Fast and resource-efficient CNNs for Radar Interference Mitigation on Embedded Hardware
2022 19th European Radar Conference (EuRAD)(2022)
摘要
Recently, Convolutional Neural Networks have demonstrated impressive performance in automotive radar interference mitigation tasks. However, high-performance hardware is typically indispensable for low-latency applications of Convolutional Neural Network models, which prohibits usage in resource-constrained automotive environments. In this paper, we propose a scalable, resource-efficient accelerator for interference mitigation, which enables low-latency and low-memory computations. In extensive experiments we contrast three substantially different implementations: (i) a software implementation in Python, (ii) a firmware implementation on an ARM CPU using either floating-point, or fixed-point arithmetic, and (iii) a Field-Programmable Gate Array implementation. These different implementations are analyzed with respect to latency, memory, and interference mitigation performance. We conclude, that hardware accelerated inference is magnitudes faster than CPU-based inference while increasing power consumption just slightly. The use of integer arithmetic due to quantization accelerates the computation without impairing interference mitigation performance. Furthermore, the parallelization of layers in programmable logic further reduces latency. This parallelization introduces a trade-off with resource requirements and power consumption versus latency, and is therefore a scalable approach for different application and hardware demands.
更多查看译文
关键词
Doppler measurement,Vehicle safety,Advanced driver assistance systems,Convolutional neural networks,Field programmable gate arrays
AI 理解论文
溯源树
样例
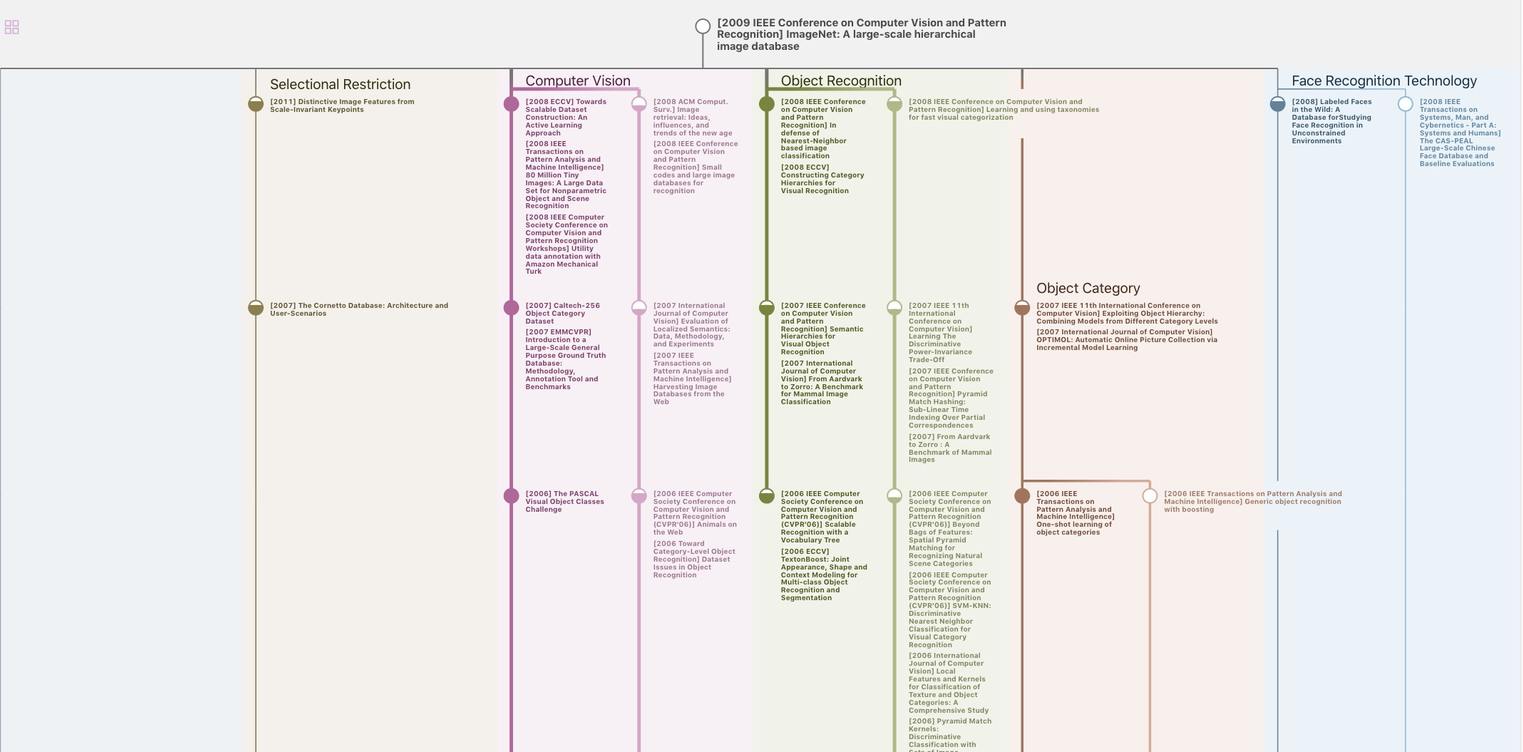
生成溯源树,研究论文发展脉络
Chat Paper
正在生成论文摘要