International Symposium on Microarchitecture (MICRO)(2022)
摘要
Federated learning (FL) enables multiple devices to collaboratively train a shared model while preserving data privacy. However, despite its emerging applications in many areas, real-world deployment of on-device FL is challenging due to wildly diverse training capability and data distribution across heterogeneous edge devices, which highly impact both model performance and training efficiency. This paper proposes Harmony, a high-performance FL framework with heterogeneity-aware hierarchical management of training devices and training data. Unlike previous work that mainly focuses on heterogeneity in either training capability or data distribution, Harmony adopts a hierarchical structure to jointly handle both heterogeneities in a unified manner. Specifically, the two core components of Harmony are a global coordinator hosted by the central server and a local coordinator deployed on each participating device. Without accessing the raw data, the global coordinator first selects the participants, and then further reorganizes their training samples based on the accurate estimation of the runtime training capability and data distribution of each device. The local coordinator keeps monitoring the local training status and conducts efficient training with guidance from the global coordinator. We conduct extensive experiments to evaluate Harmony using both hardware and simulation testbeds on representative datasets. The experimental results show that Harmony improves the accuracy performance by 1.67% - 27.62%. In addition, Harmony effectively accelerates the training process up to $3.29\times$ and $1.84\times$ on average, and saves energy up to 88.41% and 28.04% on average.
更多查看译文
关键词
Federated learning,heterogeneous systems,mobile device
AI 理解论文
溯源树
样例
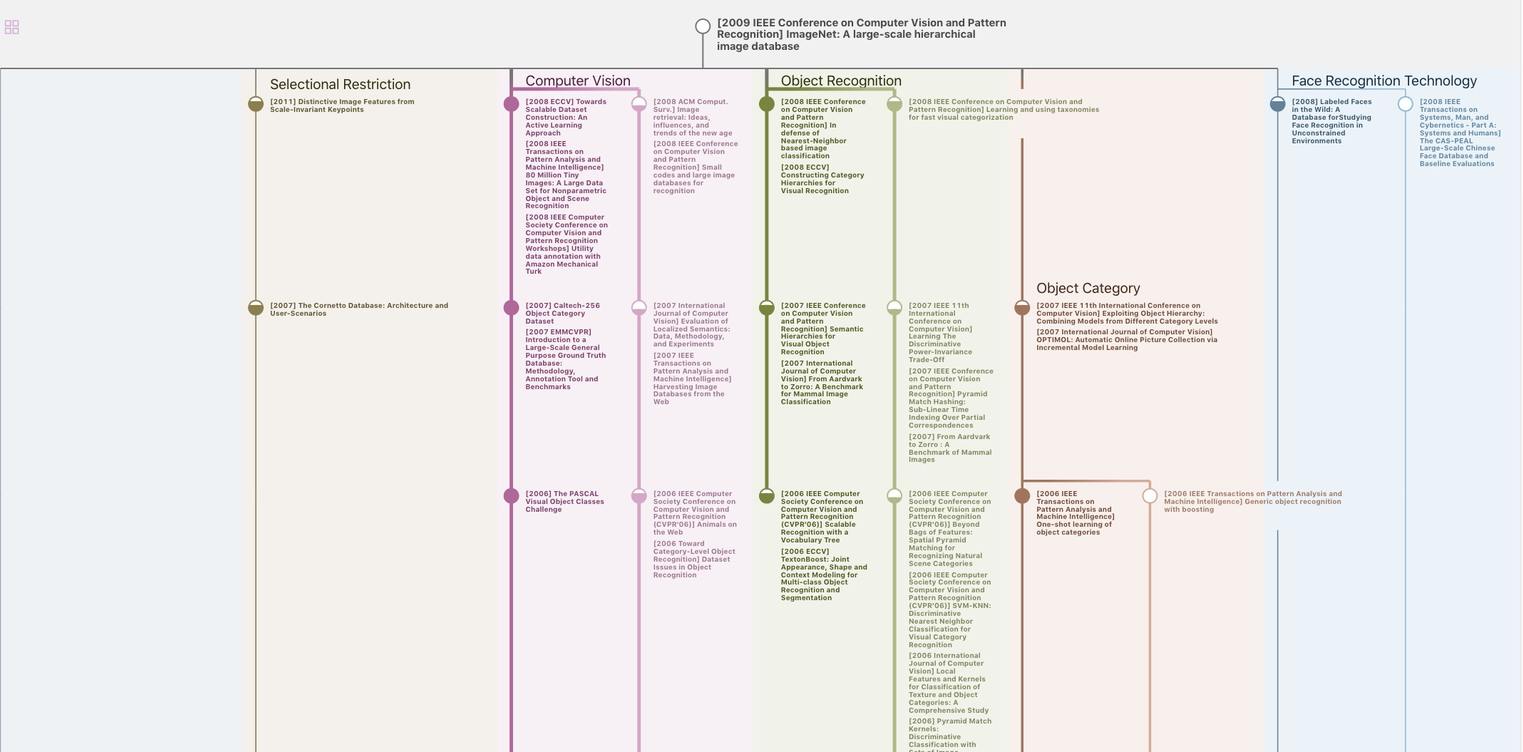
生成溯源树,研究论文发展脉络
Chat Paper
正在生成论文摘要