Characterizing and Understanding End-to-End Multi-Modal Neural Networks on GPUs
IEEE Computer Architecture Letters(2022)
摘要
Multi-modal neural networks have become increasingly pervasive in many machine learning application domains due to their superior accuracy by fusing various modalities. However, they present many unique characteristics such as multi-stage execution, frequent synchronization and high heterogeneity, which are not well understood in the system and architecture community. In this article, we first present and characterize a set of multi-modal neural network workloads of different sizes at inference stage. We then explore their important implications from system and architecture aspects. We hope that our work can help guide future software/hardware design and optimization for efficient inference of multi-modal DNN applications.
更多查看译文
关键词
computation analysis,characterization,deep learning,Multi-modal neural networks
AI 理解论文
溯源树
样例
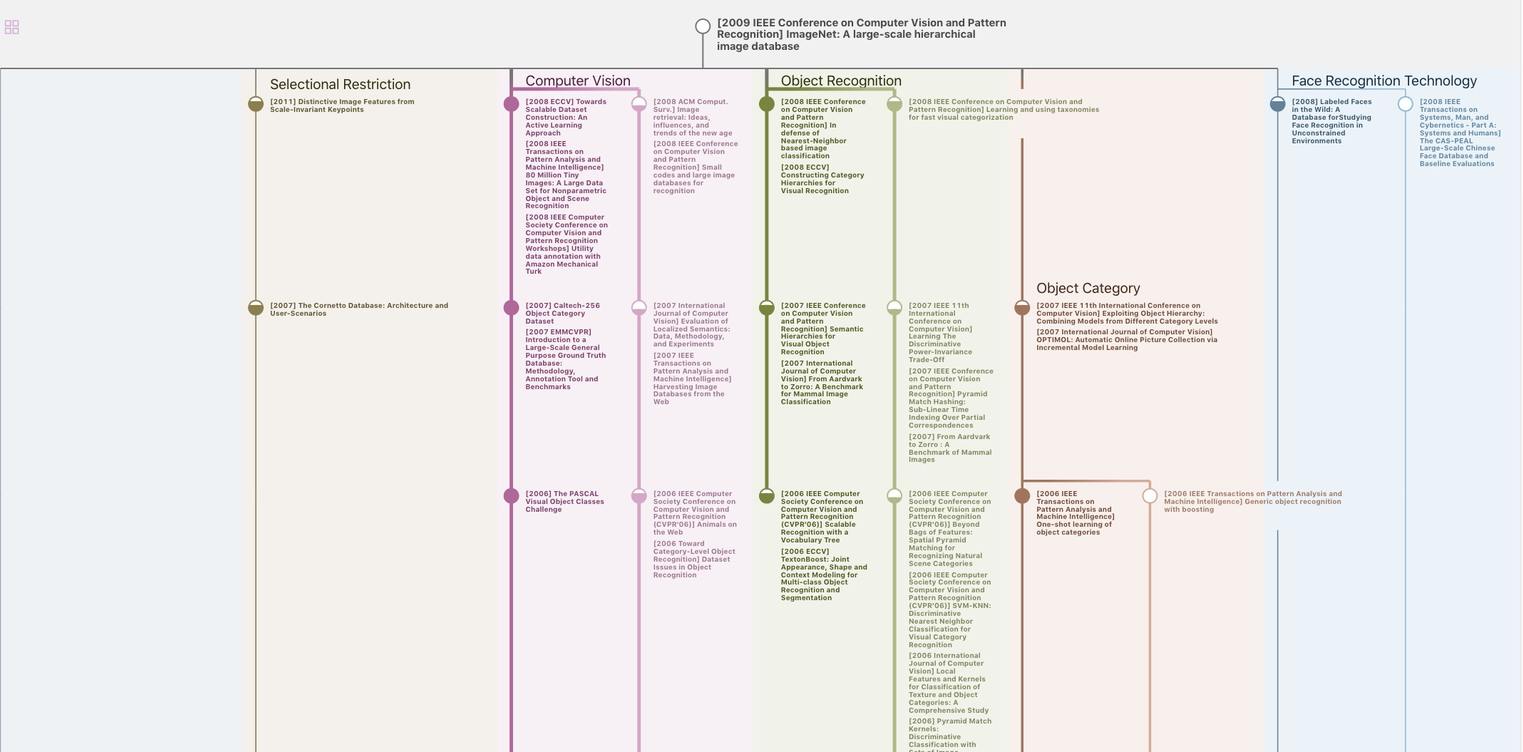
生成溯源树,研究论文发展脉络
Chat Paper
正在生成论文摘要