Hardware/Software Co-Exploration for Graph Neural Architectures on FPGAs
2022 IEEE Computer Society Annual Symposium on VLSI (ISVLSI)(2022)
摘要
The success of graph neural networks (GNNs) in the past years has aroused growing interest and effort in designing the best models to handle graph-structured data. Meanwhile, the neural architecture search (NAS) technique has been witnessed to rival against human experts in discovering efficient network topology. Most recently, it has been applied to the field of GNN engineering. However, despite the growing interest in GNN accelerator designs, existing works on graph neural architecture search all concentrate on software (SW) and do not consider hardware (HW) constraints at all, which often leads to sub-optimal system performance when the resulting networks are deployed on hardware accelerators. To address this problem, in this paper we propose a SW-HW co-design framework, namely FGNAS, for automating the search and deployment of GNNs on FPGAs. Experimental results on common benchmark datasets Cora, CiteCeer, and PubMed show that compared with a two-step method built from the state-of-the-art graph NAS framework, FGNAS achieves up to 4.8% improvement accuracy and 3x speedup under the same hardware constraint.
更多查看译文
关键词
Neural Architecture Search,Graph Neural Networks,FPGA
AI 理解论文
溯源树
样例
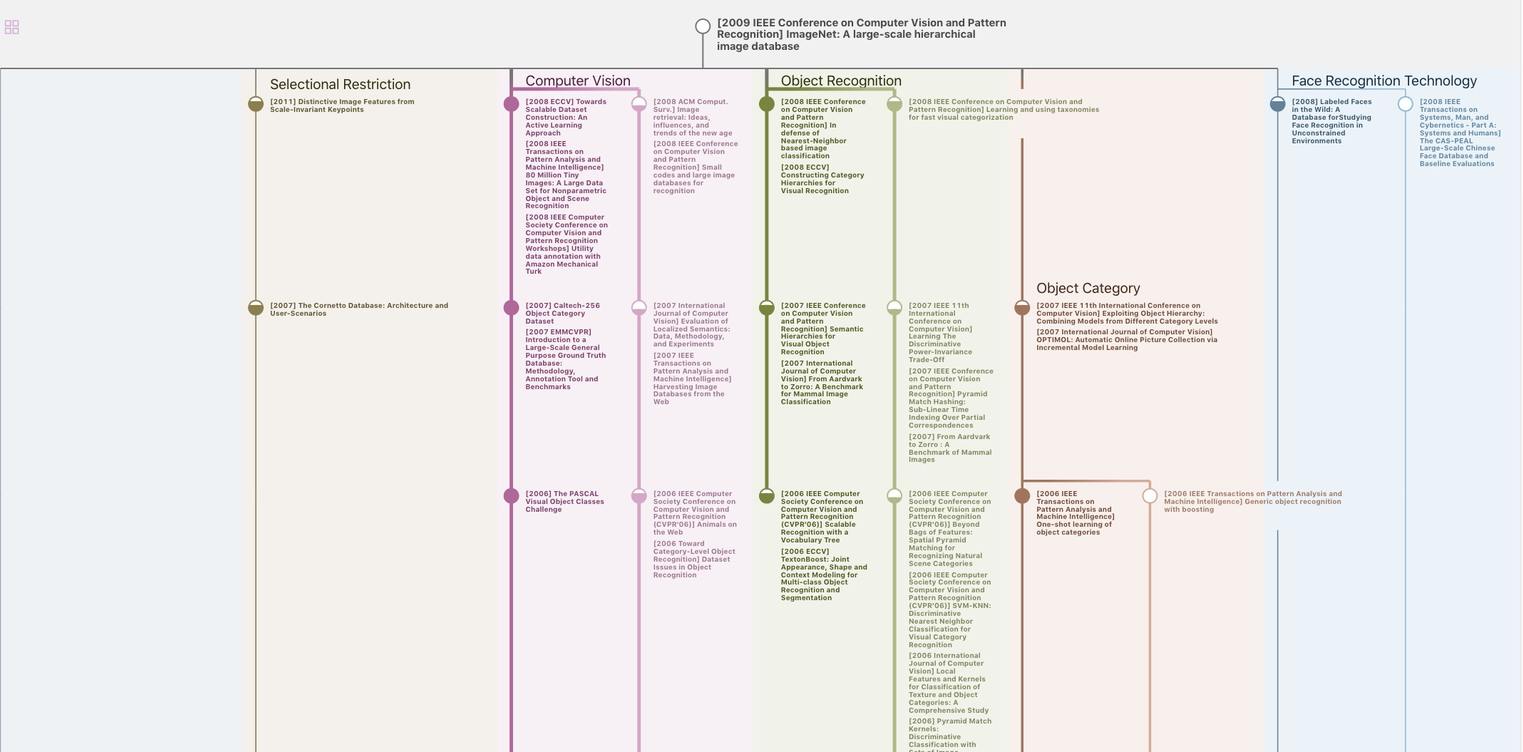
生成溯源树,研究论文发展脉络
Chat Paper
正在生成论文摘要