Architecting Decentralization and Customizability in DNN Accelerators for Hardware Defect Adaptation
IEEE Transactions on Computer-Aided Design of Integrated Circuits and Systems(2022)
摘要
The efficiency of machine intelligence techniques has improved noticeably in the embedded application domains thanks to the dedicated hardware accelerators for deep neural networks (DNNs). Despite the economic criticality of yield and reliability problems in advanced semiconductor nodes, these concerns have attracted limited attention in the context of embedded machine intelligence devices. The micro-architectural features of deep learning accelerators, when paired with the algorithmic characteristics of DNNs, unlock novel opportunities to tackle semiconductor reliability problems in embedded deep learning devices. While the fine-grained bypassing of the faulty processing elements reins the computational impact of hardware defects, a one-time training of DNNs with Hardware-Aware Dropout/Dropconnect techniques boosts model decentralization and facilitates accurate neural network inference in the degraded computational fabrics. Furthermore, on-device calibration methods can improve resilience even further without necessitating expensive defect compensation methods such as device-specific training. Our work confirms the potential for improving the yield, reliability, and operational lifetime of embedded machine intelligence devices through a highly practical co-design of DNNs and configurable hardware architectures.
更多查看译文
关键词
Deep learning hardware,fault tolerance,semiconductor defects,semiconductor yield improvement
AI 理解论文
溯源树
样例
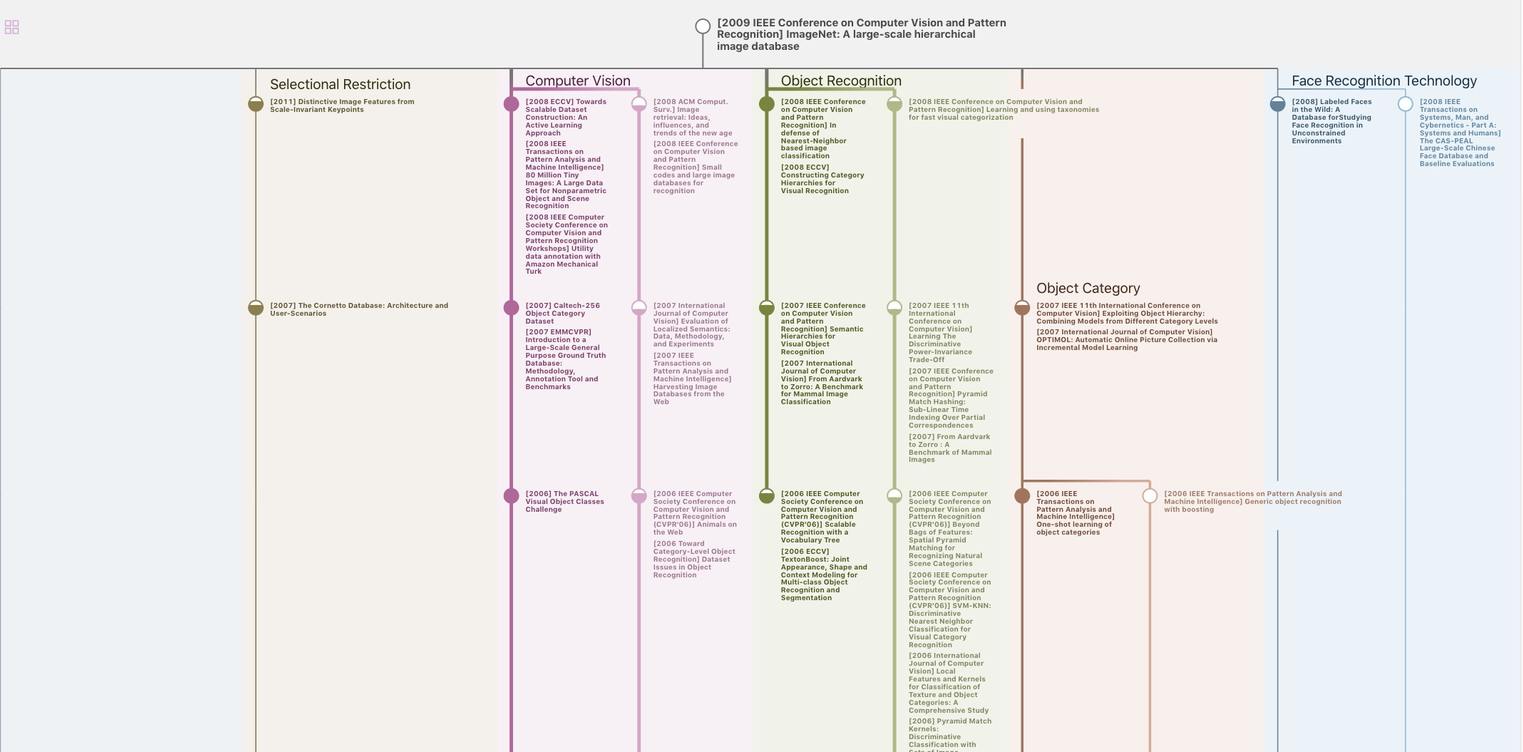
生成溯源树,研究论文发展脉络
Chat Paper
正在生成论文摘要