Few-shot Multi-domain Fault Diagnosis for Planetary Gearbox in Nuclear Circulating Water Pump
2022 IEEE International Conference on Sensing, Diagnostics, Prognostics, and Control ( SDPC)(2022)
摘要
Deep learning (DL)-based methods can realize superior performance in fault diagnosis with sufficient samples and consistent distribution. However, lacking labeled samples hampers DL’s application in nuclear circulating water pump (NCWP) fault diagnosis. Moreover, complex and varying working conditions of NCWP would remarkably lower the performance of the DL-based model because domain shift occurs in data distribution. To address these problems, the triplet adaptive attention multiscale Resnet (TAAMR) with ensemble empirical mode decomposition-local maximum mean discrepancy (EEMD-LMMD) generalized feature extraction is introduced for few-shot multi-domain fault diagnosis in NCWP planetary gearbox. Based on the EEMD-LMMD mechanism, domain-invariant features can be extracted more efficiently. TAAMR consists of an adaptive attention module, a multi-scale Resnet, and a triplet structure. With few-shot multi-domain learning, TAAMR utilizes the adaptive attention module to realize the identification of the importance of multiscale features. And the triplet structure is designed to optimize the distribution of features. The effectiveness of the proposed TAAMR is demonstrated on the NCWP test bench datasets, and the results show good adaptability in different working conditions.
更多查看译文
关键词
Adaptive attention,generalized feature extraction,few-shot multi-domain fault diagnosis,planetary gearbox,nuclear circulating water pump
AI 理解论文
溯源树
样例
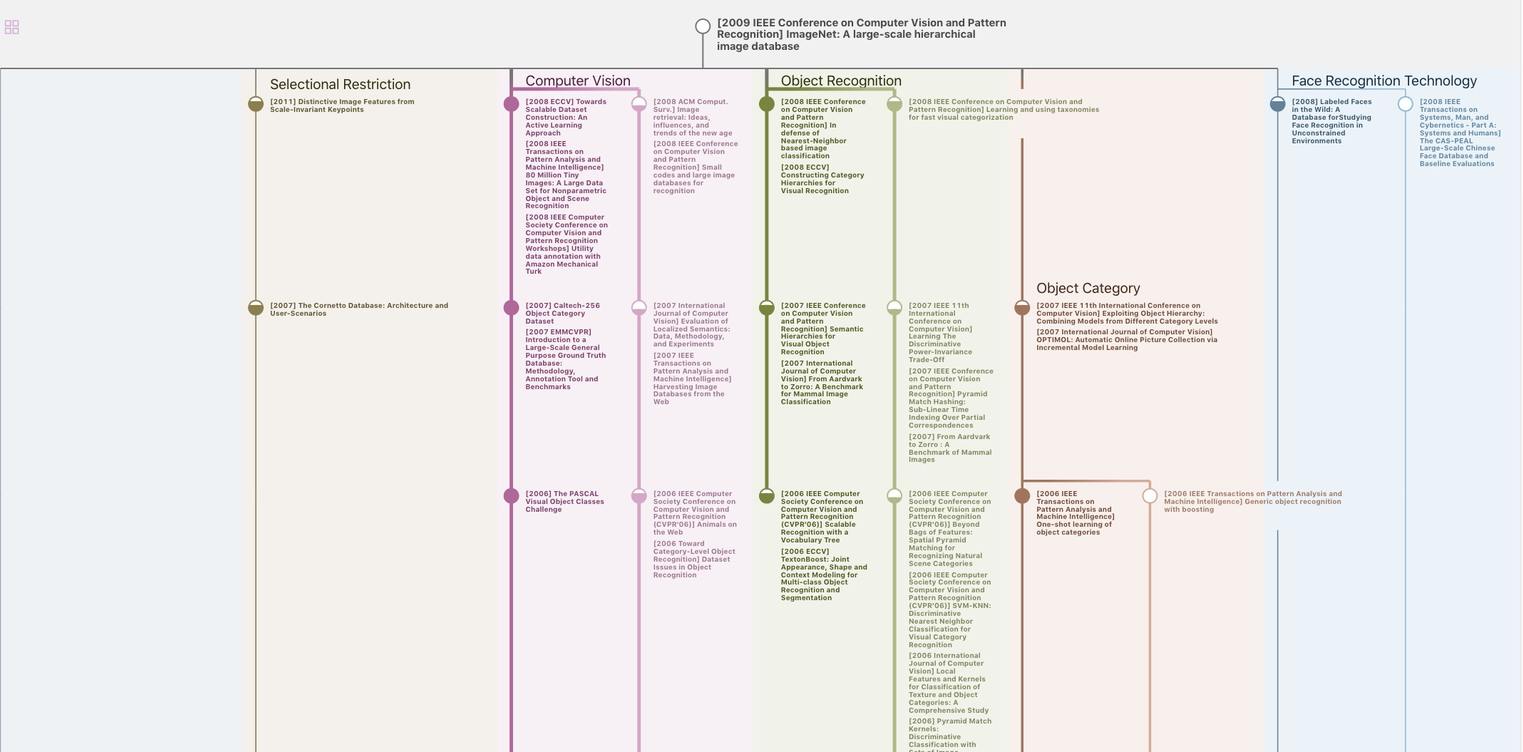
生成溯源树,研究论文发展脉络
Chat Paper
正在生成论文摘要