Darkside: 2.6GFLOPS, 8.7mW Heterogeneous RISC-V Cluster for Extreme-Edge On-Chip DNN Inference and Training
ESSCIRC 2022- IEEE 48th European Solid State Circuits Conference (ESSCIRC)(2022)
摘要
Extreme-edge applications using Deep Learning (DL) have strict requirements in terms of latency, throughput, accuracy, and flexibility. Heterogeneous clusters are promising architectural solutions that combine the programmability of DSP-enhanced cores with the performance and efficiency boost of specialized accelerators. We present Darkside, a System-on-Chip with a heterogeneous cluster of 8 RISC-V cores enhanced with 2-b to 32-b mixed-precision integer arithmetic. To further speed-up key compute-intensive Deep Neural Network (DNN) kernels, the cluster is enriched with three specialized digital accelerators: an accelerator for low-data-reuse depthwise convolution kernels (up to 30 MAC/cycle); a minimal overhead datamover to marshal 1-b to 32-b data on-the-fly; a 16-b floating point Tensor Product Engine (TPE) for tiled matrix-multiplication acceleration. Darkside is implemented in 65nm CMOS technology. The cluster achieves a peak integer performance of 65 GOPS and a peak efficiency of 835 GOPS/W when working on 2-b integer DNN kernels. When targeting floating-point tensor operations, the TPE provides up to 18.2 GFLOPS of performance or 300 GFLOPS/W of efficiency – enough to enable on-chip floating-point training at competitive speed coupled with ultra-low power quantized inference.
更多查看译文
关键词
Extreme-Edge,Heterogeneous Cluster,Tensor Product Engine,Ultra-Low-Power AI
AI 理解论文
溯源树
样例
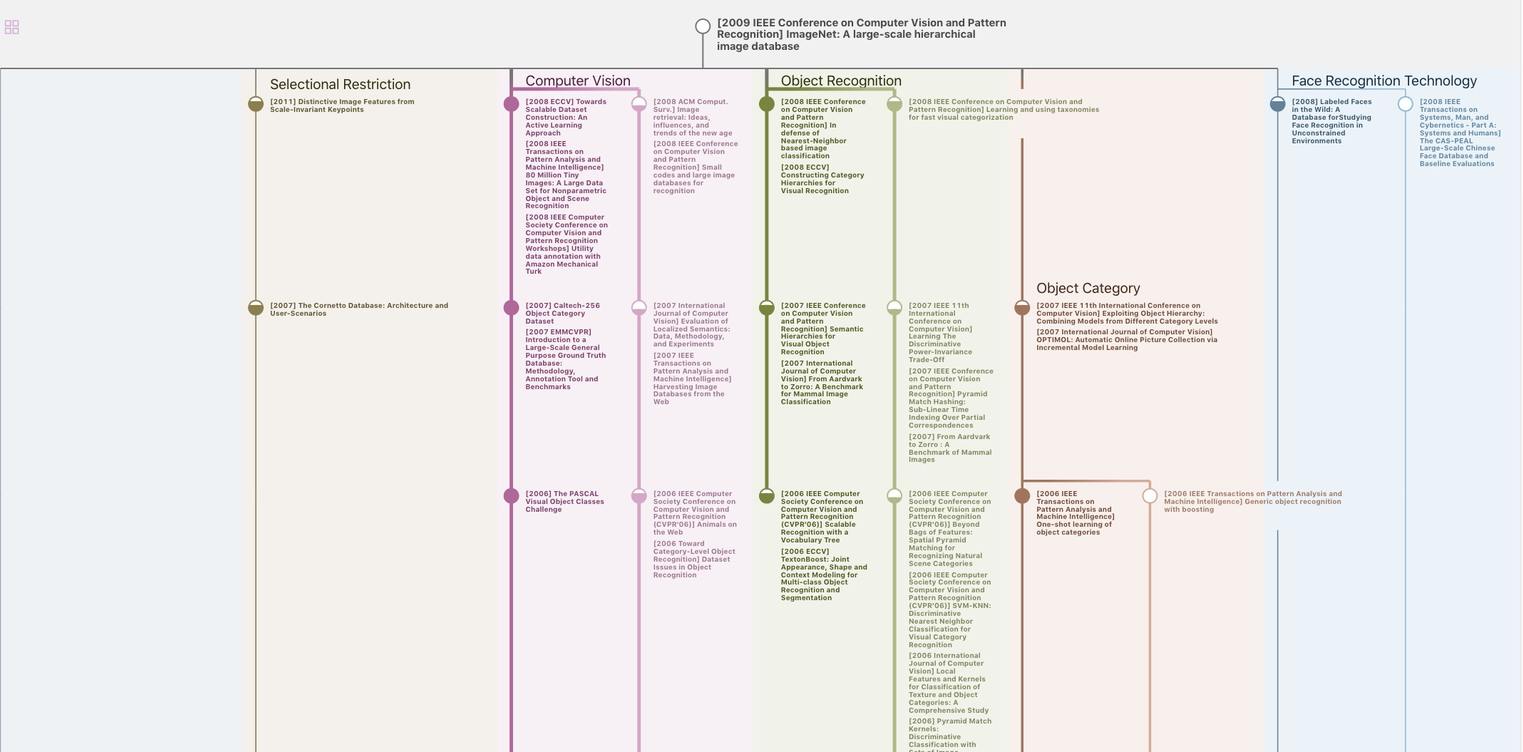
生成溯源树,研究论文发展脉络
Chat Paper
正在生成论文摘要