ConvLSTM-CRF: Sea Ice Concentration Prediction with ConvLSTM and Conditional Random Fields
2022 IEEE International Conference on Systems, Man, and Cybernetics (SMC)(2022)
摘要
Predicting the Arctic sea ice concentration (SIC) has an essential guiding role in understanding climate change trends, resource extraction and route planning. Existing deep learning models still have the problem that it is challenging to utilize the global spatial information of SIC, and the predictions of boundary regions are not accurate enough. In this paper, we propose a new deep learning model, namely ConvLSTM-CRF, to predict the monthly sea ice concentration in the Arctic. We add a dense conditional random fields to the ConvLSTM, which further extracts global spatial information and can predict SIC more accurately. The experimental results show that compared with ConvLSTM, our model has a great improvement in the overall prediction accuracy and has more accurate predictions in the SIC boundary region, especially in the melting and freezing seasons when the SIC changes drastically. Our model also shows better prediction performance when making iterative predictions. In addition, ConvLSTM-CRF can be applied to similar time series forecasting problems, such as precipitation forecasting and snowfall forecasting.
更多查看译文
关键词
Sea ice prediction,SIC prediction,Deep learning,ConvLSTM,Conditional random fields
AI 理解论文
溯源树
样例
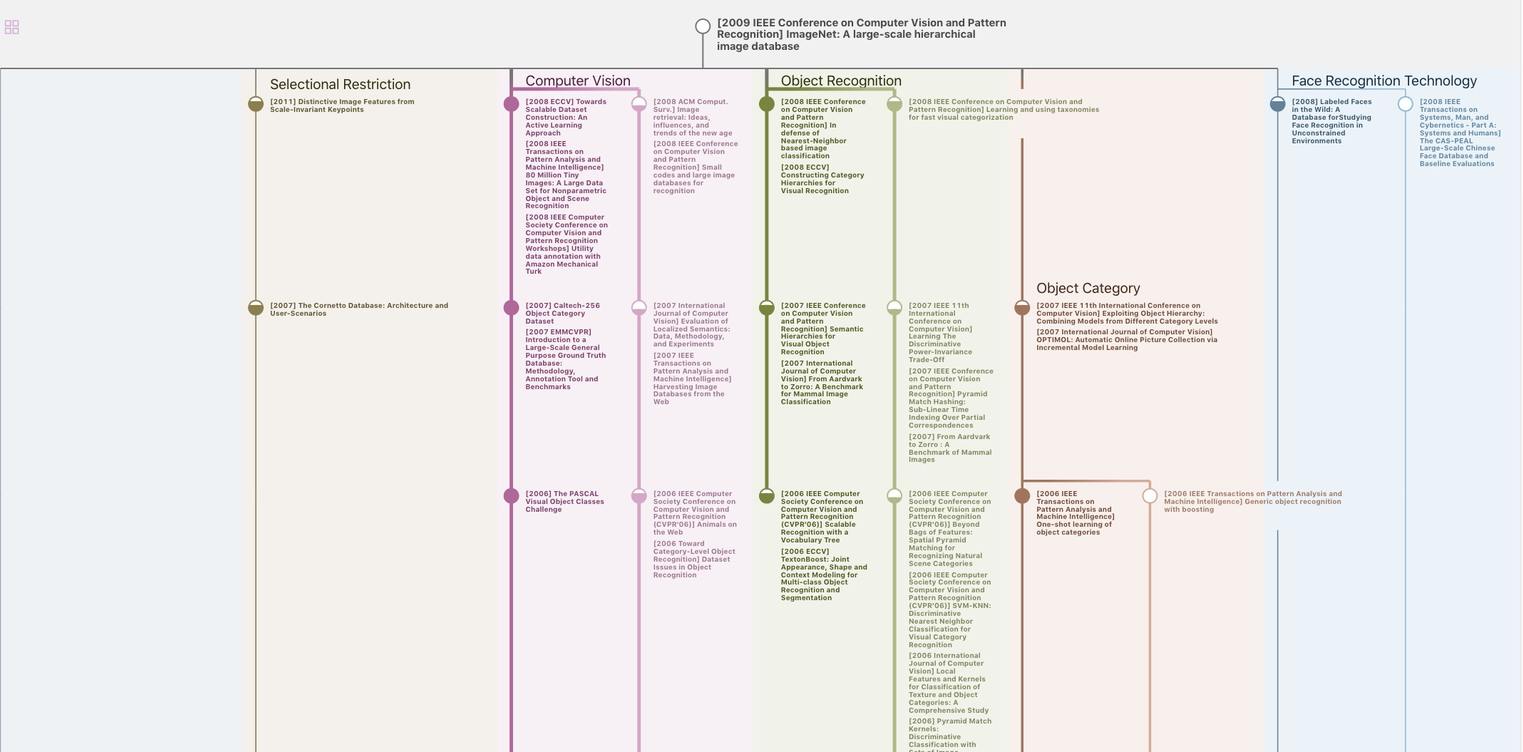
生成溯源树,研究论文发展脉络
Chat Paper
正在生成论文摘要