Improving Multiple Machine Vision Tasks in the Compressed Domain
2022 26th International Conference on Pattern Recognition (ICPR)(2022)
摘要
There is a growing number of images that are analyzed by machines rather than just humans. Recently, most machine vision tasks are based on decoded images which require an image compression (encoding/decoding) framework. However, using the decoded images in the pixel-domain has two drawbacks: 1) the complexity is high for the decoder part, 2) the accuracy (e.g., mIoU, mean absolute error, and average precision) of machine vision tasks will be degraded since decoded images only aim to optimize the human perceived quality (e.g., PSNR) so that information required for machine vision tasks will be lost during the decoding process. In this paper, we improve the machine vision tasks in the compressed domain. 1) A gate module is utilized to effectively select some compressed-domain features. 2) Knowledge distillation is introduced to improve the accuracy. 3) A training strategy is explored to support multiple tasks including the image compression. The experimental results show that we can achieve better rate-accuracy/distortion and lower complexity compared with the state-of-the-art pixel-domain work that can take both machine and human vision tasks.
更多查看译文
关键词
multiple machine vision tasks,domain
AI 理解论文
溯源树
样例
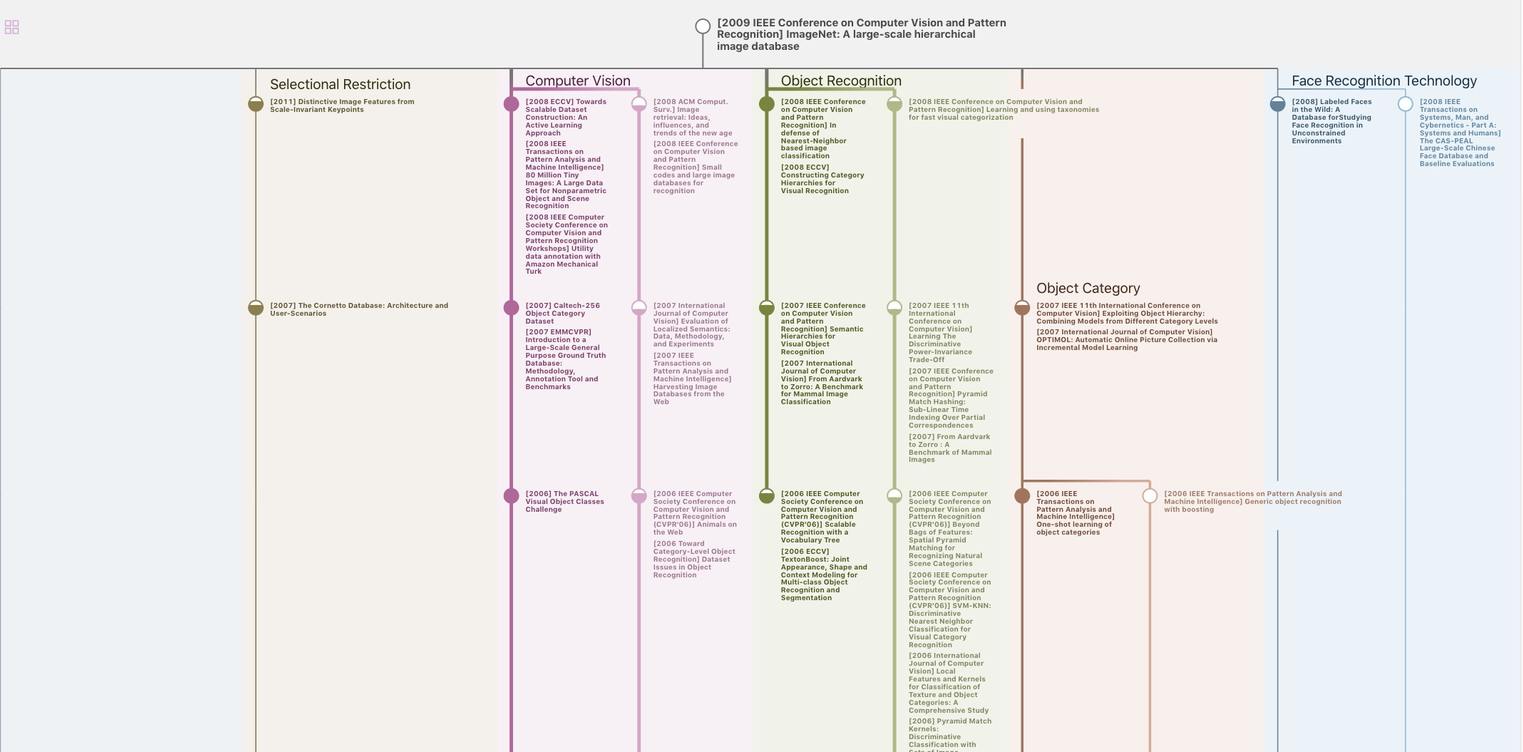
生成溯源树,研究论文发展脉络
Chat Paper
正在生成论文摘要