3D Reconstruction from 2D Images: A Two-part Autoencoder-like Tool
2022 IEEE International Conference on Systems, Man, and Cybernetics (SMC)(2022)
AI Read Science
Must-Reading Tree
Example
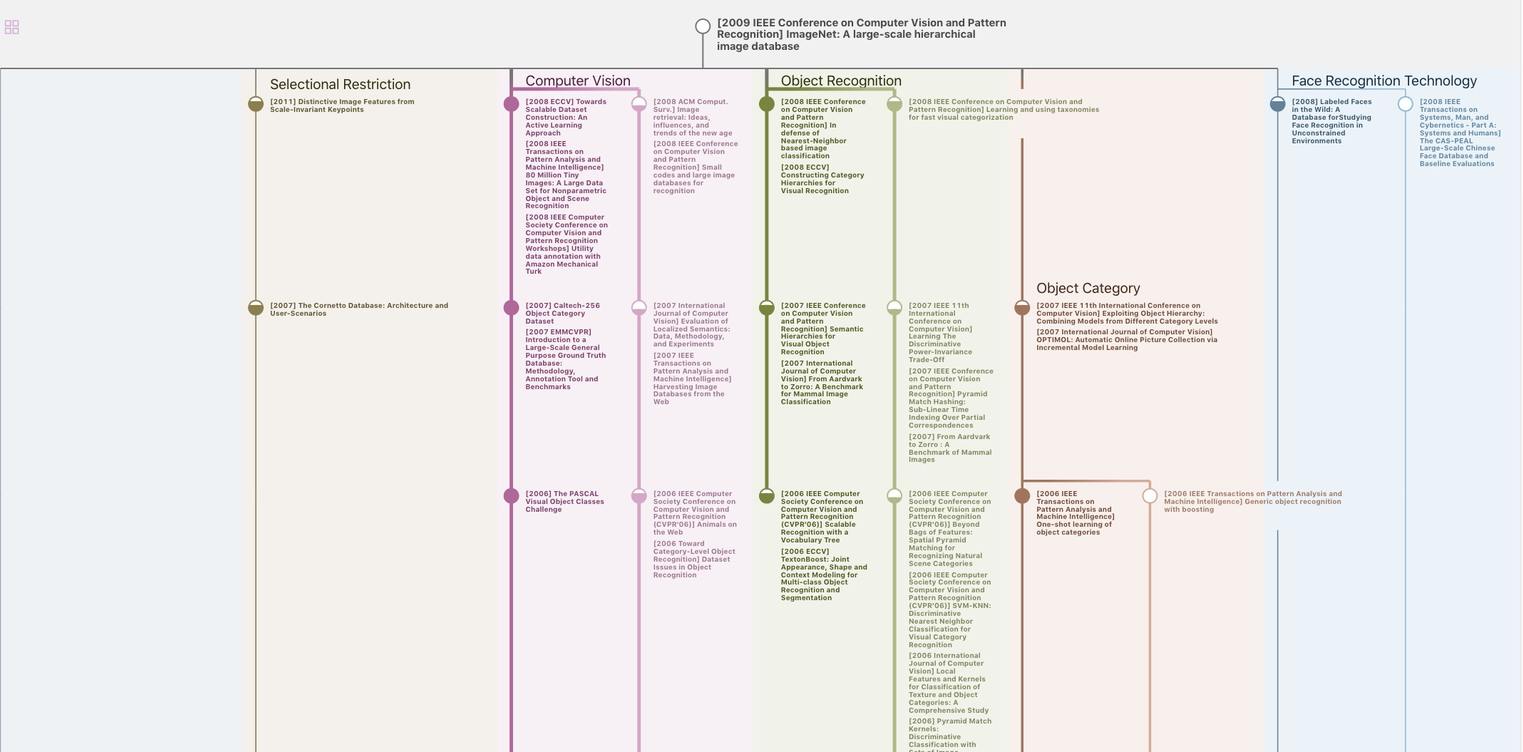
Generate MRT to find the research sequence of this paper
Chat Paper
Summary is being generated by the instructions you defined