End-to-end modeling of variability-aware neural networks based on resistive-switching memory arrays
2022 IFIP/IEEE 30th International Conference on Very Large Scale Integration (VLSI-SoC)(2022)
摘要
Resistive-switching random access memory (RRAM) is a promising technology that enables advanced applications in the field of in-memory computing (IMC). By operating the memory array in the analogue domain, RRAM-based IMC architectures can dramatically improve the energy efficiency of deep neural networks (DNNs). However, achieving a high inference accuracy is challenged by significant variation of RRAM conductance levels, which can be compensated by (i) advanced programming techniques and (ii) variability-aware training (VAT) algorithms. In both cases, however, detailed knowledge and accurate physics-based statistical models of RRAM are needed to develop programming and VAT methodologies. This work presents an end-to-end approach to the development of highly-accurate IMC circuits with RRAM, encompassing the device modeling, the precise programming algorithm, and the VAT simulations to maximize the DNN classification accuracy in presence of conductance variations.
更多查看译文
关键词
Deep neural networks,in-memory computing,device modeling,resistive-switching random access memory (RRAM),artificial intelligence
AI 理解论文
溯源树
样例
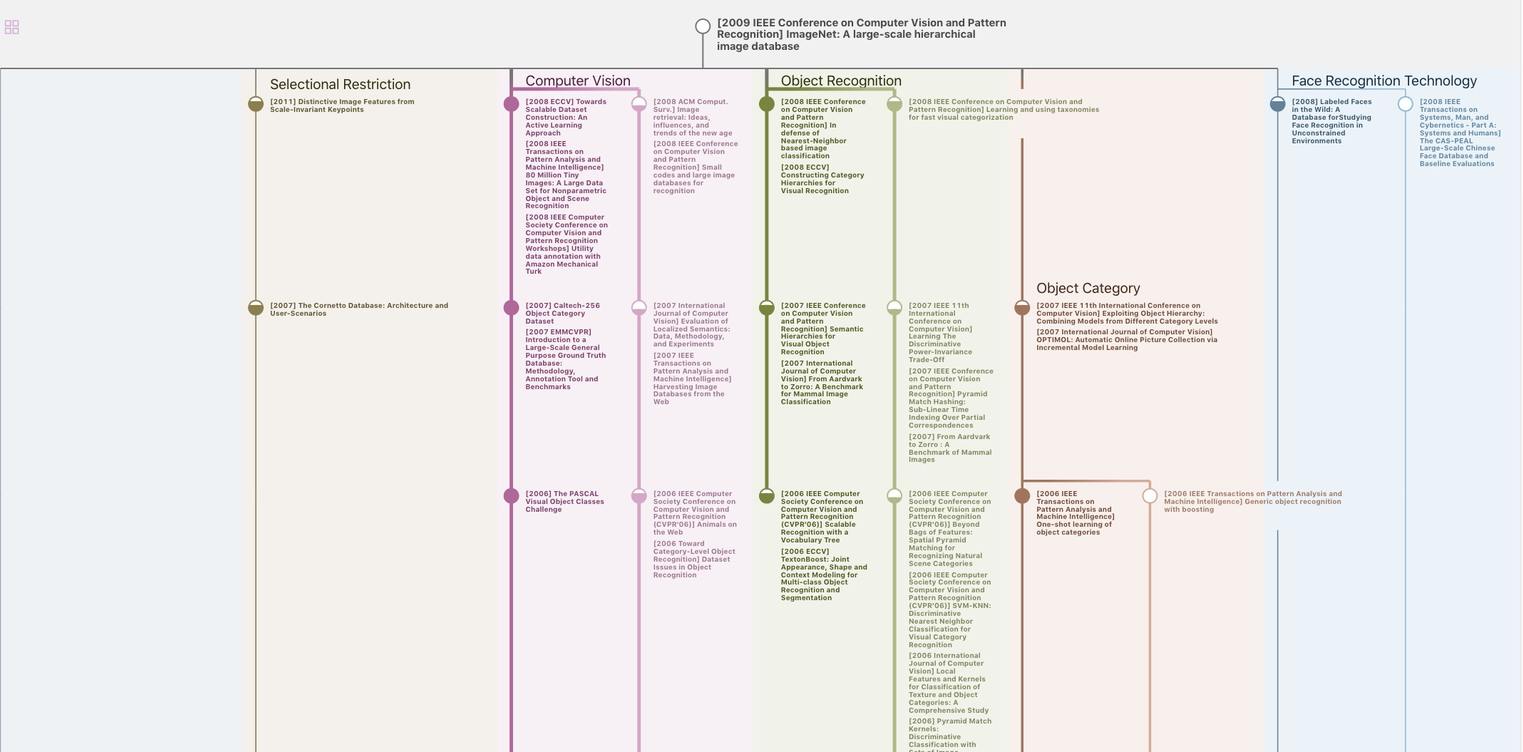
生成溯源树,研究论文发展脉络
Chat Paper
正在生成论文摘要