HI-Net: Boosting Self-Supervised Indoor Depth Estimation via Pose Optimization
IEEE Robotics and Automation Letters(2023)
摘要
Pose estimation plays a critical role in self-supervised monocular depth estimation for indoor scenes, especially those involving complex ego-motion. In this letter, we leverage the two-view geometry constraints into pose estimation to boost the accuracy of pose estimation, which ultimately improves the performance of self-supervised depth estimation. Specifically, we decompose pose estimation into two steps: initial homography estimation and iterative residual refinement. We first introduce a Homography Estimation Module (HEM) to estimate large 3-DoF rotations. Then, we refine the 6-DoF residual pose estimation with an Iterative Residual Refinement Module (IRM). Finally, the supervision signal is generated with the refined pose and used for the training of DepthNet. Experiments on the NYU depth V2 dataset show that our pose estimation approach significantly improves the performance of DepthNet, and the proposed method achieves state-of-the-art depth estimation results. Furthermore, experiments on the ScanNet dataset demonstrate the generalization ability of our method for both pose estimation and depth estimation.
更多查看译文
关键词
Deep learning for visual perception,deep learning methods,visual learning
AI 理解论文
溯源树
样例
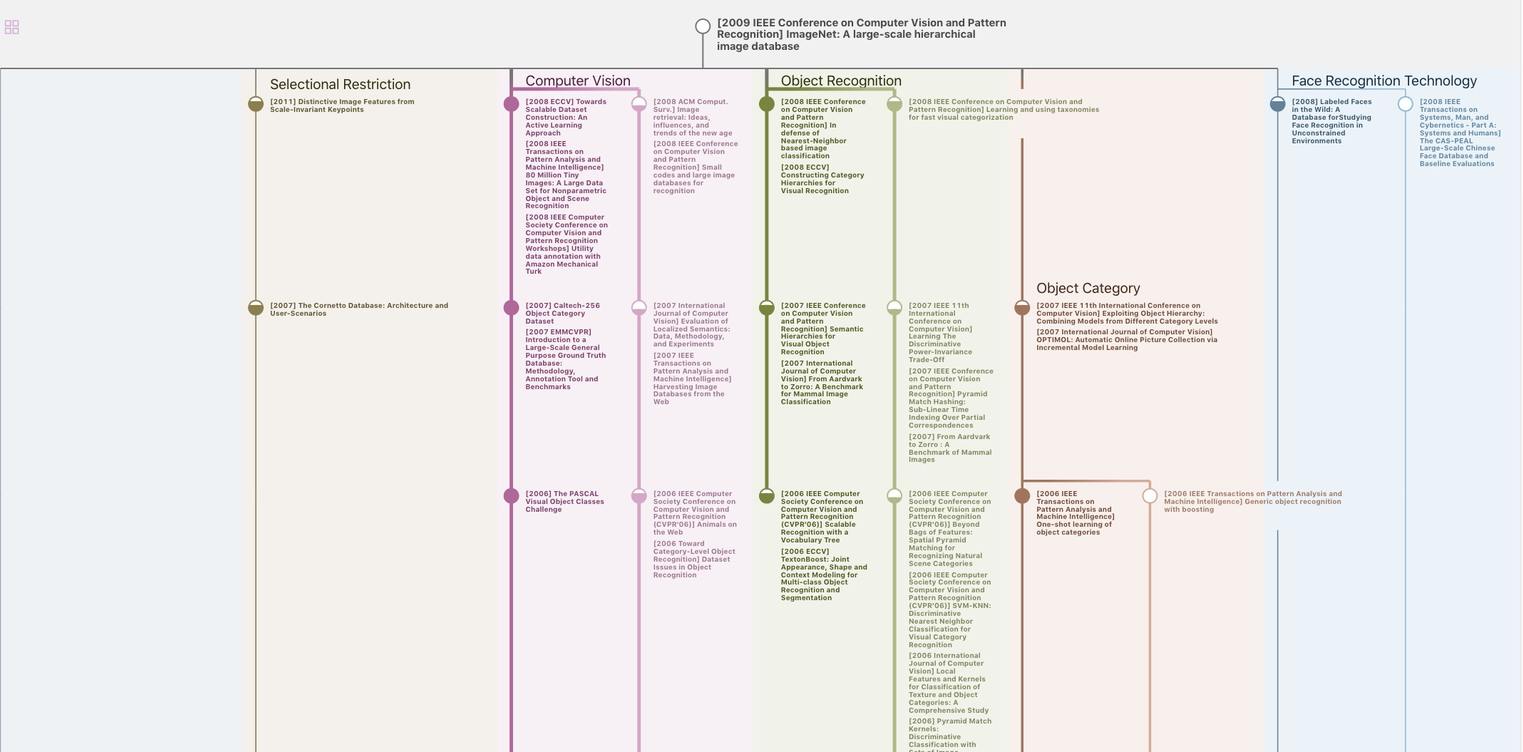
生成溯源树,研究论文发展脉络
Chat Paper
正在生成论文摘要