ViA: A Novel Vision-Transformer Accelerator Based on FPGA
IEEE Transactions on Computer-Aided Design of Integrated Circuits and Systems(2022)
摘要
Since Google proposed Transformer in 2017, it has made significant natural language processing (NLP) development. However, the increasing cost is a large amount of calculation and parameters. Previous researchers designed and proposed some accelerator structures for transformer models in field-programmable gate array (FPGA) to deal with NLP tasks efficiently. Now, the development of Transformer has also affected computer vision (CV) and has rapidly surpassed convolution neural networks (CNNs) in various image tasks. And there are apparent differences between the image data used in CV and the sequence data in NLP. The details in the models contained with transformer units in these two fields are also different. The difference in terms of data brings about the problem of the locality. The difference in the model structure brings about the problem of path dependence, which is not noticed in the existing related accelerator design. Therefore, in this work, we propose the ViA, a novel vision transformer (ViT) accelerator architecture based on FPGA, to execute the transformer application efficiently and avoid the cost of these challenges. By analyzing the data structure in the ViT, we design an appropriate partition strategy to reduce the impact of data locality in the image and improve the efficiency of computation and memory access. Meanwhile, by observing the computing flow of the ViT, we use the half-layer mapping and throughput analysis to reduce the impact of path dependence caused by the shortcut mechanism and fully utilize hardware resources to execute the Transformer efficiently. Based on optimization strategies, we design two reuse processing engines with the internal stream, different from the previous overlap or stream design patterns. In the stage of the experiment, we implement the ViA architecture in Xilinx Alveo U50 FPGA and finally achieved similar to 5.2 times improvement of energy efficiency compared with NVIDIA Tesla V100, and 4-10 times improvement of performance compared with related accelerators based on FPGA, that obtained nearly 309.6 GOP/s computing performance in the peek.
更多查看译文
关键词
Accelerator,field-programmable gate array (FPGA),vision transformer (ViT)
AI 理解论文
溯源树
样例
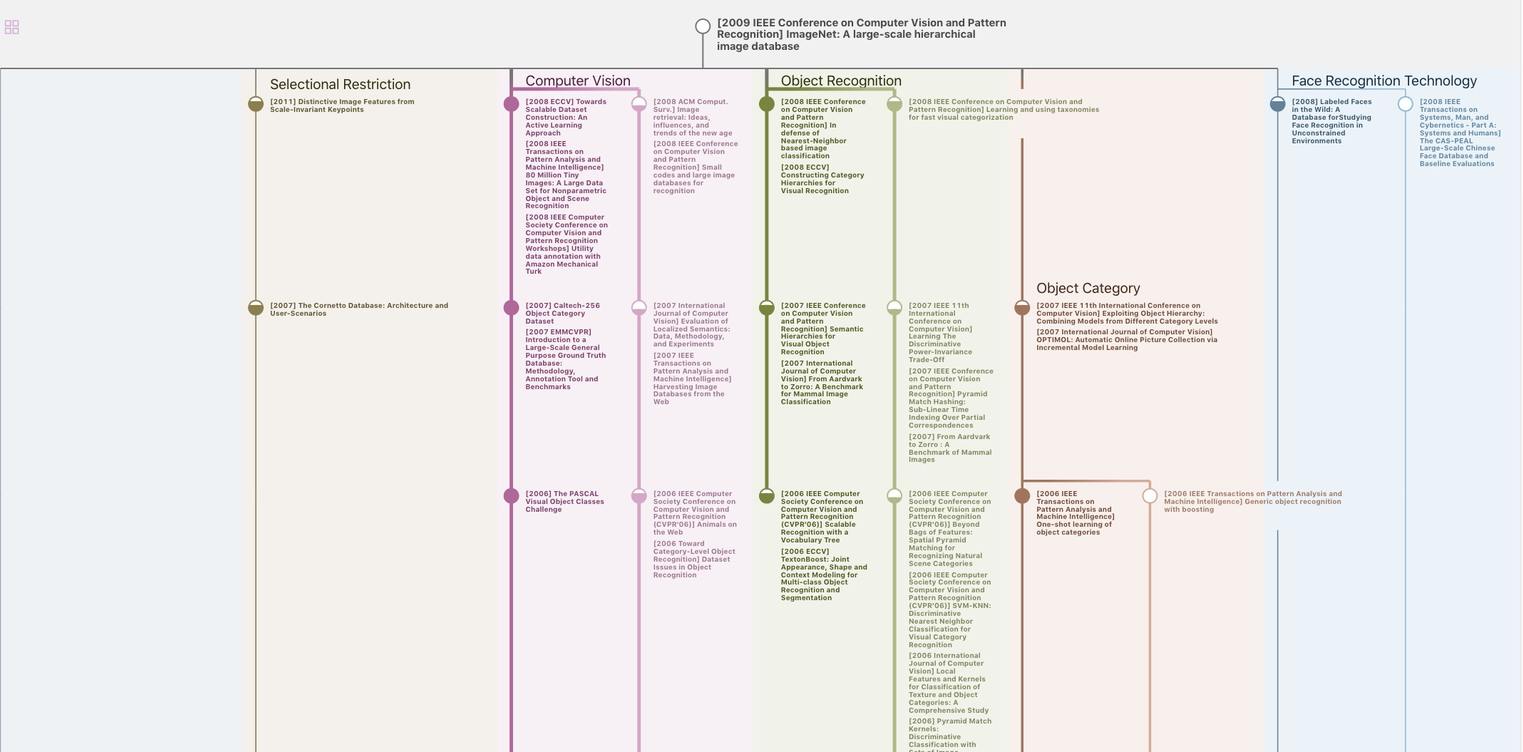
生成溯源树,研究论文发展脉络
Chat Paper
正在生成论文摘要