Probabilistic Neural Circuits leveraging AI-Enhanced Codesign for Random Number Generation
arxiv(2022)
摘要
Stochasticity is ubiquitous in the world around us. However, our predominant computing paradigm is deterministic. Random number generation (RNG) can be a computationally inefficient operation in this system especially for larger workloads. Our work leverages the underlying physics of emerging devices to develop probabilistic neural circuits for RNGs from a given distribution. However, codesign for novel circuits and systems that leverage inherent device stochasticity is a hard problem. This is mostly due to the large design space and complexity of doing so. It requires concurrent input from multiple areas in the design stack from algorithms, architectures, circuits, to devices. In this paper, we present examples of optimal circuits developed leveraging AI-enhanced codesign techniques using constraints from emerging devices and algorithms. Our AI-enhanced codesign approach accelerated design and enabled interactions between experts from different areas of the microelectronics design stack including theory, algorithms, circuits, and devices. We demonstrate optimal probabilistic neural circuits using magnetic tunnel junction and tunnel diode devices that generate an RNG from a given distribution.
更多查看译文
关键词
Probabilistic Devices,Probabilistic Neural Circuits,AI-Enhanced Codesign,Codesign,Neuromorphic computing
AI 理解论文
溯源树
样例
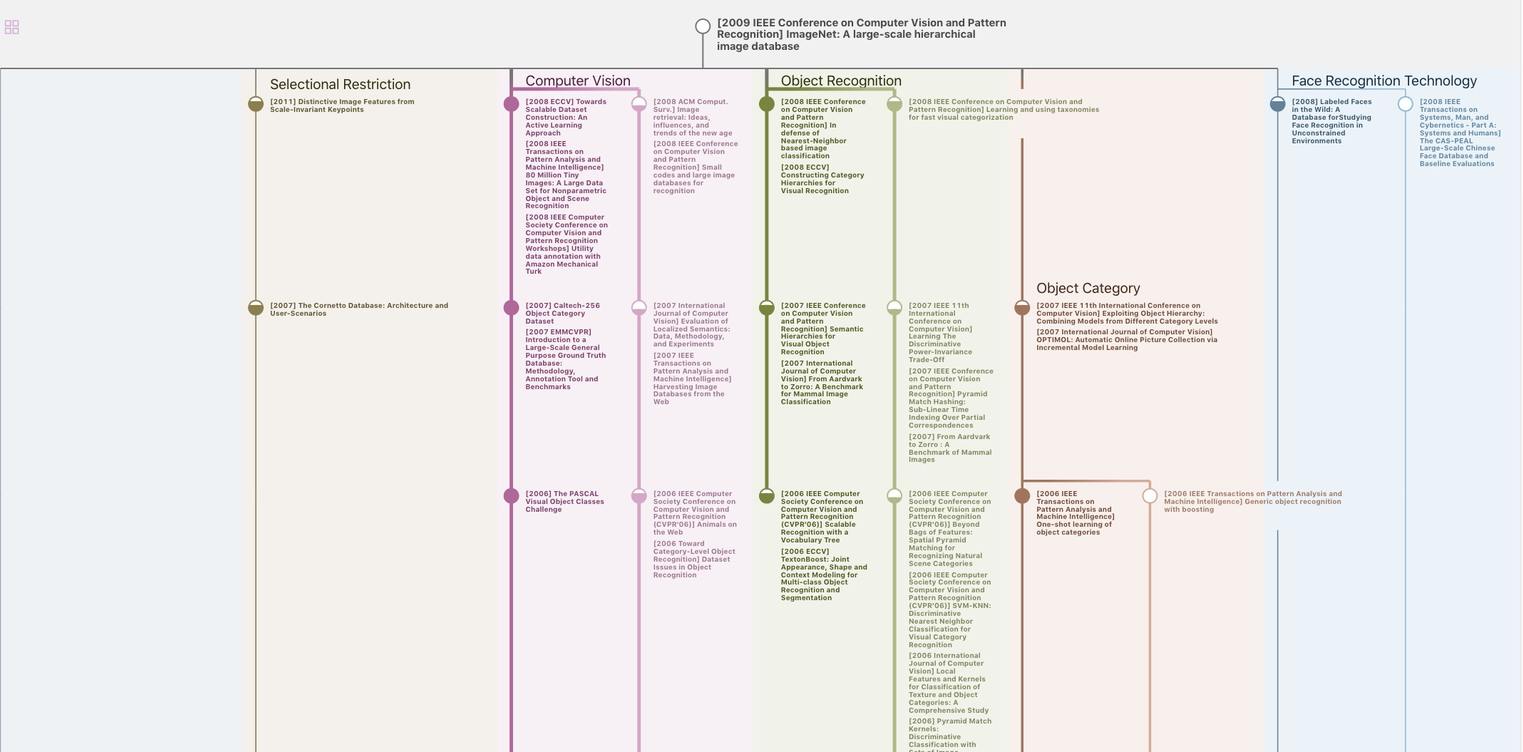
生成溯源树,研究论文发展脉络
Chat Paper
正在生成论文摘要